Multi-Modal Feature Constraint Based Tightly Coupled Monocular Visual-LiDAR Odometry and Mapping
IEEE Transactions on Intelligent Vehicles(2023)
Abstract
In this paper, we present a novel multi-sensor fusion framework for tightly coupled monocular visual-LiDAR odometry and mapping. Compared to previous visual-LiDAR fusion frameworks, our proposed framework leverages more constraints among LiDAR features and visual features and integrates that in a tightly coupled approach. Specifically, the framework starts with a preprocess module which contains LiDAR feature extraction, visual feature extraction and tracking, and visual feature depth recover. Then a frame-to-frame odometry module is established by fusing visual feature tracking and LiDAR feature match between frames, aiming to provide a coarse pose estimation for next module. Finally, to refine the pose and build a multi-modal map, we introduce a multi-modal mapping module that tightly couple multi-modal feature constraints by matching or registering multi-modal features to multi-modal map. In addition, the proposed fusion framework also functions well in sensor-degraded environment (texture-less or structure-less), which increases its robustness. The effectiveness and performance of the proposed fusion framework are demonstrated and evaluated on the public KITTI odometry benchmark, and results show that our proposed fusion framework achieves comparable performance compared with the state-of-the-art visual-LiDAR odometry frameworks.
MoreTranslated text
Key words
mapping,multi-modal,visual-lidar
AI Read Science
Must-Reading Tree
Example
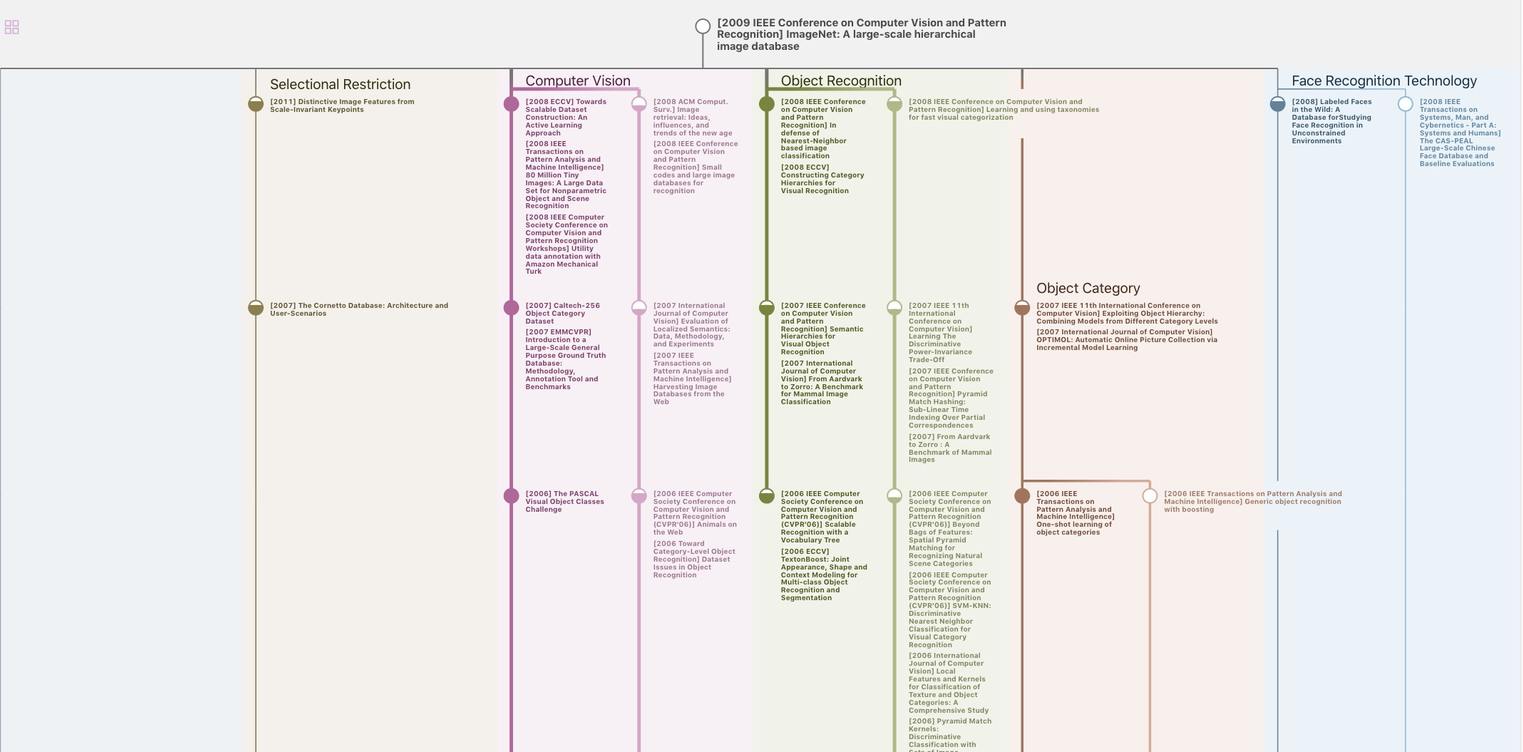
Generate MRT to find the research sequence of this paper
Chat Paper
Summary is being generated by the instructions you defined