Quality Control of Casting Aluminum Parts: A Comparison of Deep Learning Models for Filings Detection
2023 IEEE International Conference on Autonomous Robot Systems and Competitions (ICARSC)(2023)
摘要
Quality control inspection systems are crucial and a key factor in maintaining and ensuring the integrity of any product. The quality inspection task is a repetitive task, when performed by operators only, it can be slow and susceptible to failures due to the lack of attention and fatigue. This work focuses on the inspection of parts made of high-pressure diecast aluminum for components of the automotive industry. In the present case study, last year, 18240 parts needed to be reinspected, requiring approximately 96 hours, a time that could be spent on other tasks. This article performs a comparison of four deep learning models: Faster R-CNN, RetinaNet, YOLOv7, and YOLOv7-tiny, to find out which one is more suited to perform the quality inspection task of detecting metal filings on casting aluminum parts. As for this use-case the prototype must be highly intolerant to False Negatives, that is, the part being defective and passing undetected, Faster R-CNN was considered the bestperforming model based on a Recall value of 96.00%.
更多查看译文
关键词
Quality control,Convolutional Neural Networks,Filings detection,Casting aluminum,Automotive industry
AI 理解论文
溯源树
样例
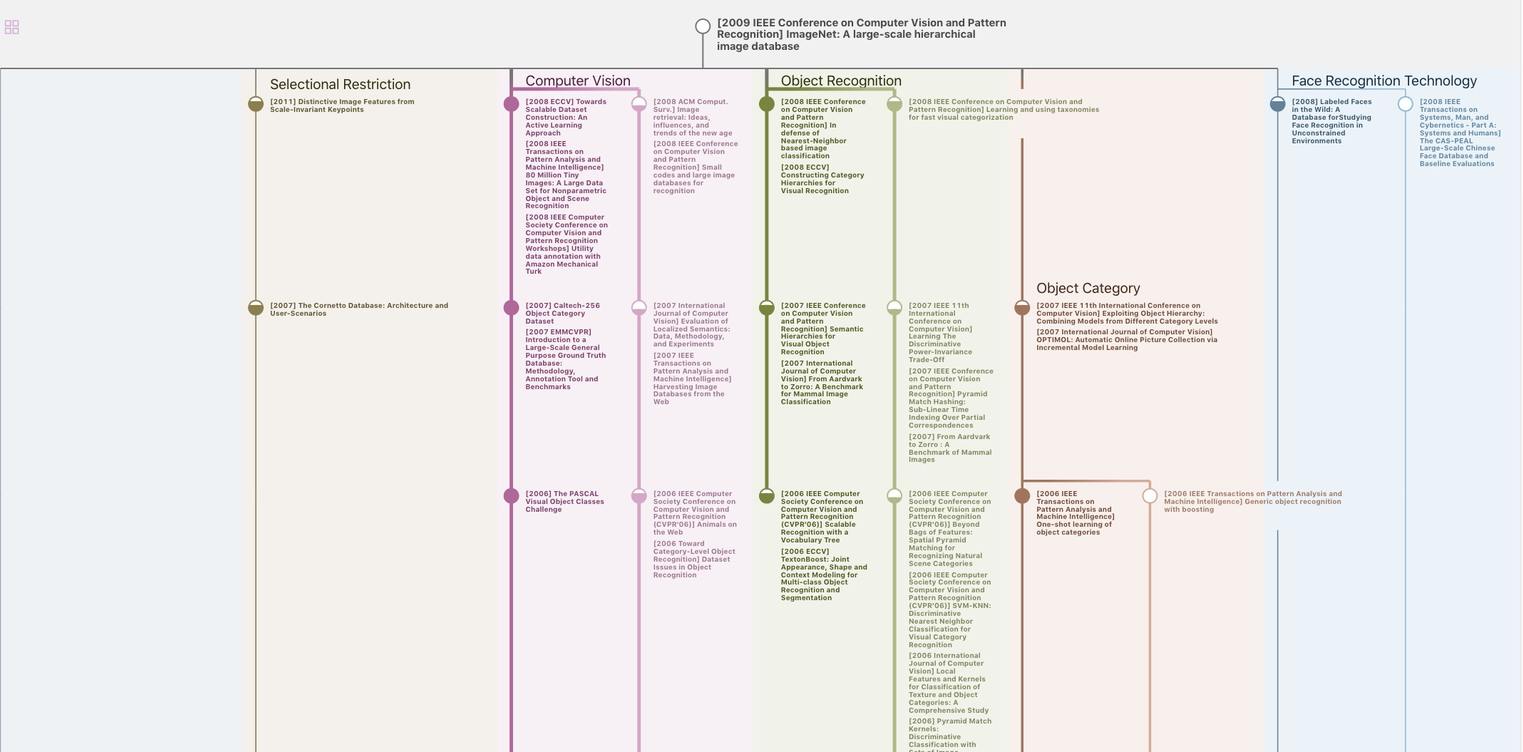
生成溯源树,研究论文发展脉络
Chat Paper
正在生成论文摘要