Diversity-Aware Coherence Loss for Improving Neural Topic Models
61ST CONFERENCE OF THE THE ASSOCIATION FOR COMPUTATIONAL LINGUISTICS, ACL 2023, VOL 2(2023)
摘要
The standard approach for neural topic modeling uses a variational autoencoder (VAE) framework that jointly minimizes the KL divergence between the estimated posterior and prior, in addition to the reconstruction loss. Since neural topic models are trained by recreating individual input documents, they do not explicitly capture the coherence between topic words on the corpus level. In this work, we propose a novel diversity-aware coherence loss that encourages the model to learn corpus-level coherence scores while maintaining a high diversity between topics. Experimental results on multiple datasets show that our method significantly improves the performance of neural topic models without requiring any pretraining or additional parameters.
更多查看译文
关键词
coherence,diversity-aware
AI 理解论文
溯源树
样例
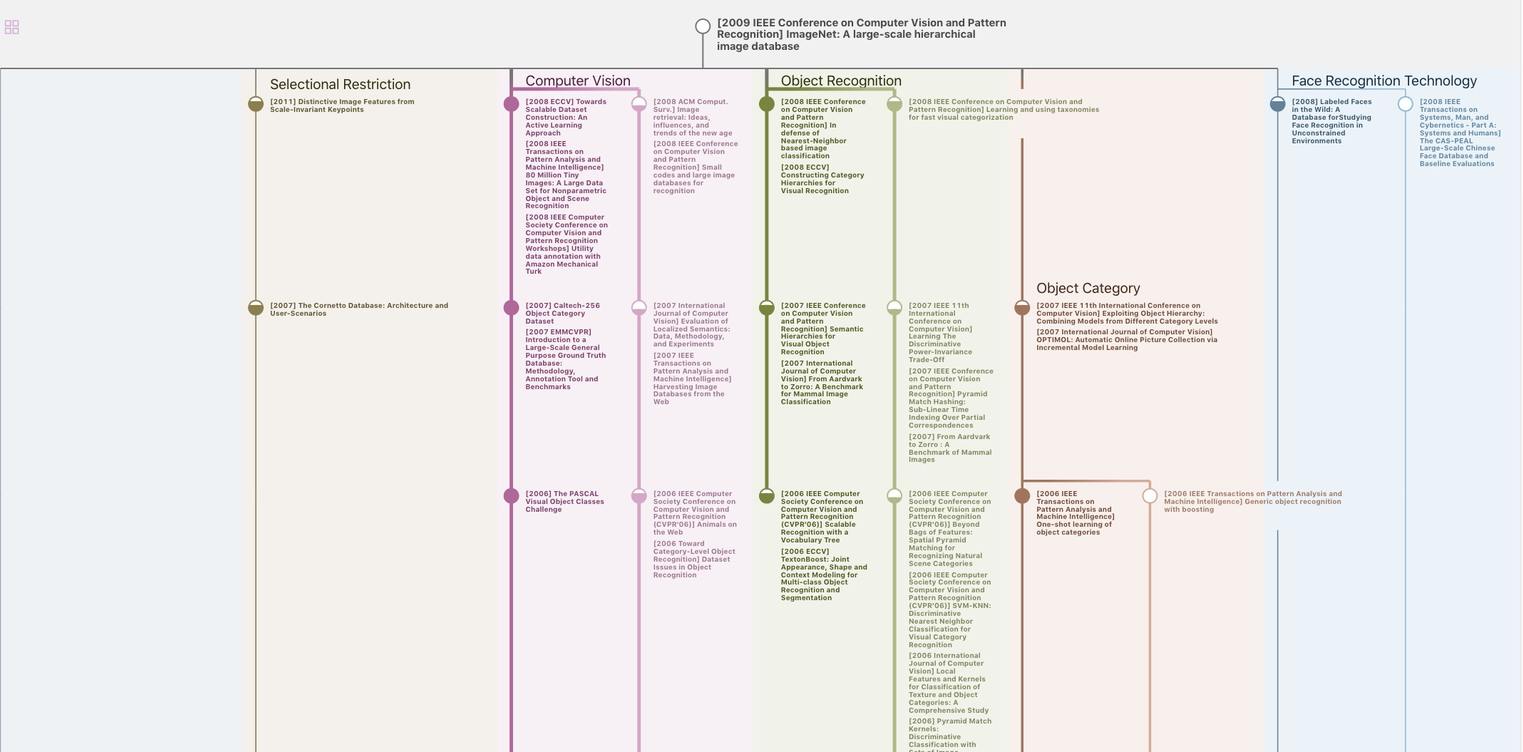
生成溯源树,研究论文发展脉络
Chat Paper
正在生成论文摘要