Learning across Data Owners with Joint Differential Privacy
CoRR(2023)
摘要
In this paper, we study the setting in which data owners train machine learning models collaboratively under a privacy notion called joint differential privacy [Kearns et al., 2018]. In this setting, the model trained for each data owner $j$ uses $j$'s data without privacy consideration and other owners' data with differential privacy guarantees. This setting was initiated in [Jain et al., 2021] with a focus on linear regressions. In this paper, we study this setting for stochastic convex optimization (SCO). We present an algorithm that is a variant of DP-SGD [Song et al., 2013; Abadi et al., 2016] and provides theoretical bounds on its population loss. We compare our algorithm to several baselines and discuss for what parameter setups our algorithm is more preferred. We also empirically study joint differential privacy in the multi-class classification problem over two public datasets. Our empirical findings are well-connected to the insights from our theoretical results.
更多查看译文
关键词
data owners,privacy,learning
AI 理解论文
溯源树
样例
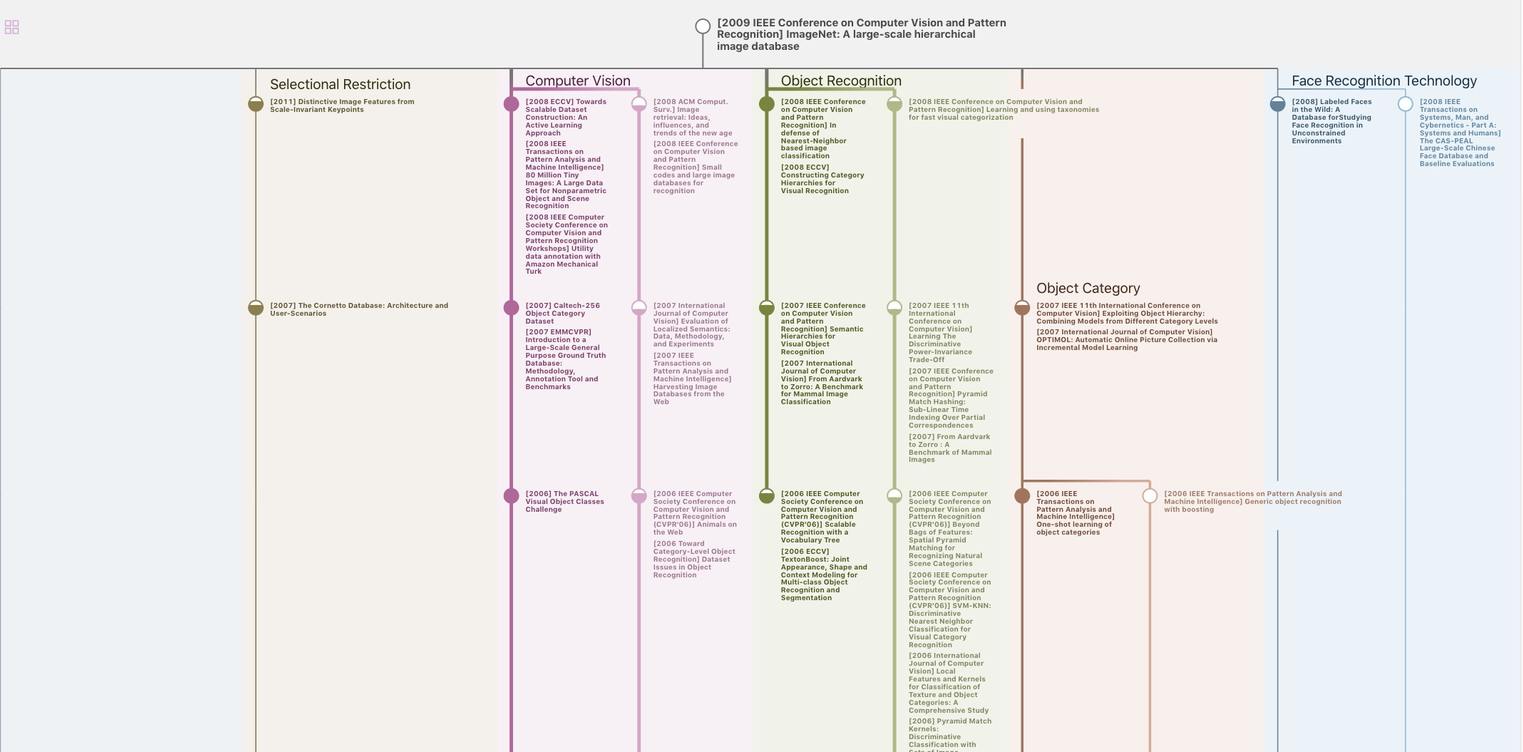
生成溯源树,研究论文发展脉络
Chat Paper
正在生成论文摘要