Regularized Neural Detection for One-Bit Massive MIMO Communication Systems
arXiv (Cornell University)(2023)
摘要
Detection for one-bit massive MIMO systems presents several challenges especially for higher order constellations. Recent advances in both model-based analysis and deep learning frameworks have resulted in several robust one-bit detector designs. Our work builds on the current state-of-the-art gradient descent (GD)-based detector. We introduce two novel contributions in our detector design: (i) We augment each GD iteration with a deep learning-aided regularization step, and (ii) We introduce a novel constellation-based loss function for our regularized DNN detector. This one-bit detection strategy is applied to two different DNN architectures based on algorithm unrolling, namely, a deep unfolded neural network and a deep recurrent neural network. Being trained on multiple randomly sampled channel matrices, these networks are developed as general one-bit detectors. The numerical results show that the combination of the DNN-augmented regularized GD and constellation-based loss function improve the quality of our one-bit detector, especially for higher order M-QAM constellations.
更多查看译文
关键词
massive mimo communication
AI 理解论文
溯源树
样例
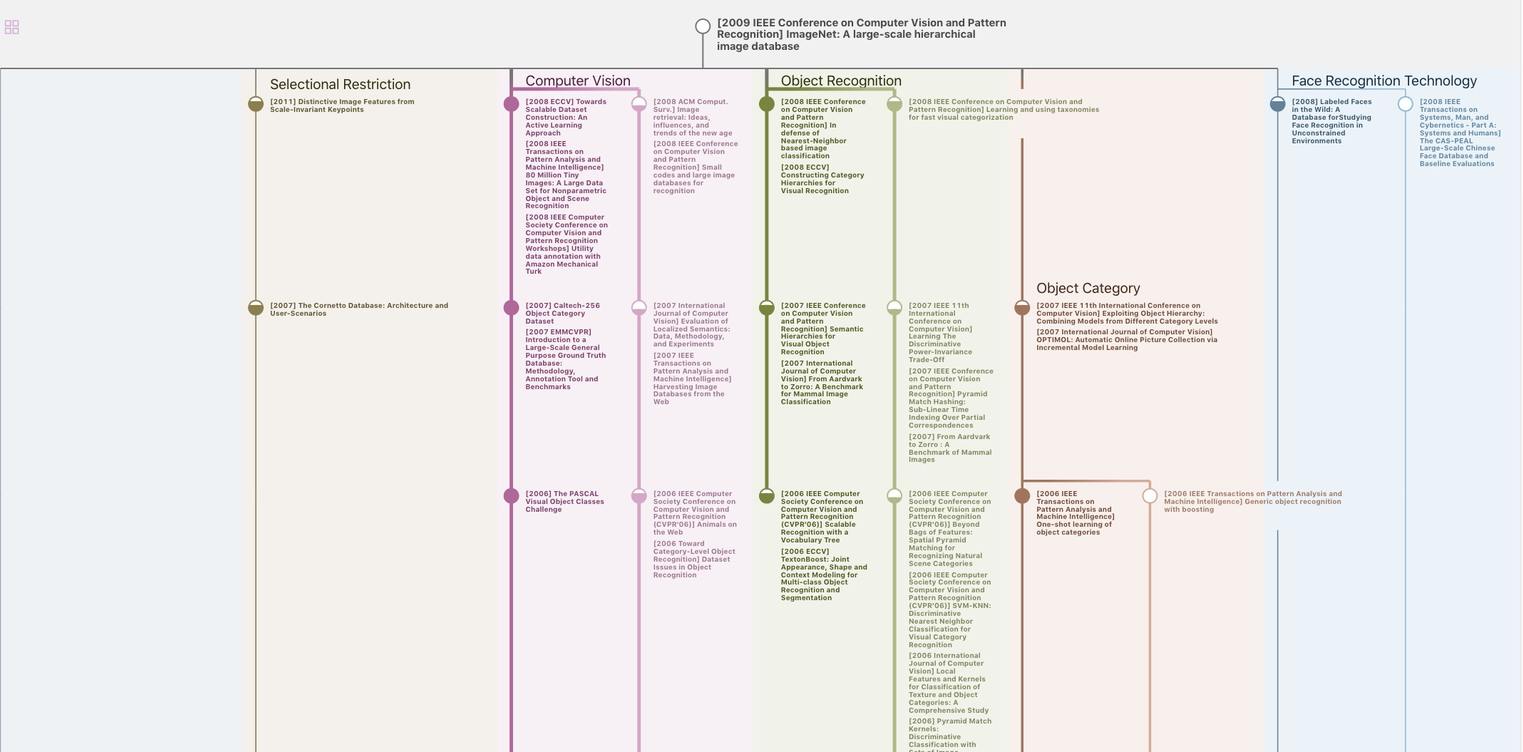
生成溯源树,研究论文发展脉络
Chat Paper
正在生成论文摘要