Quantitative bias analysis for outcome phenotype error correction in comparative effect estimation: an empirical and synthetic evaluation
arXiv (Cornell University)(2023)
摘要
Outcome phenotype measurement error is rarely corrected in comparative effect estimation studies in observational pharmacoepidemiology. Quantitative bias analysis (QBA) is a misclassification correction method that algebraically adjusts person counts in exposure-outcome contingency tables to reflect the magnitude of misclassification. The extent QBA minimizes bias is unclear because few systematic evaluations have been reported. We empirically evaluated QBA impact on odds ratios (OR) in several comparative effect estimation scenarios. We estimated non-differential and differential phenotype errors with internal validation studies using a probabilistic reference. Further, we synthesized an analytic space defined by outcome incidence, uncorrected ORs, and phenotype errors to identify which combinations produce invalid results indicative of input errors. We evaluated impact with relative bias [(OR-ORQBA)]/OR*100%]. Results were considered invalid if any contingency table cell was corrected to a negative number. Empirical bias correction was greatest in lower incidence scenarios where uncorrected ORs were larger. Similarly, synthetic bias correction was greater in lower incidence settings with larger uncorrected estimates. The invalid proportion of synthetic scenarios increased as uncorrected estimates increased. Results were invalid in common, low incidence scenarios indicating problematic inputs. This demonstrates the importance of accurately and precisely estimating phenotype errors before implementing QBA in comparative effect estimation studies.
更多查看译文
关键词
outcome phenotype error correction,comparative effect estimation,quantitative bias analysis,error correction
AI 理解论文
溯源树
样例
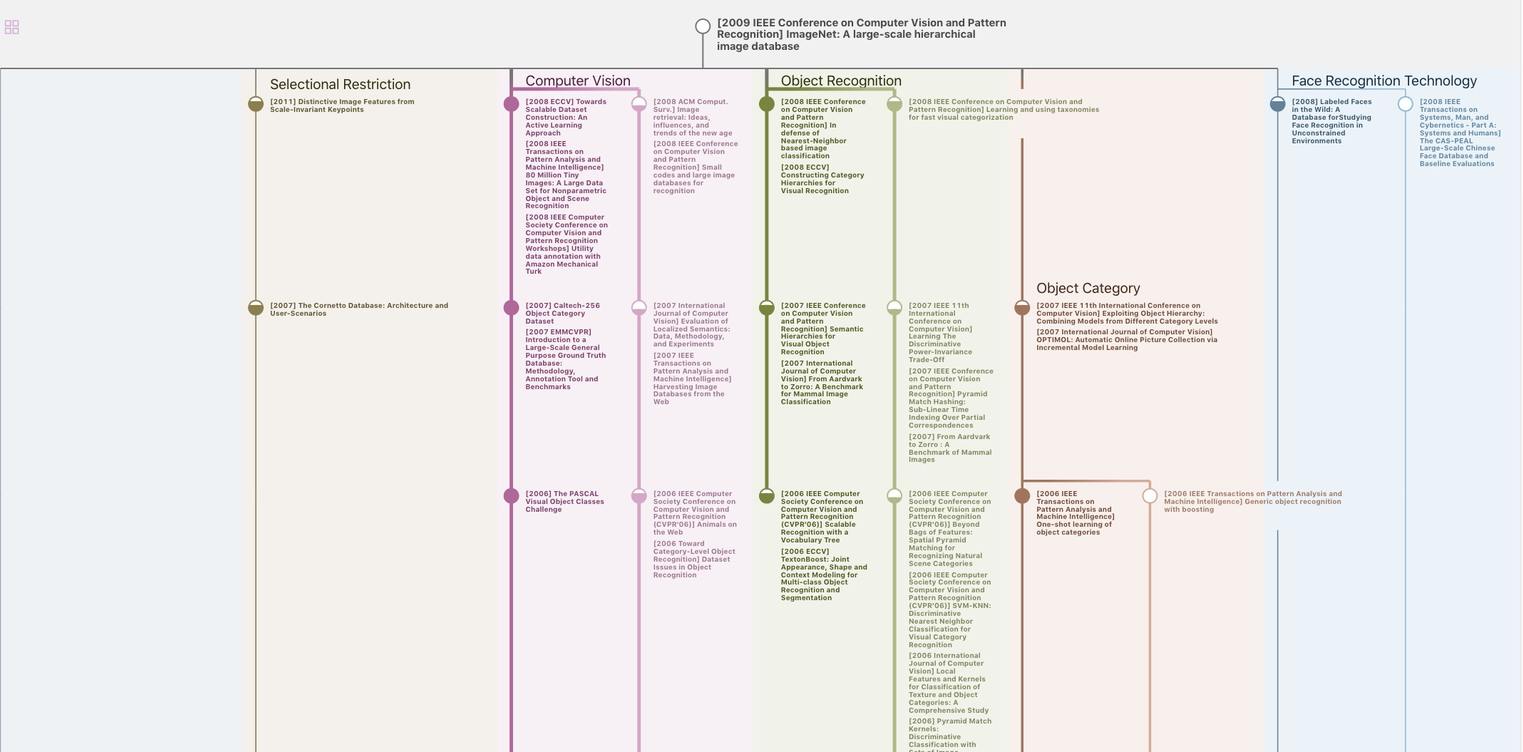
生成溯源树,研究论文发展脉络
Chat Paper
正在生成论文摘要