Intelligent Fault Diagnosis With Noisy Labels via Semisupervised Learning on Industrial Time Series
IEEE Transactions on Industrial Informatics(2023)
摘要
Deep neural networks (DNNs) excel at industrial fault diagnosis. Their performance heavily relies on the quality of human-annotated labels. Due to perception limitations of annotators, industrial time series samples (such as vibration and voltage signals) are frequently mislabeled in several conditions, such as samples with frequency domain feature differences and samples on class borders. Hence, an annotated industrial dataset will inevitably contain noisy labels at a certain level, leading to overfitting and poor generalization of DNNs. In this work, we introduce an industrial noisy label semisupervised learning (INL-SSL) fault diagnosis approach, addressing the problem that a certain number of samples in an industrial dataset are mislabeled. The proposed INL-SSL architecture simultaneously trains two DNNs, which cross-train on each other to filter noisy label errors. In particular, a fitted Gaussian mixture model divides time series samples of each DNN flow into an unlabeled set with samples likely to be noisy and a labeled set with samples likely to be clean. Given the labeled and unlabeled data, we proposed a time series MixMatch semisupervised learning strategy to train the diagnostic model. Ablation study verifies the benefit of the proposed time series augmentation techniques for semisupervised training. Extensive experiments on a benchmark industrial dataset of rolling element bearings (REB) reveal that the INL-SSL outperforms state-of-the-art approaches. On another self-collected REB dataset, the proposed approach also exceeds other comparison methods under noise ratios from 20% to 90%, validating the model's generalizability.
更多查看译文
关键词
intelligent fault diagnosis,fault diagnosis,semisupervised learning,industrial time series,noisy labels
AI 理解论文
溯源树
样例
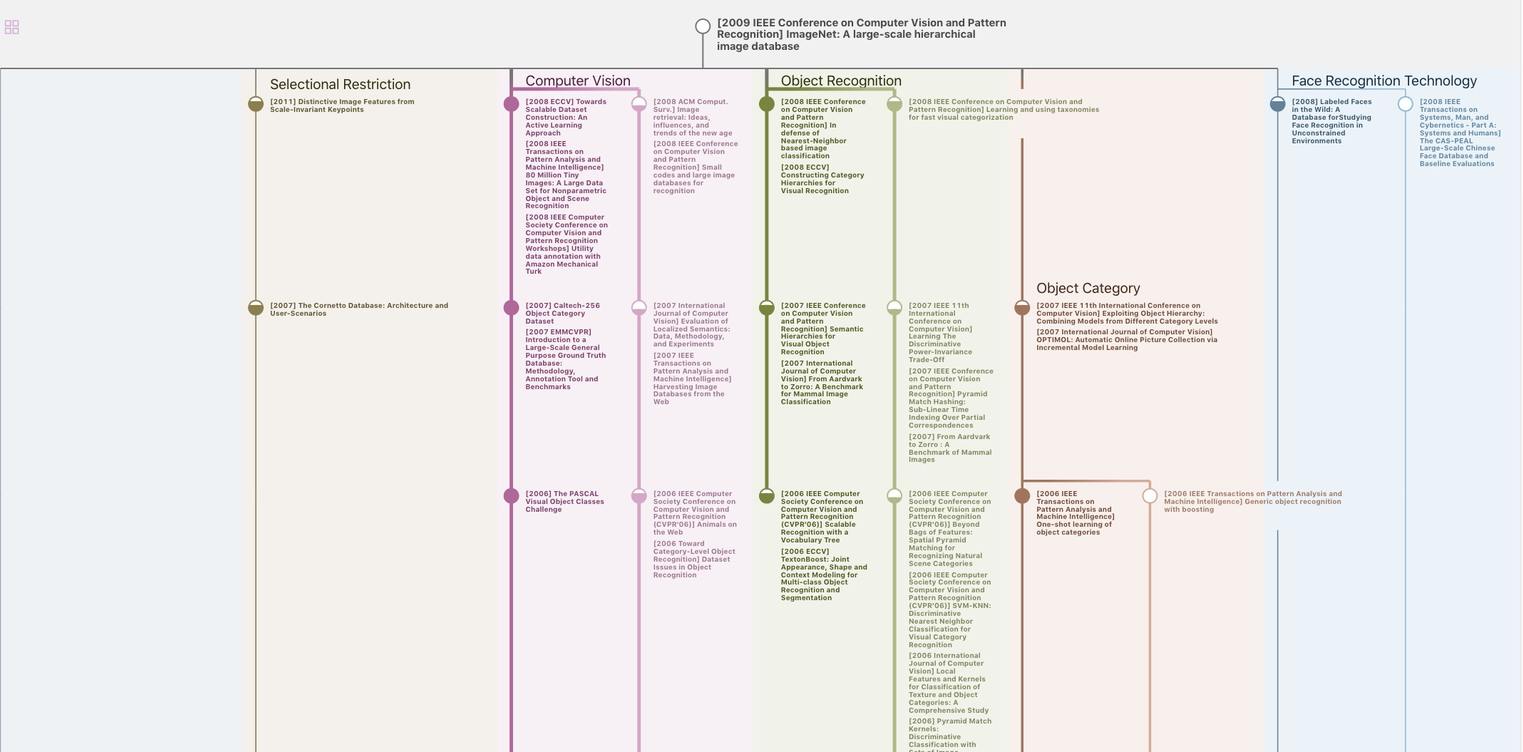
生成溯源树,研究论文发展脉络
Chat Paper
正在生成论文摘要