A Deep Learning Architecture for Egocentric Time-to-Saccade Prediction usingWeibull Mixture-Models and Historic Priors
2023 Symposium on Eye Tracking Research and Applications(2023)
摘要
Real-time detection of saccades is of major interest for many applications in human-computer interaction and mixed reality. However, due to relatively low update rates and high latencies of current commercially available eye trackers, gaze events are typically detected after they occur with some delay. This limits interaction scenarios such as intent-based gaze interaction, redirected walking, or gaze forecasting. In this paper, we propose a deep learning framework for time-to-event prediction of saccades. In contrast to previous approaches, we utilize past multimodal data captured from head-mounted displays. We combine the well-established transformer architecture with a Weibull Mixture Model. This also allows estimating the uncertainty of the prediction. Additionally, we propose a sampling strategy that differs from conventional approaches to better account for the temporal properties of gaze sequences. We demonstrate that our model achieves state-of-the-art performance by evaluating it on three datasets and performing multiple ablation studies.
更多查看译文
关键词
time-to-saccade,time-to-event prediction,gaze event classification,virtual reality,deep learning
AI 理解论文
溯源树
样例
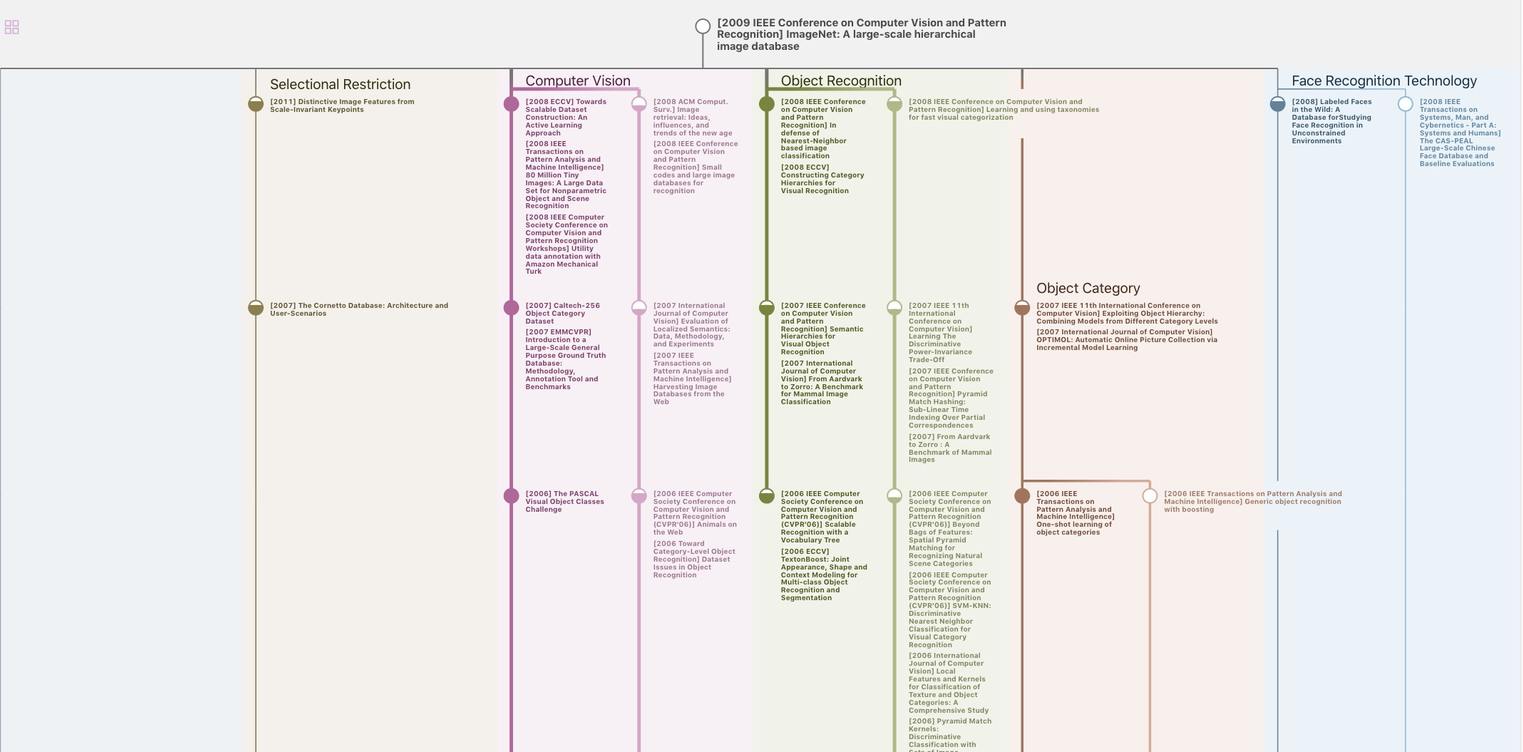
生成溯源树,研究论文发展脉络
Chat Paper
正在生成论文摘要