Scene-insensitive Driving Style Recognition using CAN Signals based on Factor Analysis
2023 IEEE 6th International Conference on Industrial Cyber-Physical Systems (ICPS)(2023)
Abstract
Driving style recognition plays a vital role in developing human-centered intelligent vehicles that consider drivers' preferences. However, the feature selection of driving style recognition is diverse and inconsistent, which varies with driving scenarios. Therefore, the application of driving style is limited by the accuracy and the rapidity of the driving scene recognition algorithm, which is difficult for low-cost onboard chips. To solve the problem, this paper proposes a scene-insensitive method for driving style recognition. Factor analysis is employed to extract common factors in diverse driving scenes from high-dimensional driving data segmentation. The unified common factors reflect the differences in drivers' driving behaviors with different styles, verified in the publicly available dataset and 100-driver experimental data. Then, an efficient driving style recognition algorithm is developed based on K-means Clustering. Finally, natural driving data from 100 drivers in Changchun, China, is collected to evaluate the proposed method with the driving style questionnaire. Compared with six supervised learning methods, experimental results demonstrate that the proposed method provides an efficient and scene-insensitive way to recognize the driving style.
MoreTranslated text
Key words
driving style, human-centered, factor analysis, scene-insensitive
AI Read Science
Must-Reading Tree
Example
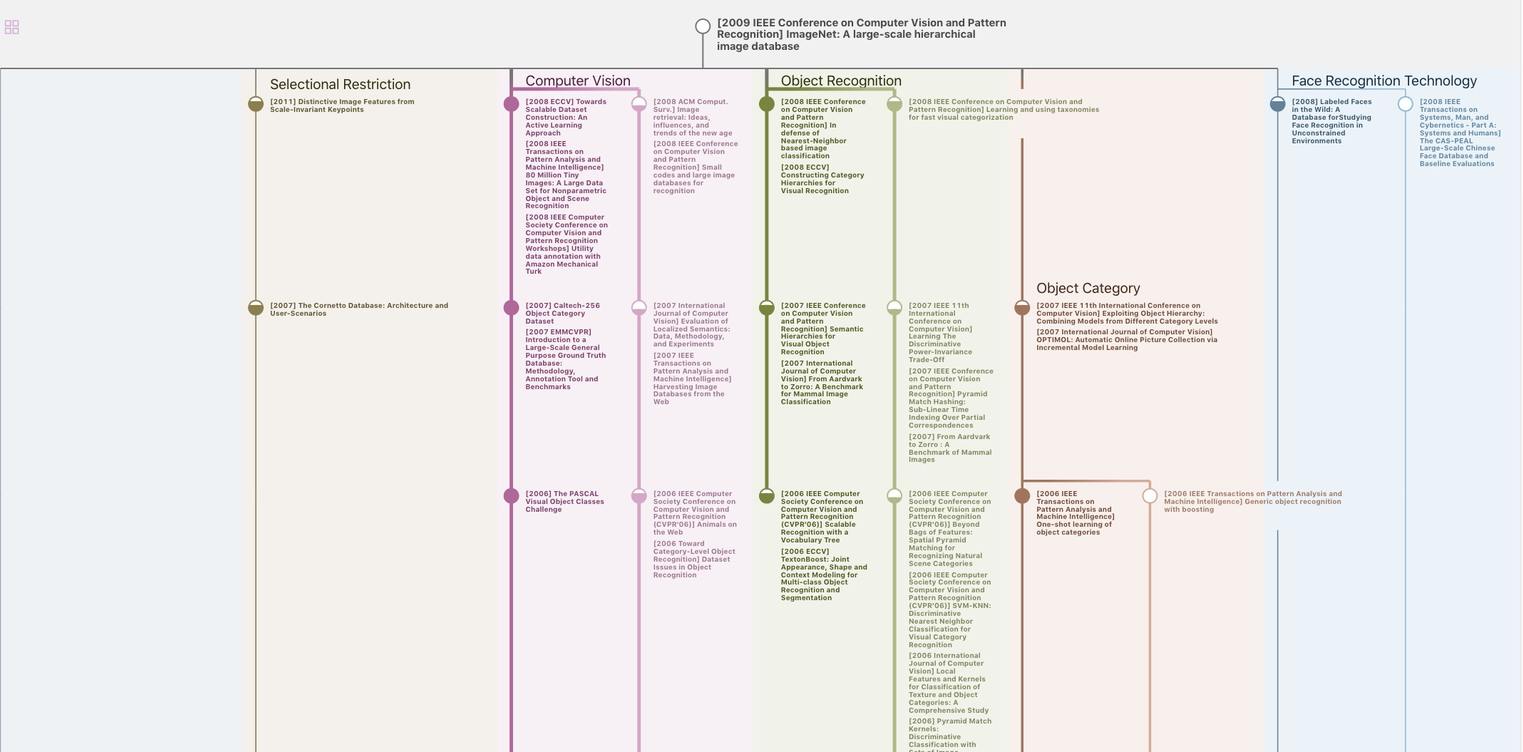
Generate MRT to find the research sequence of this paper
Chat Paper
Summary is being generated by the instructions you defined