Adaptive Data Augmentation for Supervised Learning over Missing Data
Proc VLDB Endow(2021)
摘要
Real-world data is dirty, which causes serious problems in (supervised) machine learning (ML). The widely used practice in such scenario is to first repair the labeled source (a.k.a. train) data using rule-, statistical- or ML-based methods and then use the "repaired" source to train an ML model. During production, unlabeled target (a.k.a. test) data will also be repaired, and is then fed in the trained ML model for prediction. However, this process often causes a performance degradation when the source and target datasets are dirty with different noise patterns, which is common in practice. In this paper, we propose an adaptive data augmentation approach, for handling missing data in supervised ML. The approach extracts noise patterns from target data, and adapts the source data with the extracted target noise patterns while still preserving supervision signals in the source. Then, it patches the ML model by retraining it on the adapted data, in order to better serve the target. To effectively support adaptive data augmentation, we propose a novel generative adversarial network (GAN) based framework, called DAGAN, which works in an unsupervised fashion. DAGAN consists of two connected GAN networks. The first GAN learns the noise pattern from the target, for target mask generation. The second GAN uses the learned target mask to augment the source data, for source data adaptation. The augmented source data is used to retrain the ML model. Extensive experiments show that our method significantly improves the ML model performance and is more robust than the state-of-the-art missing data imputation solutions for handling datasets with different missing value patterns.
更多查看译文
关键词
adaptive data augmentation,supervised learning
AI 理解论文
溯源树
样例
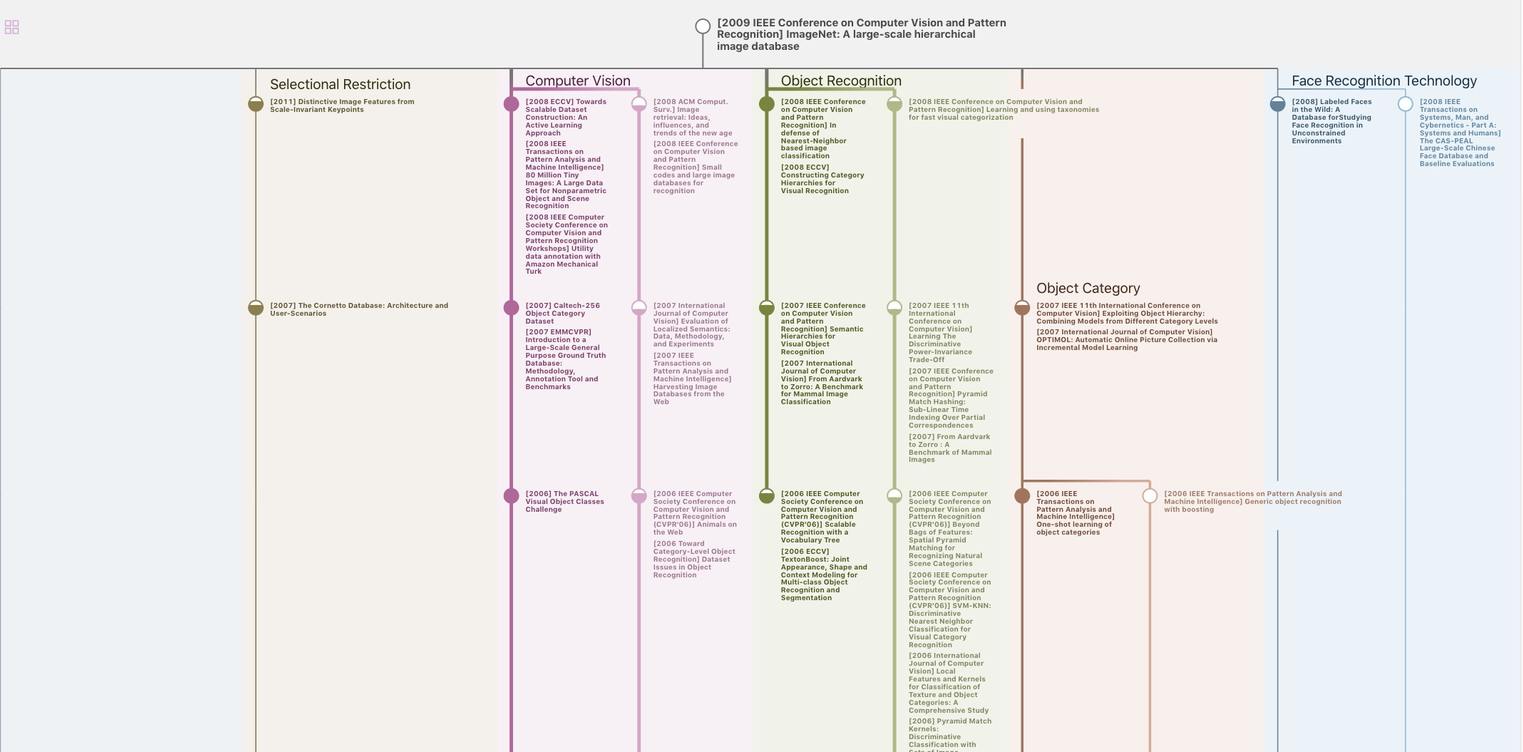
生成溯源树,研究论文发展脉络
Chat Paper
正在生成论文摘要