Machine learning forecasting of extreme fluctuations in a human balancing task
Knowledge-Based Systems(2023)
Abstract
Here we consider the problem of predicting falls using real data from human stick balancing experiments implementing k-nearest neighbors, random forest, support vector machine, and a neural network. Carrying out two different approaches to evaluate the temporal series, it is shown that, in one case, prefall segments can be classified with an F1 score larger than 0.97, using the neural network and the random forest; being able to detect changes in the pattern preceding a fall around 0.83 s before. An additional approach can identify the temporal position of fall precursors in a best-case scenario with an F1 score >0.9, using the neural network, at times around 0.83 s before. In general, we found that the classifiers behave well when dealing with this complex classification task. Best performing methods in both approaches coincide in determining a falling precursor at 0.83 s before the escape — coinciding with previous results. These results may help establishing a reference for future works using other algorithms best suited to predict complex temporal series.
MoreTranslated text
Key words
68T10,37M10,70L05,37N35,Human stick balancing,Forecasting,Machine learning,Complex temporal series
AI Read Science
Must-Reading Tree
Example
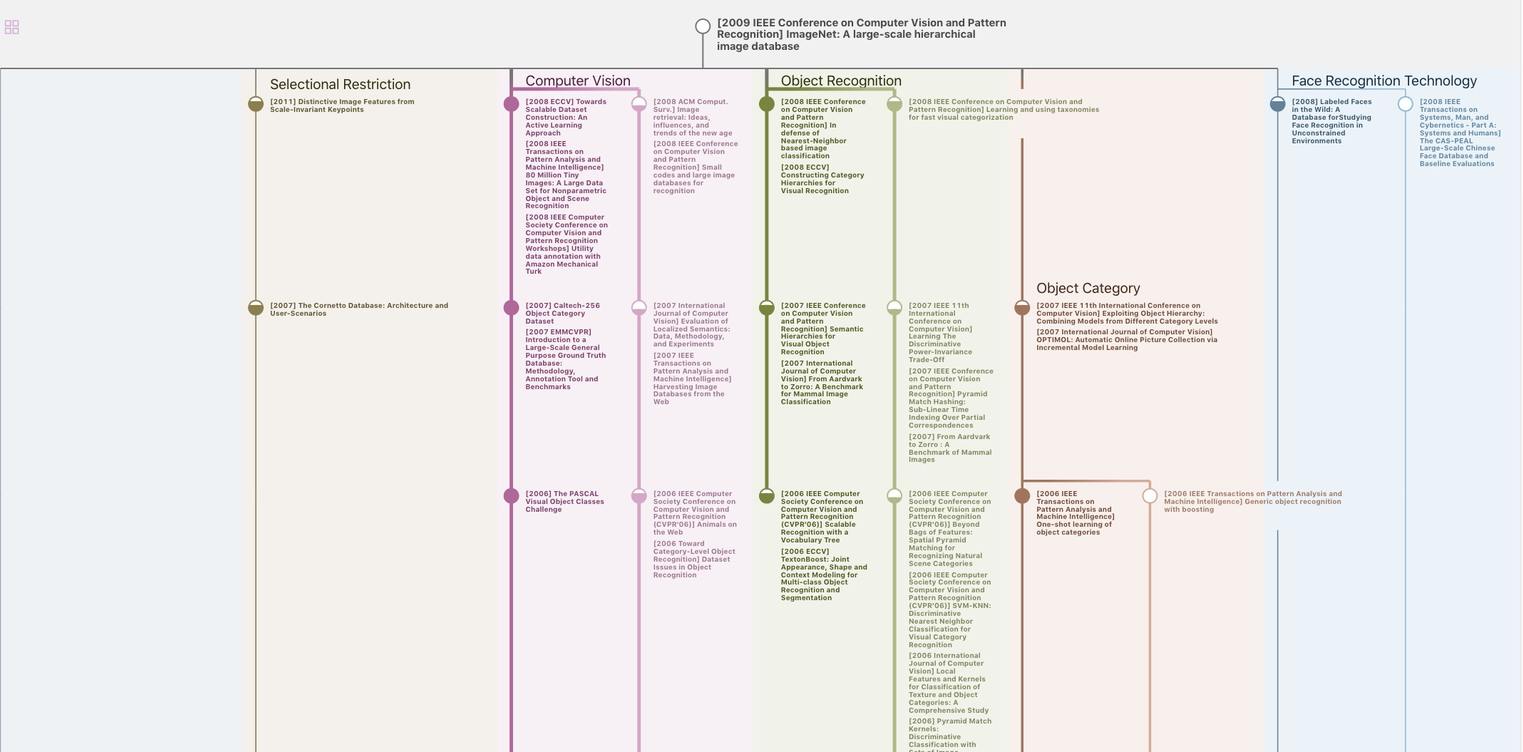
Generate MRT to find the research sequence of this paper
Chat Paper
Summary is being generated by the instructions you defined