Active Learning for Natural Language Generation.
CoRR(2023)
摘要
The field of text generation suffers from a severe shortage of labeled data due to the extremely expensive and time consuming process involved in manual annotation. A natural approach for coping with this problem is active learning (AL), a well-known machine learning technique for improving annotation efficiency by selectively choosing the most informative examples to label. However, while AL has been well-researched in the context of text classification, its application to text generation remained largely unexplored. In this paper, we present a first systematic study of active learning for text generation, considering a diverse set of tasks and multiple leading AL strategies. Our results indicate that existing AL strategies, despite their success in classification, are largely ineffective for the text generation scenario, and fail to consistently surpass the baseline of random example selection. We highlight some notable differences between the classification and generation scenarios, and analyze the selection behaviors of existing AL strategies. Our findings motivate exploring novel approaches for applying AL to NLG tasks.
更多查看译文
关键词
active learning,generation,language
AI 理解论文
溯源树
样例
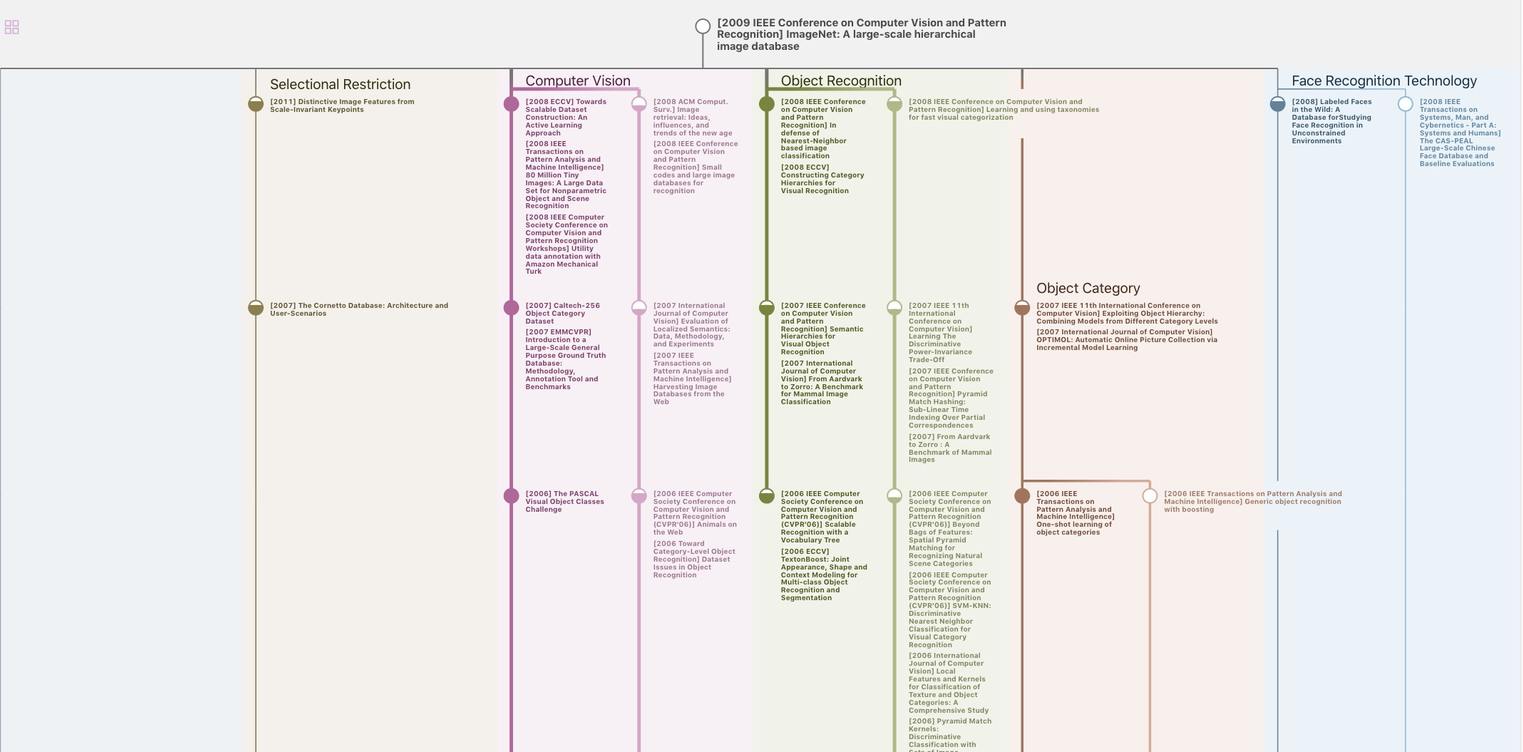
生成溯源树,研究论文发展脉络
Chat Paper
正在生成论文摘要