Sampling-based Uncertainty Estimation for an Instance Segmentation Network
CoRR(2023)
Abstract
The examination of uncertainty in the predictions of machine learning (ML) models is receiving increasing attention. One uncertainty modeling technique used for this purpose is Monte-Carlo (MC)-Dropout, where repeated predictions are generated for a single input. Therefore, clustering is required to describe the resulting uncertainty, but only through efficient clustering is it possible to describe the uncertainty from the model attached to each object. This article uses Bayesian Gaussian Mixture (BGM) to solve this problem. In addition, we investigate different values for the dropout rate and other techniques, such as focal loss and calibration, which we integrate into the Mask-RCNN model to obtain the most accurate uncertainty approximation of each instance and showcase it graphically.
MoreTranslated text
Key words
instance segmentation
AI Read Science
Must-Reading Tree
Example
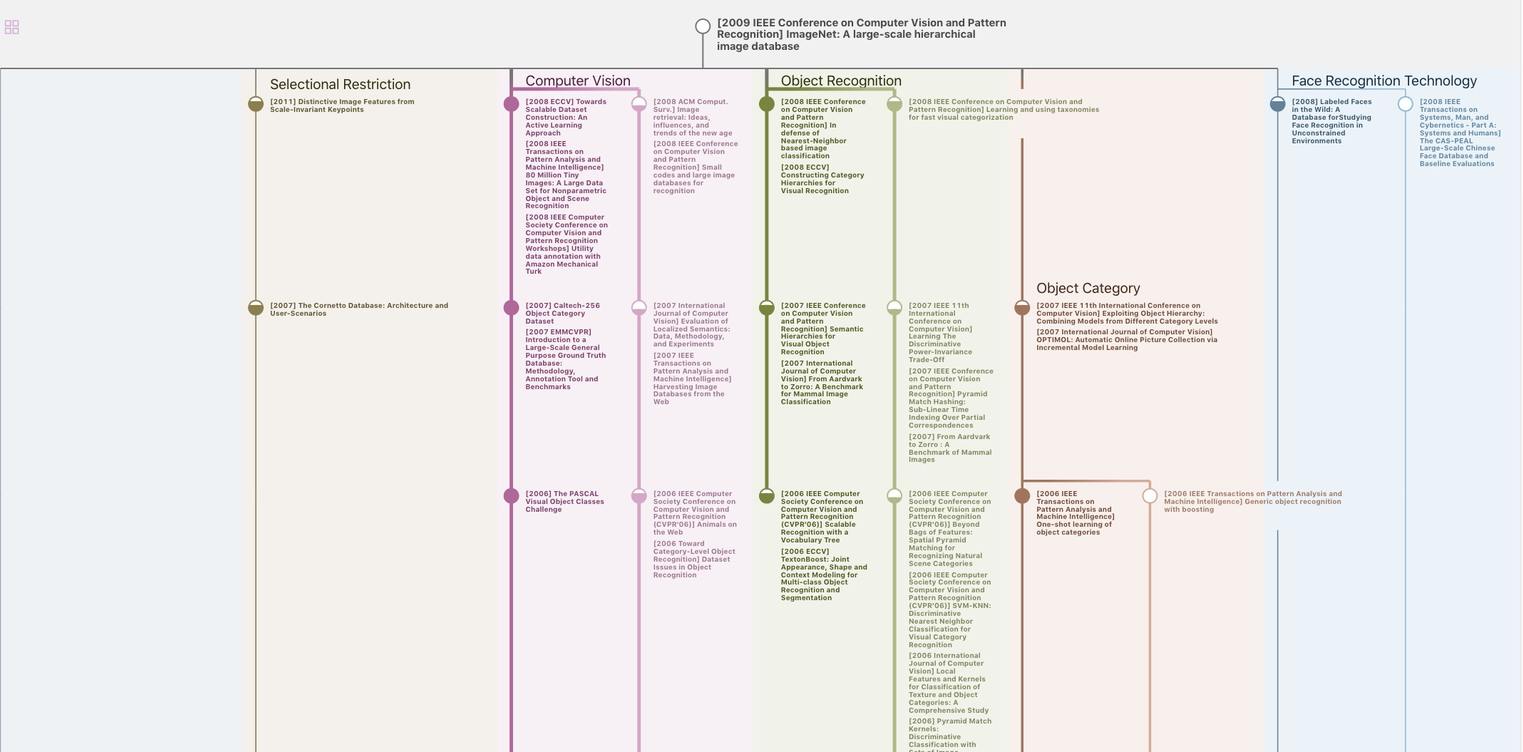
Generate MRT to find the research sequence of this paper
Chat Paper
Summary is being generated by the instructions you defined