Mitigating Temporal Misalignment by Discarding Outdated Facts.
CoRR(2023)
摘要
While large language models are able to retain vast amounts of world knowledge seen during pretraining, such knowledge is prone to going out of date and is nontrivial to update. Furthermore, these models are often used under temporal misalignment, tasked with answering questions about the present, despite having only been trained on data collected in the past. To mitigate the effects of temporal misalignment, we propose fact duration prediction: the task of predicting how long a given fact will remain true. In our experiments, we demonstrate how identifying facts that are prone to rapid change can help models avoid from reciting outdated information and identify which predictions require seeking out up-to-date knowledge sources. We also show how modeling fact duration improves calibration for knowledge-intensive tasks, such as open-retrieval question answering, under temporal misalignment by discarding volatile facts. Our data and code will be released publicly at https://github.com/mikejqzhang/mitigating_misalignment.
更多查看译文
AI 理解论文
溯源树
样例
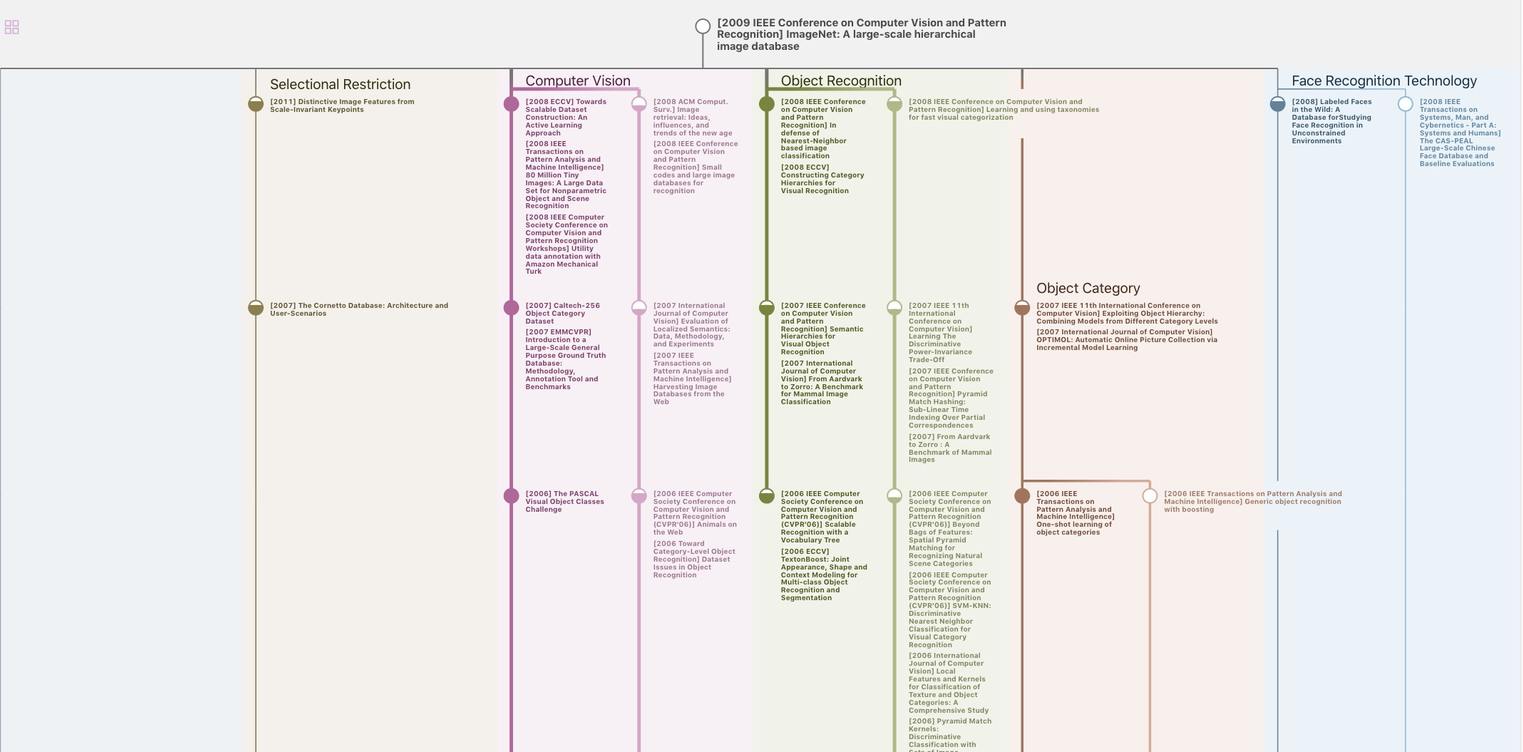
生成溯源树,研究论文发展脉络
Chat Paper
正在生成论文摘要