Can Copyright be Reduced to Privacy?
CoRR(2023)
摘要
There is an increasing concern that generative AI models may produce outputs that are remarkably similar to the copyrighted input content on which they are trained. This worry has escalated as the quality and complexity of generative models have immensely improved, and the availability of large datasets containing copyrighted material has increased. Researchers are actively exploring strategies to mitigate the risk of producing infringing samples, and a recent line of work suggests to employ techniques such as differential privacy and other forms of algorithmic stability to safeguard copyrighted content. In this work, we examine the question whether algorithmic stability techniques such as differential privacy are suitable to ensure the responsible use of generative models without inadvertently violating copyright laws. We argue that there are fundamental differences between privacy and copyright that should not be overlooked. In particular we highlight that although algorithmic stability may be perceived as a practical tool to detect copying, it does not necessarily equate to copyright protection. Therefore, if it is adopted as standard for copyright infringement, it may undermine copyright law intended purposes.
更多查看译文
关键词
privacy,copyright
AI 理解论文
溯源树
样例
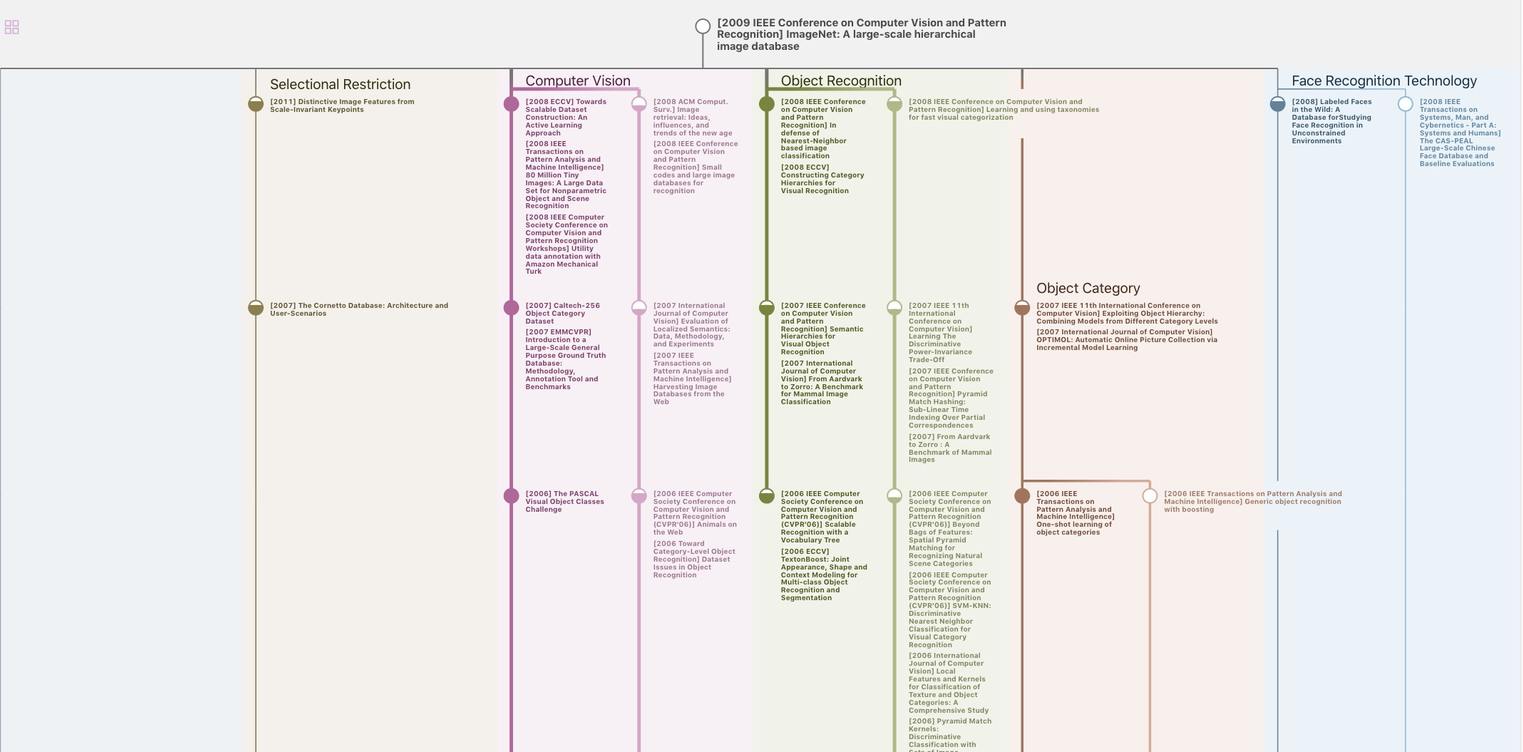
生成溯源树,研究论文发展脉络
Chat Paper
正在生成论文摘要