On progressive sharpening, flat minima and generalisation
CoRR(2023)
摘要
We present a new approach to understanding the relationship between loss curvature and generalisation in deep learning. Specifically, we use existing empirical analyses of the spectrum of deep network loss Hessians to ground an ansatz tying together the loss Hessian and the input-output Jacobian of a deep neural network. We then prove a series of theoretical results which quantify the degree to which the input-output Jacobian of a model approximates its Lipschitz norm over a data distribution, and deduce a novel generalisation bound in terms of the empirical Jacobian. We use our ansatz, together with our theoretical results, to give a new account of the recently observed progressive sharpening phenomenon, as well as the generalisation properties of flat minima. Experimental evidence is provided to validate our claims.
更多查看译文
关键词
flat minima
AI 理解论文
溯源树
样例
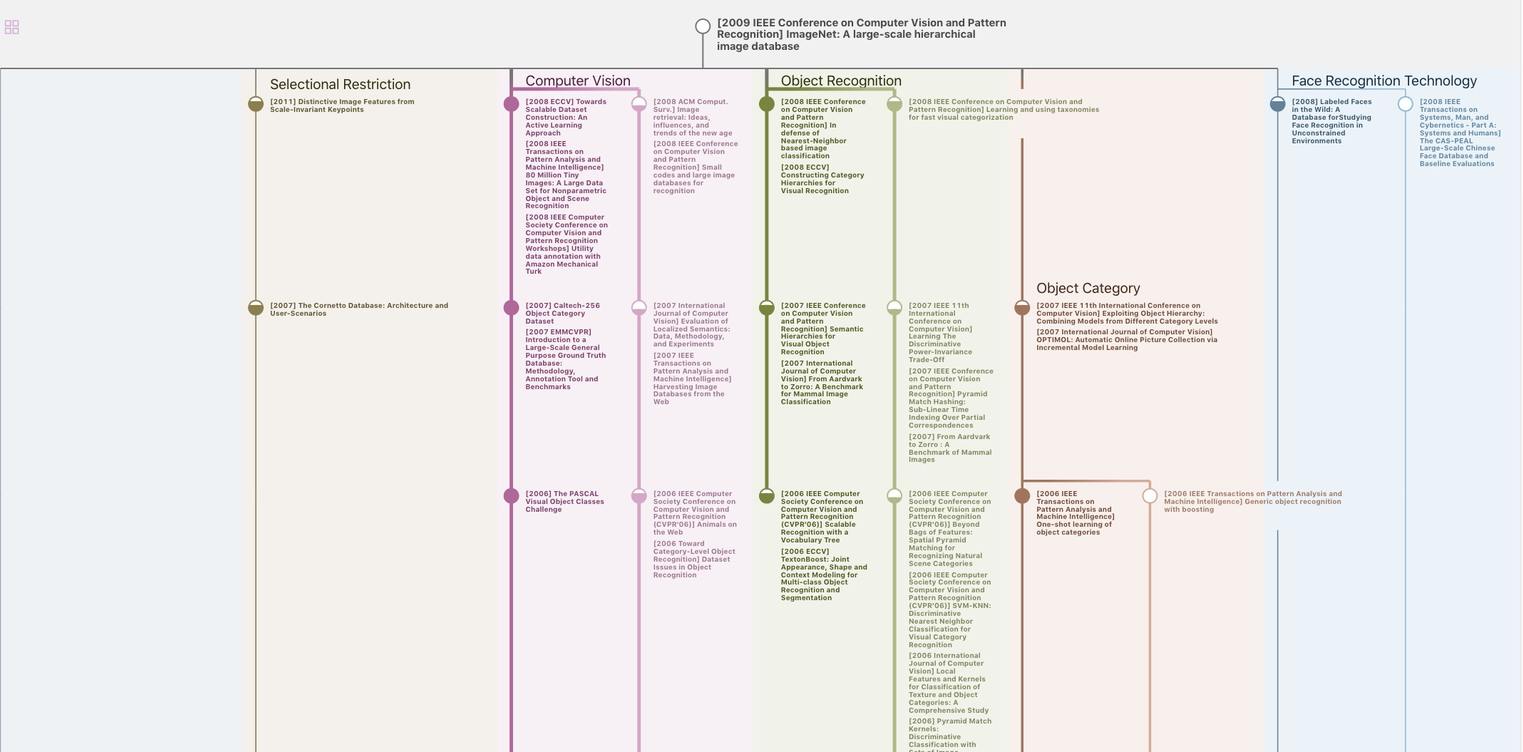
生成溯源树,研究论文发展脉络
Chat Paper
正在生成论文摘要