T1: Scaling Diffusion Probabilistic Fields to High-Resolution on Unified Visual Modalities
CoRR(2023)
摘要
Diffusion Probabilistic Field (DPF) models the distribution of continuous functions defined over metric spaces. While DPF shows great potential for unifying data generation of various modalities including images, videos, and 3D geometry, it does not scale to a higher data resolution. This can be attributed to the ``scaling property'', where it is difficult for the model to capture local structures through uniform sampling. To this end, we propose a new model comprising of a view-wise sampling algorithm to focus on local structure learning, and incorporating additional guidance, e.g., text description, to complement the global geometry. The model can be scaled to generate high-resolution data while unifying multiple modalities. Experimental results on data generation in various modalities demonstrate the effectiveness of our model, as well as its potential as a foundation framework for scalable modality-unified visual content generation.
更多查看译文
关键词
visual modalities,diffusion,high-resolution high-resolution,scaling,probabilistic fields
AI 理解论文
溯源树
样例
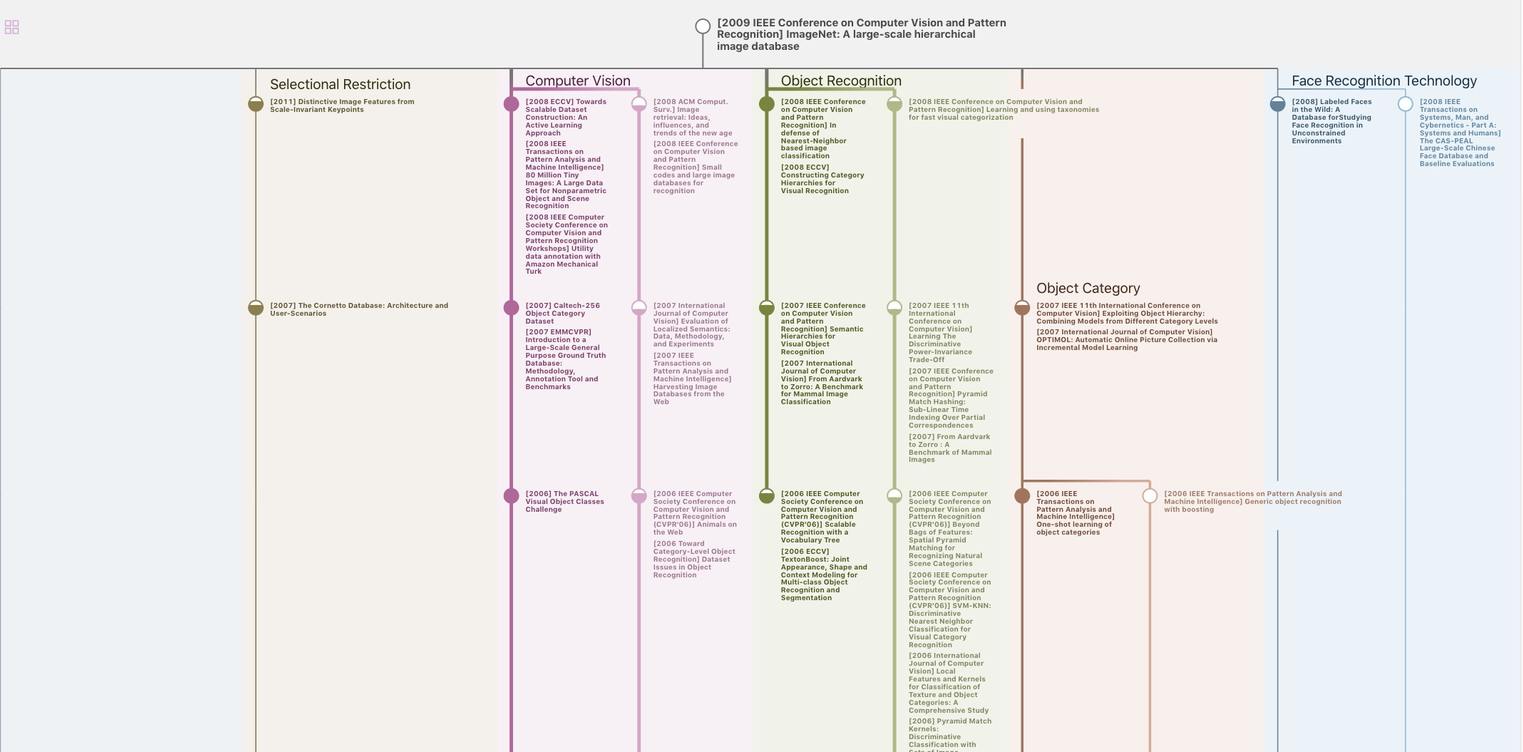
生成溯源树,研究论文发展脉络
Chat Paper
正在生成论文摘要