Shiitake Mushroom Semantic Segmentation Method Based on Search Focus Network
2023 9th International Conference on Automation, Robotics and Applications (ICARA)(2023)
Abstract
The substantially similar texture features of sticks and shiitake mushrooms in the mushroom-growing environment make precisely labeled samples more expensive and semantic segmentation of shiitake mushrooms more challenging. In this paper, a search focus network(SFNet) for semantic segmentation of shiitake mushrooms was proposed, which utilized the group-reversal attention module(GRAM) to strengthen semantic information understanding and trained via transfer learning and data augmentation strategies. The experimental results on the self-built shiitake mushroom sticks dataset revealed that structural measure
$S_{\alpha}$
, weighted F-measure
$F_{\beta}^{\omega}$
, adaptive E-measure
$E_{\phi}^{ad}$
, and absolute mean error
$M$
of SFNet were 0.9161, 0.9113, 0.9808, and 0.0049, respectively, with practical and steady performance. With only a few training samples, the proposed approach can accomplish the semantic segmentation task of shiitake mushrooms.
MoreTranslated text
Key words
Semantic segmentation,Transfer learning,Data augmentation,Attention mechanism
AI Read Science
Must-Reading Tree
Example
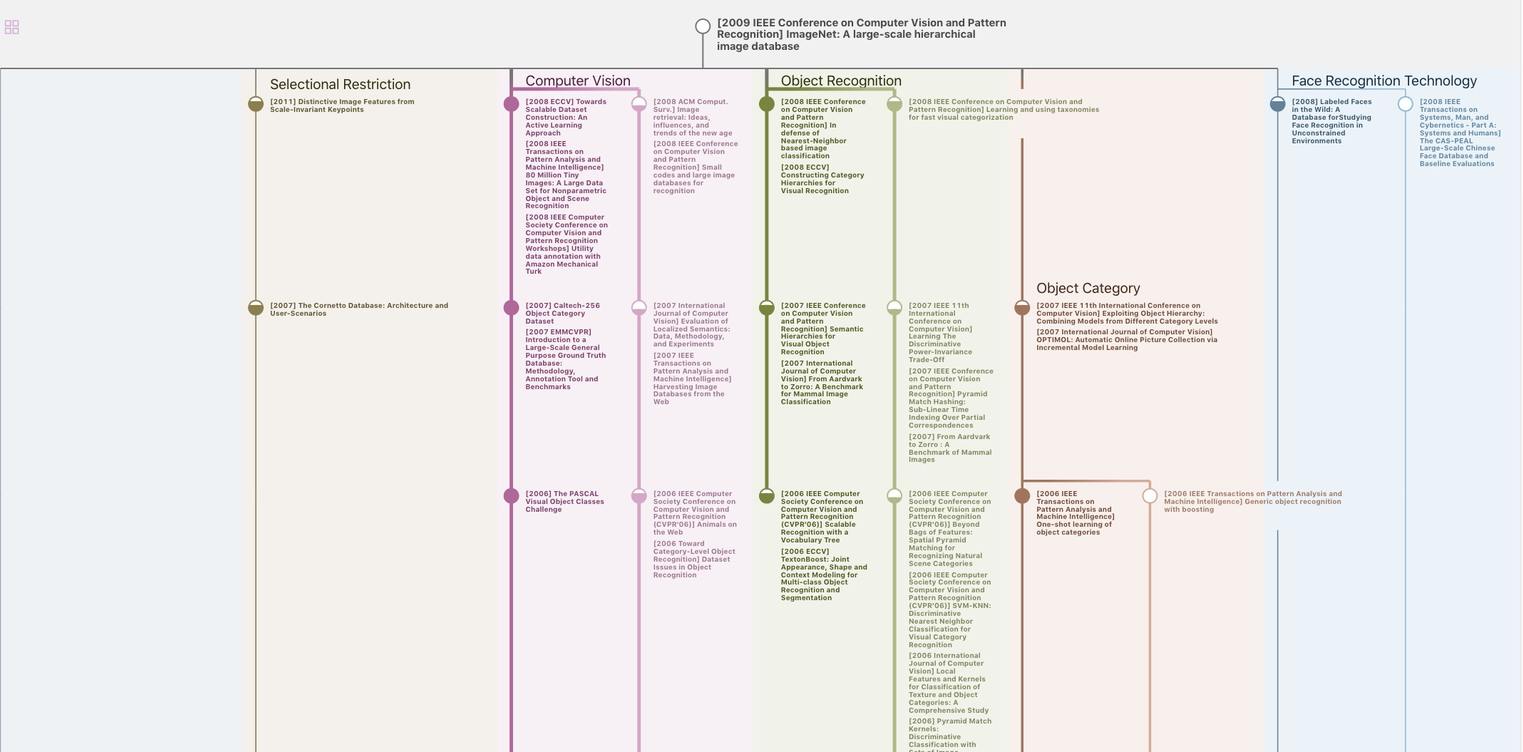
Generate MRT to find the research sequence of this paper
Chat Paper
Summary is being generated by the instructions you defined