Thyroid Disease Prediction Model on Boosting-based Stacking Ensemble Approach
2023 IEEE 8th International Conference for Convergence in Technology (I2CT)(2023)
摘要
The thyroid gland plays a significant role in the human body's metabolism, growth, and development. Though it is not a life-threatening disease, a person suffering from thyroid faces many complications in their daily life. Recent trends have shown that women suffer more from thyroid-related diseases than men. The many contributing factors that lead to thyroid disease may be controlled upon early diagnosis stages. Machine learning prediction models help healthcare professionals diagnose thyroid diseases at an initial stage and take measures accordingly. This study deployed initial Sixteen ML models, including six boosting algorithms, on a dataset of 9172 instances with related features. The model performances have been judged through various standard performance metrics. The boosting algorithms showed exceptional results, and Cat Boost (CB) model produced the best accuracy of 95.75%. The hyperparameter tuning performed on boosting models by implementing Randomized Search CV increased the accuracy to 96.19% for CB. The stacking ensemble approach was applied on top of the six boosting tuned models with the CB classifier as the meta-learner. At the same time, the other boosting algorithms were kept as a base learner for the final model prediction. The accuracy of the stack model was impressive, with 95.32% compared with default models, the ROC-AUC at 0.95, and the other results were also promising. The model’s standard deviation was significantly less at 0.57, implying the model’s stability and robustness, and the False Negative (FN) rate reached 1.8%.
更多查看译文
关键词
Machine Learning,Thyroid Disease Prediction,Cat Boost,Stacking,Boosting,Tuned Model,Accuracy,ROCAUC Score
AI 理解论文
溯源树
样例
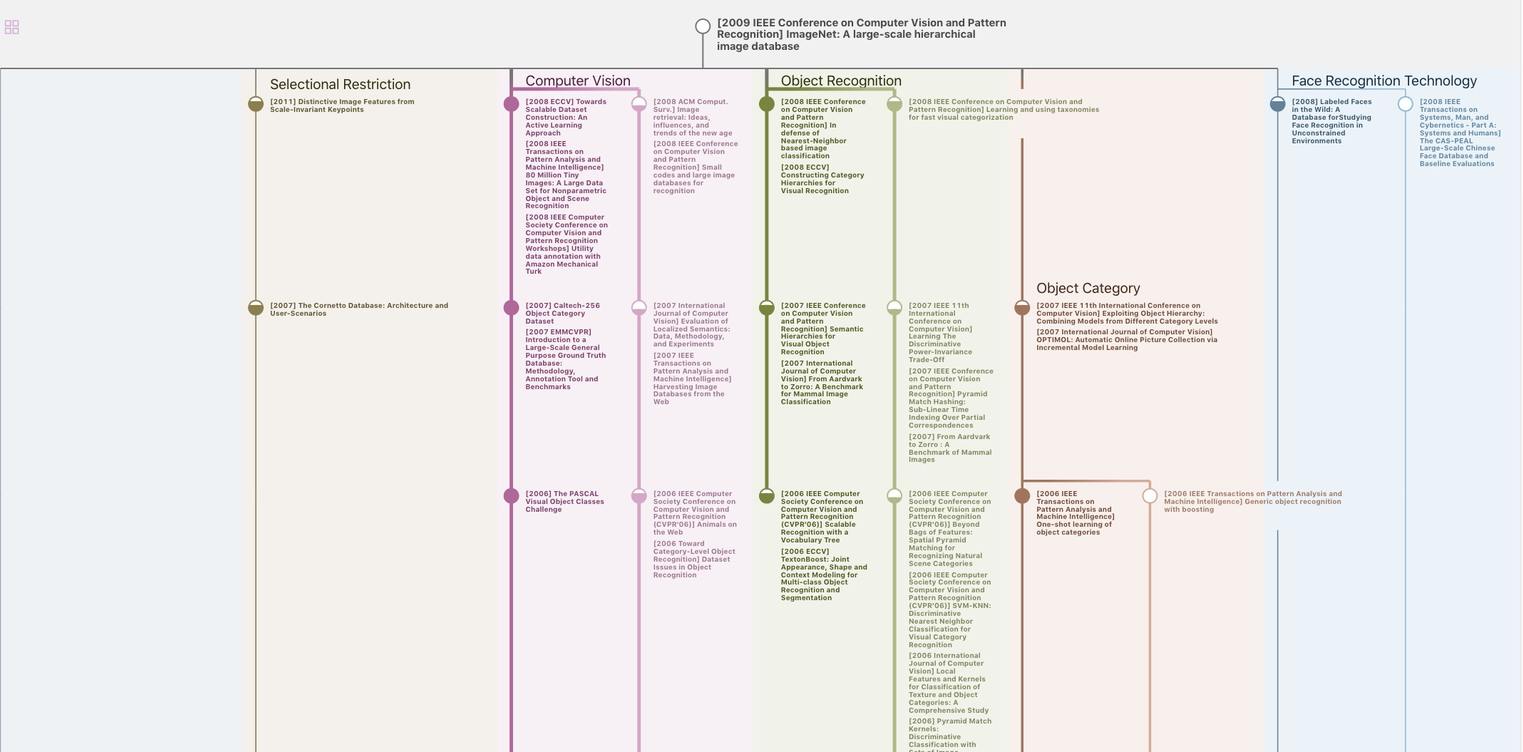
生成溯源树,研究论文发展脉络
Chat Paper
正在生成论文摘要