Improving Factuality and Reasoning in Language Models through Multiagent Debate
ICML 2024(2024)
Abstract
Large language models (LLMs) have demonstrated remarkable capabilities in language generation, understanding, and few-shot learning in recent years. An extensive body of work has explored how their performance may be further improved through the tools of prompting, ranging from verification, self-consistency, or intermediate scratchpads. In this paper, we present a complementary approach to improve language responses where multiple language model instances propose and debate their individual responses and reasoning processes over multiple rounds to arrive at a common final answer. Our findings indicate that this approach significantly enhances mathematical and strategic reasoning across a number of tasks. We also demonstrate that our approach improves the factual validity of generated content, reducing fallacious answers and hallucinations that contemporary models are prone to. Our approach may be directly applied to existing black-box models and uses identical procedure and prompts for all tasks we investigate. Overall, our findings suggest that such "society of minds" approach has the potential to significantly advance the capabilities of LLMs and pave the way for further breakthroughs in language generation and understanding.
MoreTranslated text
Key words
factuality,language models,debate,reasoning,multiagent
AI Read Science
Must-Reading Tree
Example
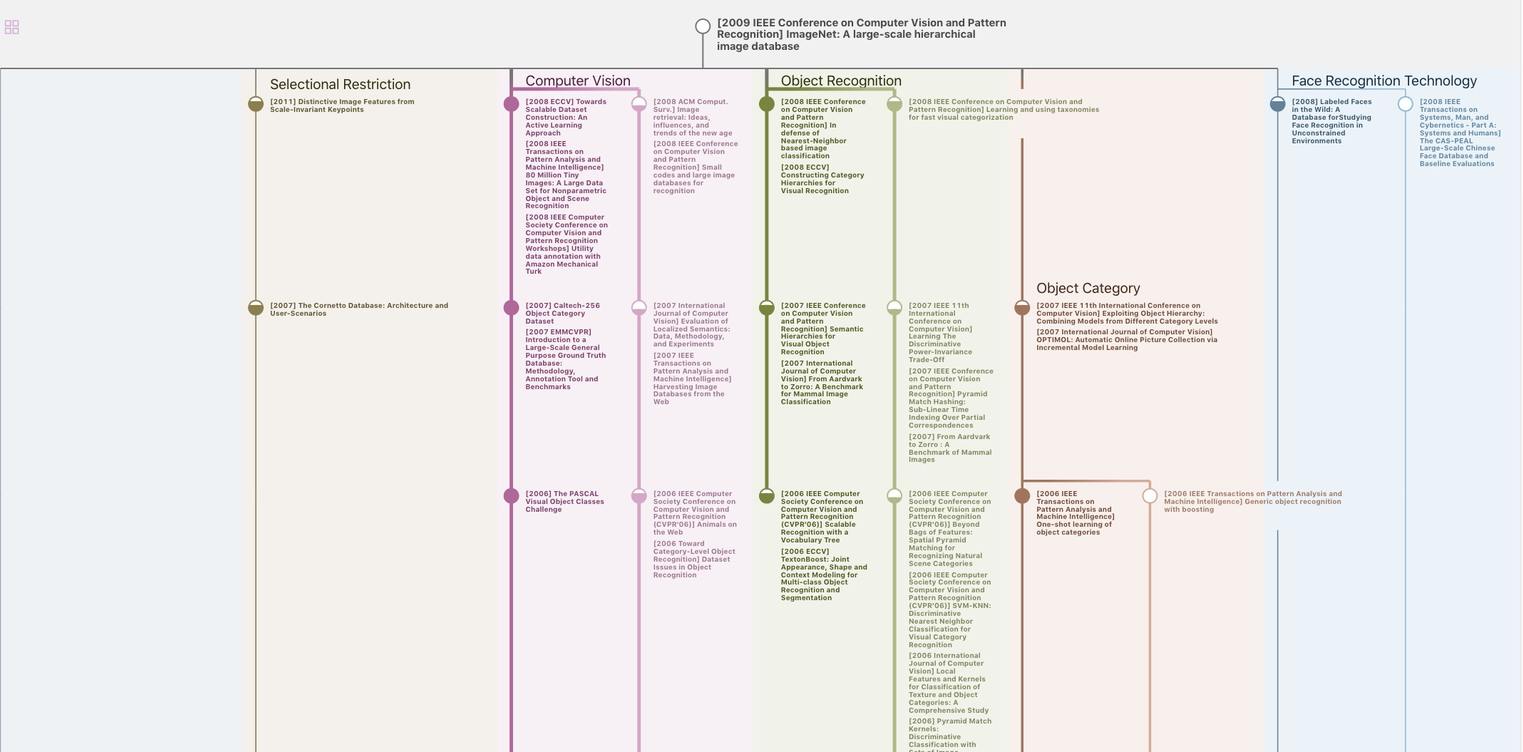
Generate MRT to find the research sequence of this paper
Chat Paper
Summary is being generated by the instructions you defined