Statistical Indistinguishability of Learning Algorithms
CoRR(2023)
摘要
When two different parties use the same learning rule on their own data, how can we test whether the distributions of the two outcomes are similar? In this paper, we study the similarity of outcomes of learning rules through the lens of the Total Variation (TV) distance of distributions. We say that a learning rule is TV indistinguishable if the expected TV distance between the posterior distributions of its outputs, executed on two training data sets drawn independently from the same distribution, is small. We first investigate the learnability of hypothesis classes using TV indistinguishable learners. Our main results are information-theoretic equivalences between TV indistinguishability and existing algorithmic stability notions such as replicability and approximate differential privacy. Then, we provide statistical amplification and boosting algorithms for TV indistinguishable learners.
更多查看译文
关键词
statistical indistinguishability,algorithms,learning
AI 理解论文
溯源树
样例
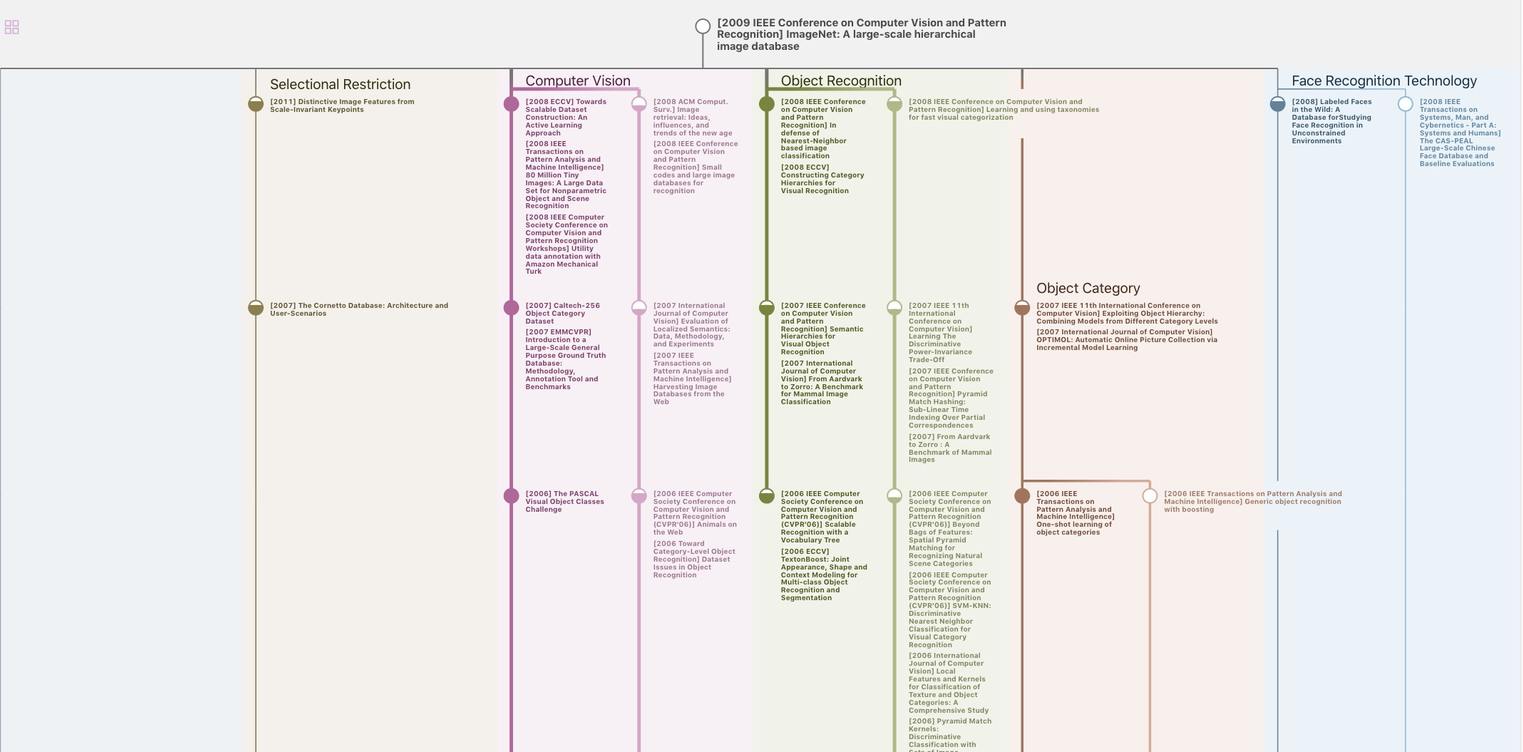
生成溯源树,研究论文发展脉络
Chat Paper
正在生成论文摘要