INSTRUCTSCORE: Towards Explainable Text Generation Evaluation with Automatic Feedback.
CoRR(2023)
摘要
The field of automatic evaluation of text generation made tremendous progress in the last few years. In particular, since the advent of neural metrics, like COMET, BLEURT, and SEScore2, the newest generation of metrics show a high correlation with human judgment. Unfortunately, quality scores generated with neural metrics are not interpretable, and it is unclear which part of the generation output is criticized by the metrics. To address this limitation, we present INSTRUCTSCORE, an open-source, explainable evaluation metric for text generation. By harnessing both explicit human instruction and the implicit knowledge of GPT4, we fine-tune a LLAMA model to create an evaluative metric that can produce a diagnostic report aligned with human judgment. We evaluate INSTRUCTSCORE on the WMT22 Zh-En translation task, where our 7B model surpasses other LLM-based baselines, including those based on 175B GPT3. Impressively, our INSTRUCTSCORE, even without direct supervision from human-rated data, achieves performance levels on par with state-of-the-art metrics like COMET22, which was fine-tuned on human ratings.
更多查看译文
AI 理解论文
溯源树
样例
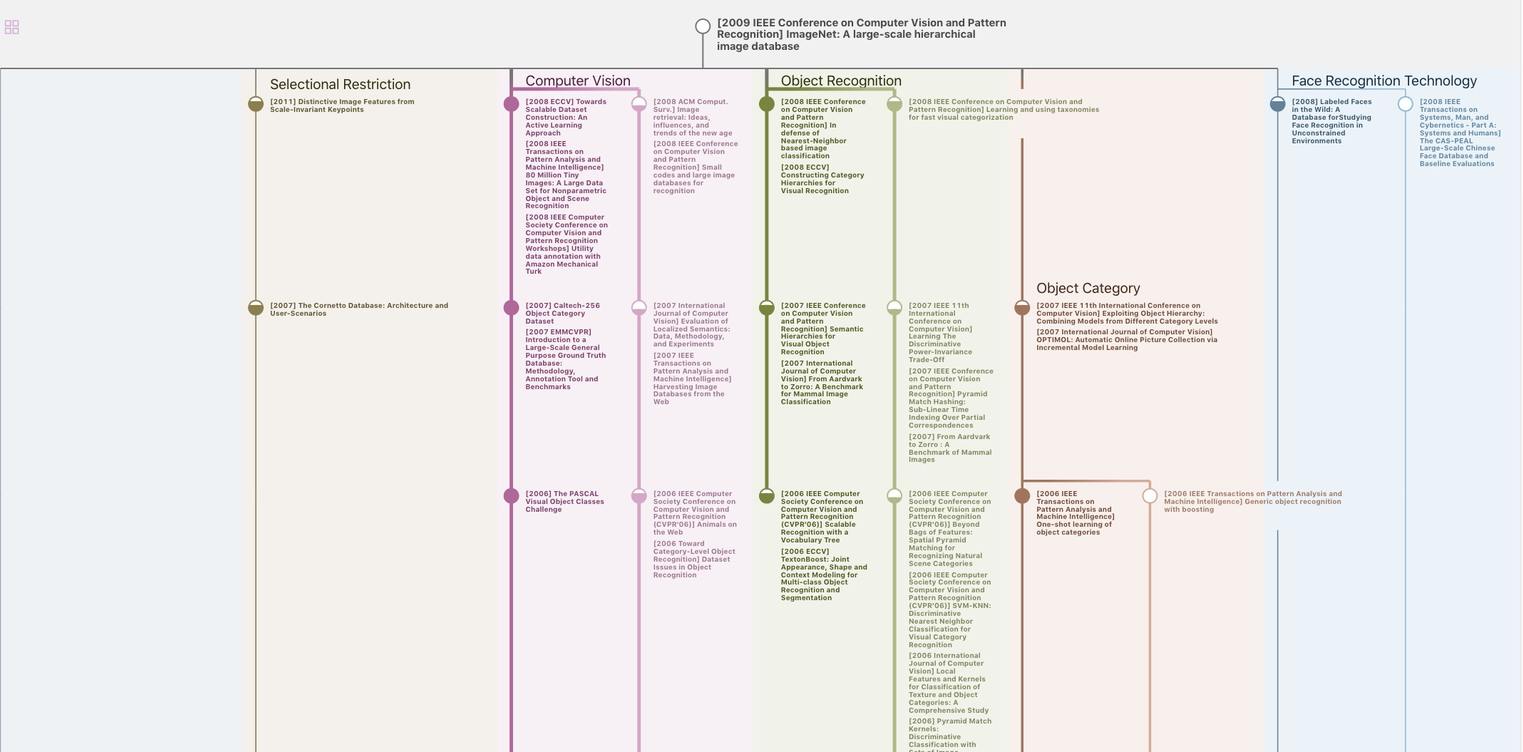
生成溯源树,研究论文发展脉络
Chat Paper
正在生成论文摘要