Distributed Inexact Newton Method with Adaptive Step Sizes
arXiv (Cornell University)(2023)
摘要
We consider two formulations for distributed optimization wherein $N$ agents in a generic connected network solve a problem of common interest: distributed personalized optimization and consensus optimization. A new method termed DINAS (Distributed Inexact Newton method with Adaptive Stepsize) is proposed. DINAS employs large adaptively computed step-sizes, requires a reduced global parameters knowledge with respect to existing alternatives, and can operate without any local Hessian inverse calculations nor Hessian communications. When solving personalized distributed learning formulations, DINAS achieves quadratic convergence with respect to computational cost and linear convergence with respect to communication cost, the latter rate being independent of the local functions condition numbers or of the network topology. When solving consensus optimization problems, DINAS is shown to converge to the global solution. Extensive numerical experiments demonstrate significant improvements of DINAS over existing alternatives. As a result of independent interest, we provide for the first time convergence analysis of the Newton method with the adaptive Polyak's step-size when the Newton direction is computed inexactly in centralized environment.
更多查看译文
关键词
inexact newton method,newton method,step
AI 理解论文
溯源树
样例
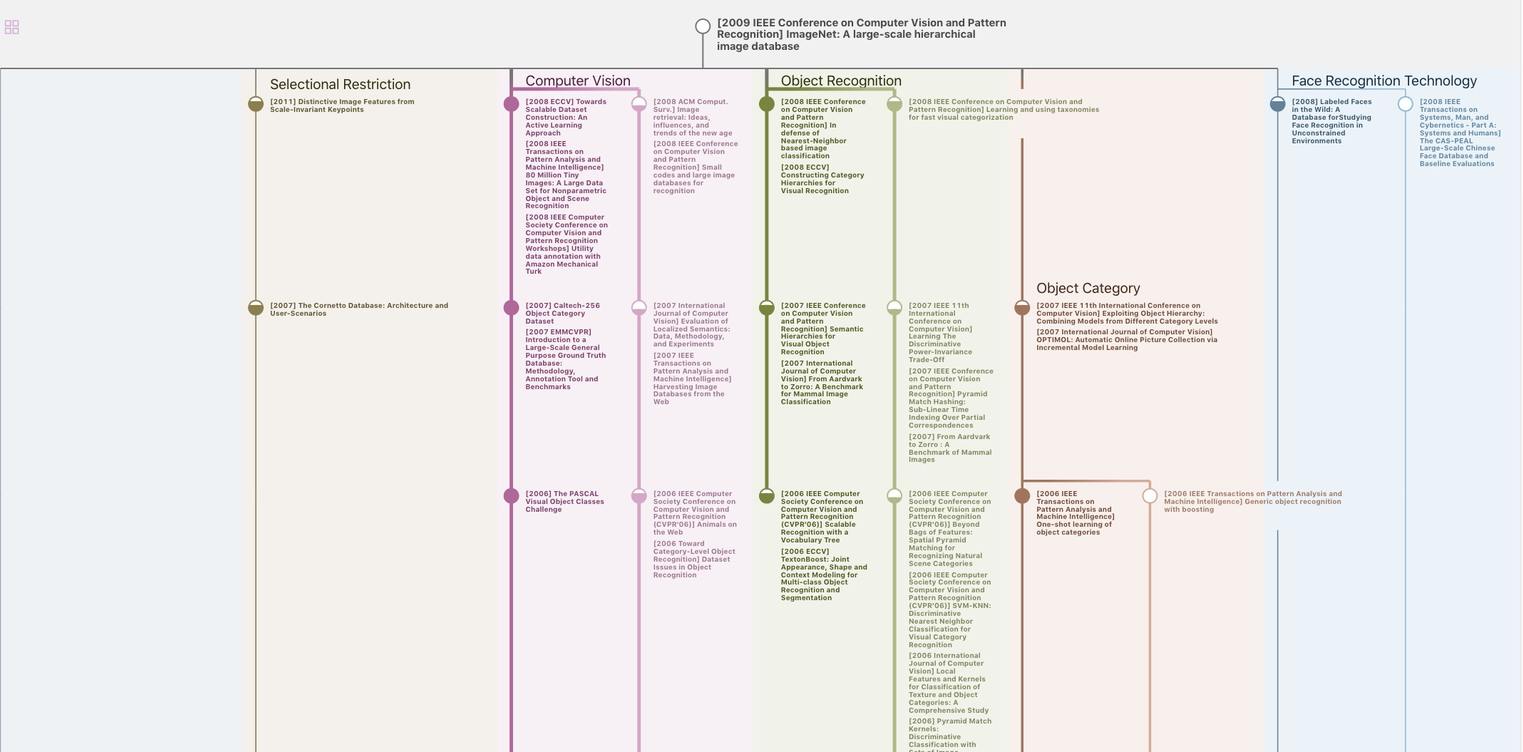
生成溯源树,研究论文发展脉络
Chat Paper
正在生成论文摘要