Prompt-Based Monte-Carlo Tree Search for Goal-oriented Dialogue Policy Planning.
CoRR(2023)
Abstract
Planning for goal-oriented dialogue often requires simulating future dialogue interactions and estimating task progress. Many approaches thus consider training neural networks to perform look-ahead search algorithms such as A* search and Monte Carlo Tree Search (MCTS). However, this training often require abundant annotated data, which creates challenges when faced with noisy annotations or low-resource settings. We introduce GDP-Zero, an approach using Open-Loop MCTS to perform goal-oriented dialogue policy planning without any model training. GDP-Zero prompts a large language model to act as a policy prior, value function, user simulator, and system model during the tree search. We evaluate GDP-Zero on the goal-oriented task PersuasionForGood, and find that its responses are preferred over ChatGPT up to 59.32% of the time, and are rated more persuasive than ChatGPT during interactive evaluations.
MoreTranslated text
Key words
dialogue policy
AI Read Science
Must-Reading Tree
Example
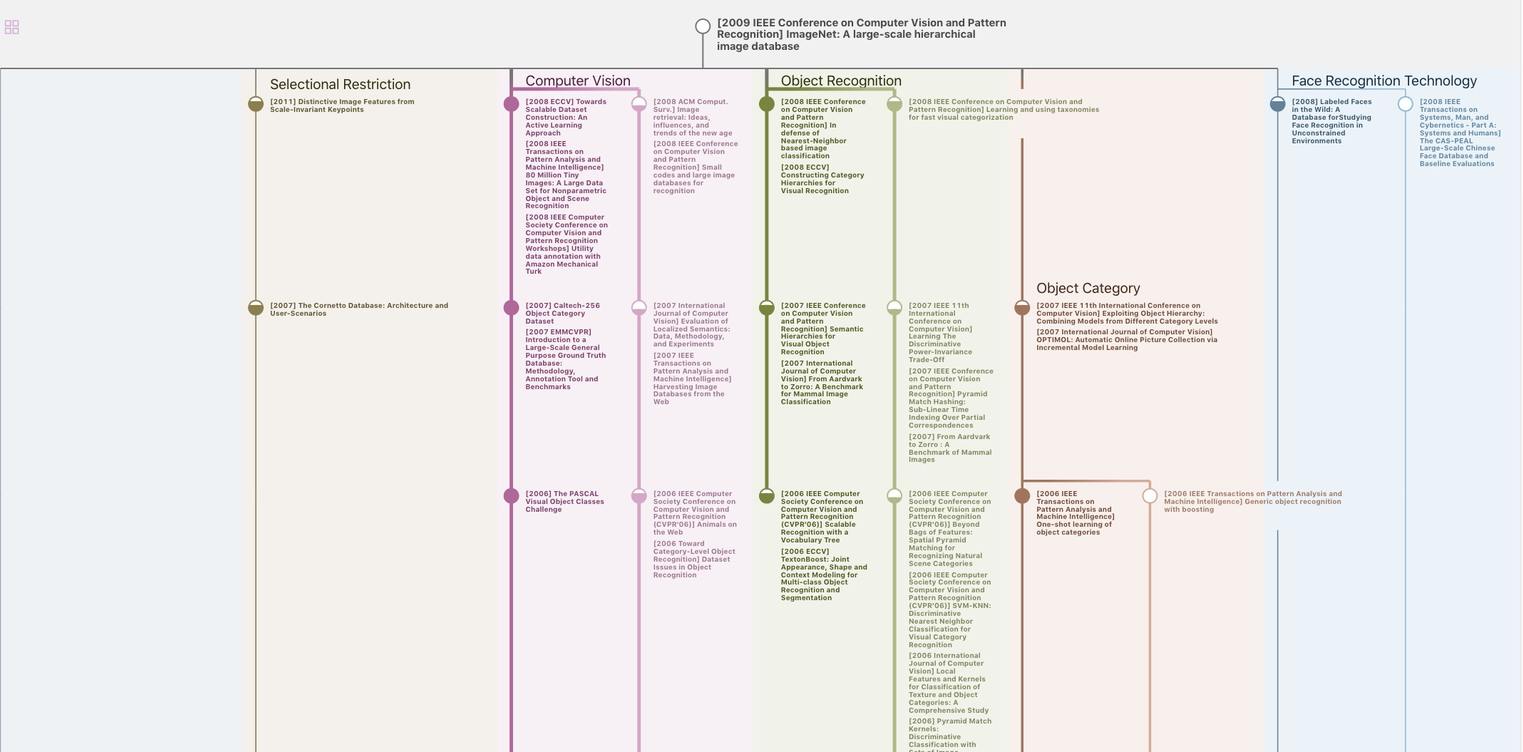
Generate MRT to find the research sequence of this paper
Chat Paper
Summary is being generated by the instructions you defined