Non-parametric, Nearest-neighbor-assisted Fine-tuning for Neural Machine Translation
CoRR(2023)
摘要
Non-parametric, k-nearest-neighbor algorithms have recently made inroads to assist generative models such as language models and machine translation decoders. We explore whether such non-parametric models can improve machine translation models at the fine-tuning stage by incorporating statistics from the kNN predictions to inform the gradient updates for a baseline translation model. There are multiple methods which could be used to incorporate kNN statistics and we investigate gradient scaling by a gating mechanism, the kNN's ground truth probability, and reinforcement learning. For four standard in-domain machine translation datasets, compared with classic fine-tuning, we report consistent improvements of all of the three methods by as much as 1.45 BLEU and 1.28 BLEU for German-English and English-German translations respectively. Through qualitative analysis, we found particular improvements when it comes to translating grammatical relations or function words, which results in increased fluency of our model.
更多查看译文
关键词
neural machine
AI 理解论文
溯源树
样例
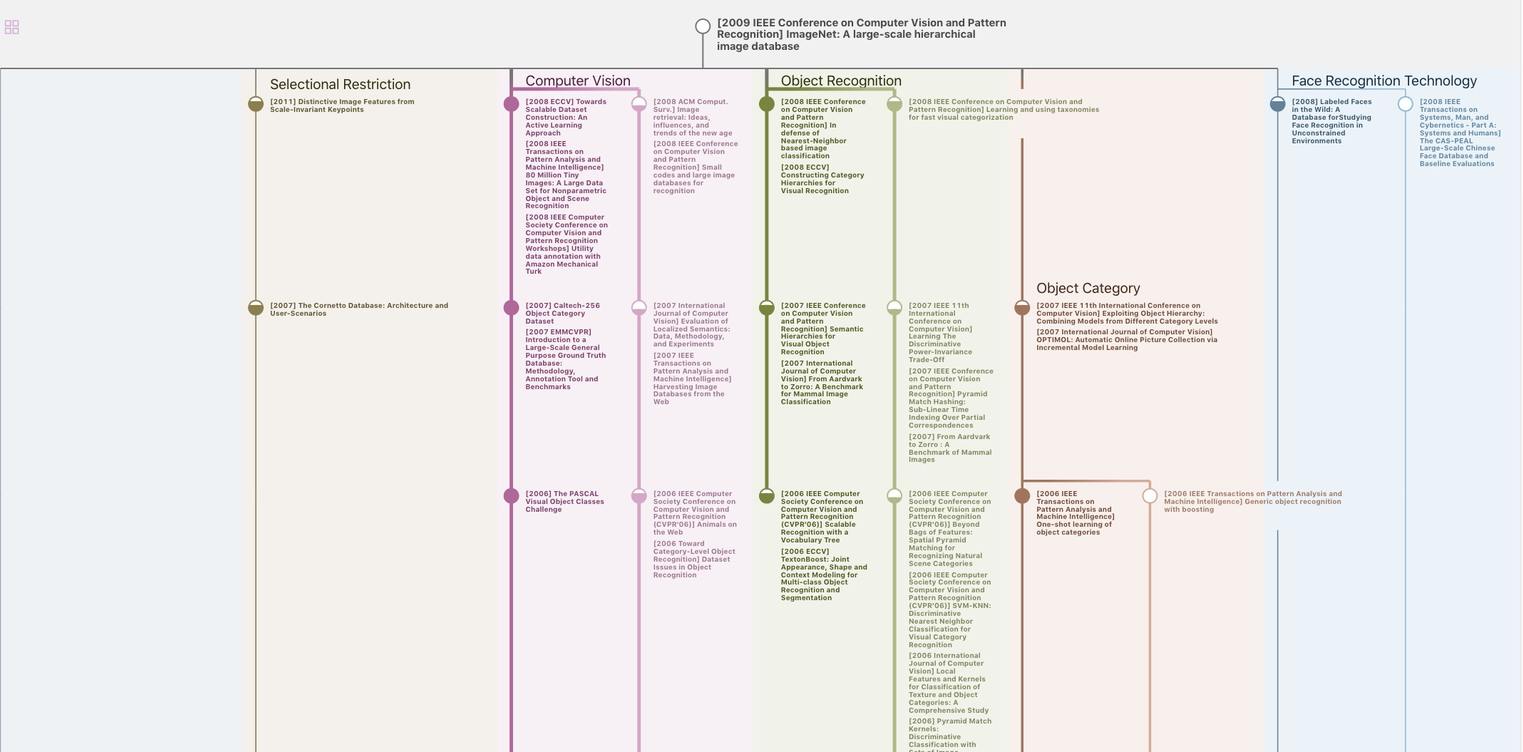
生成溯源树,研究论文发展脉络
Chat Paper
正在生成论文摘要