Open-world Semi-supervised Generalized Relation Discovery Aligned in a Real-world Setting.
CoRR(2023)
摘要
Open-world Relation Extraction (OpenRE) has recently garnered significant attention. However, existing approaches tend to oversimplify the problem by assuming that all unlabeled texts belong to novel classes, thereby limiting the practicality of these methods. We argue that the OpenRE setting should be more aligned with the characteristics of real-world data. Specifically, we propose two key improvements: (a) unlabeled data should encompass known and novel classes, including hard-negative instances; and (b) the set of novel classes should represent long-tail relation types. Furthermore, we observe that popular relations such as titles and locations can often be implicitly inferred through specific patterns, while long-tail relations tend to be explicitly expressed in sentences. Motivated by these insights, we present a novel method called KNoRD (Known and Novel Relation Discovery), which effectively classifies explicitly and implicitly expressed relations from known and novel classes within unlabeled data. Experimental evaluations on several Open-world RE benchmarks demonstrate that KNoRD consistently outperforms other existing methods, achieving significant performance gains.
更多查看译文
关键词
relation,discovery,open-world,semi-supervised,real-world
AI 理解论文
溯源树
样例
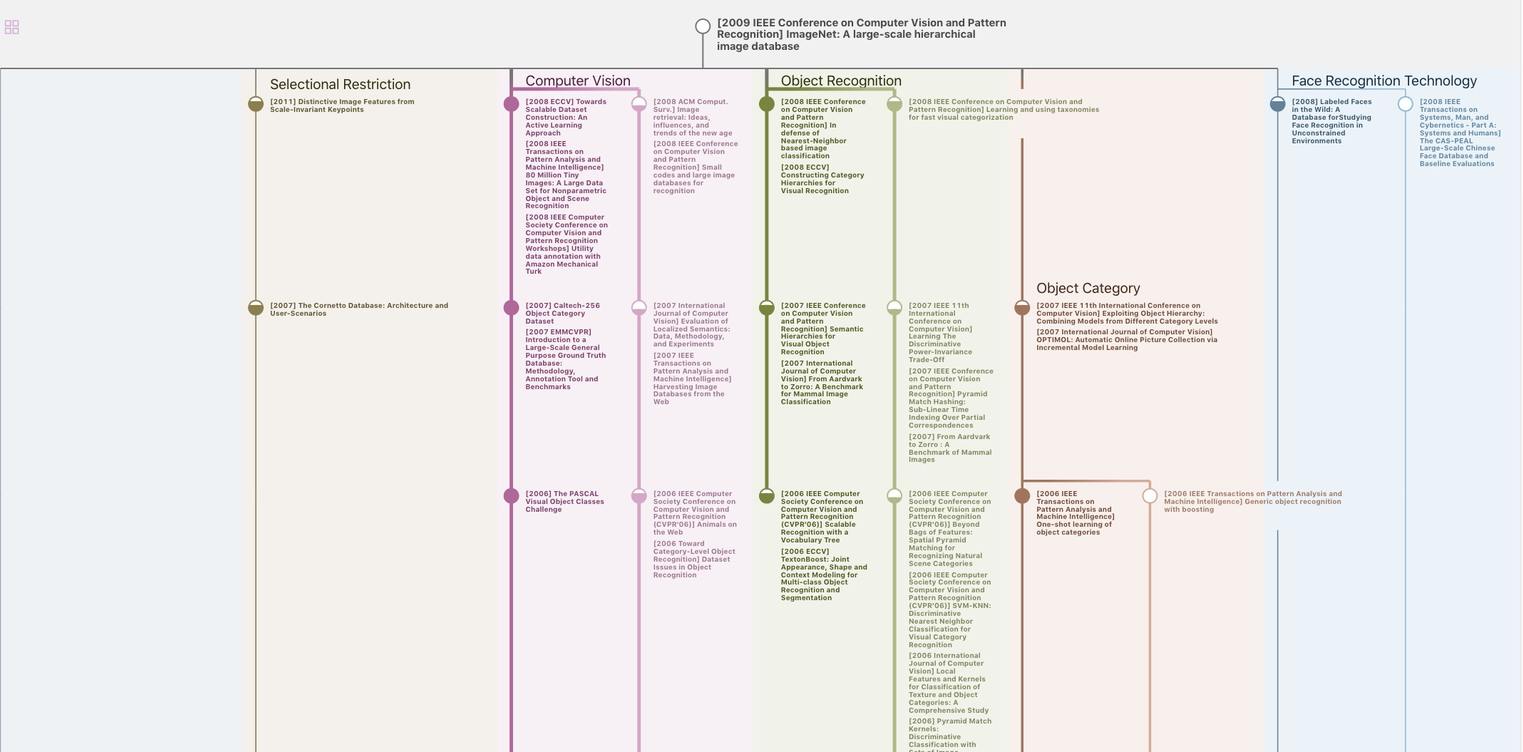
生成溯源树,研究论文发展脉络
Chat Paper
正在生成论文摘要