Improved ResNet Image Classification Model Based on Tensor Synthesis Attention
Laser & Optoelectronics Progress(2023)
摘要
An improved ResNet- 101 network model that fuses tensor synthesis attention ( RTSA Net- 101) is proposed to solve insufficient feature extraction and the indiscriminate contribution of the extracted features when processing image classification tasks using a convolutional neural network. First, the image features are extracted using a Resnet-101 backbone network and the tensor synthesis attention module is embedded after the convolution structure of the residual network. The features are calculated using a three-tensor product to obtain the attention feature matrix. Next, the Softmax function is used to normalize the attention feature matrix to assign weights to features and distinguish the contribution of features. Finally, the weighted sum of the weights and critical values are calculated as the final features in our proposed method to improve the image classification performance. Comparative experiments are conducted on natural image datasets, CIFAR-10 and CIFAR-100, and street brand dataset, SVHN. The classification accuracy values of the models are 96. 12%, 81. 60%, and 96. 67%, respectively, and the average test running time of the images are 0. 0258 s, 0. 0260 s, and 0. 0262 s, respectively. The experimental results show that compared with the other seven advanced image classification models, the RTSA Net-101 model can achieve higher classification accuracy and shorter test run time, and it can effectively enhance the feature learning ability of the network, thereby render the proposed model innovative and efficient.
更多查看译文
关键词
tensor synthesis attention,residual network,self-attention,feature extraction,image classification
AI 理解论文
溯源树
样例
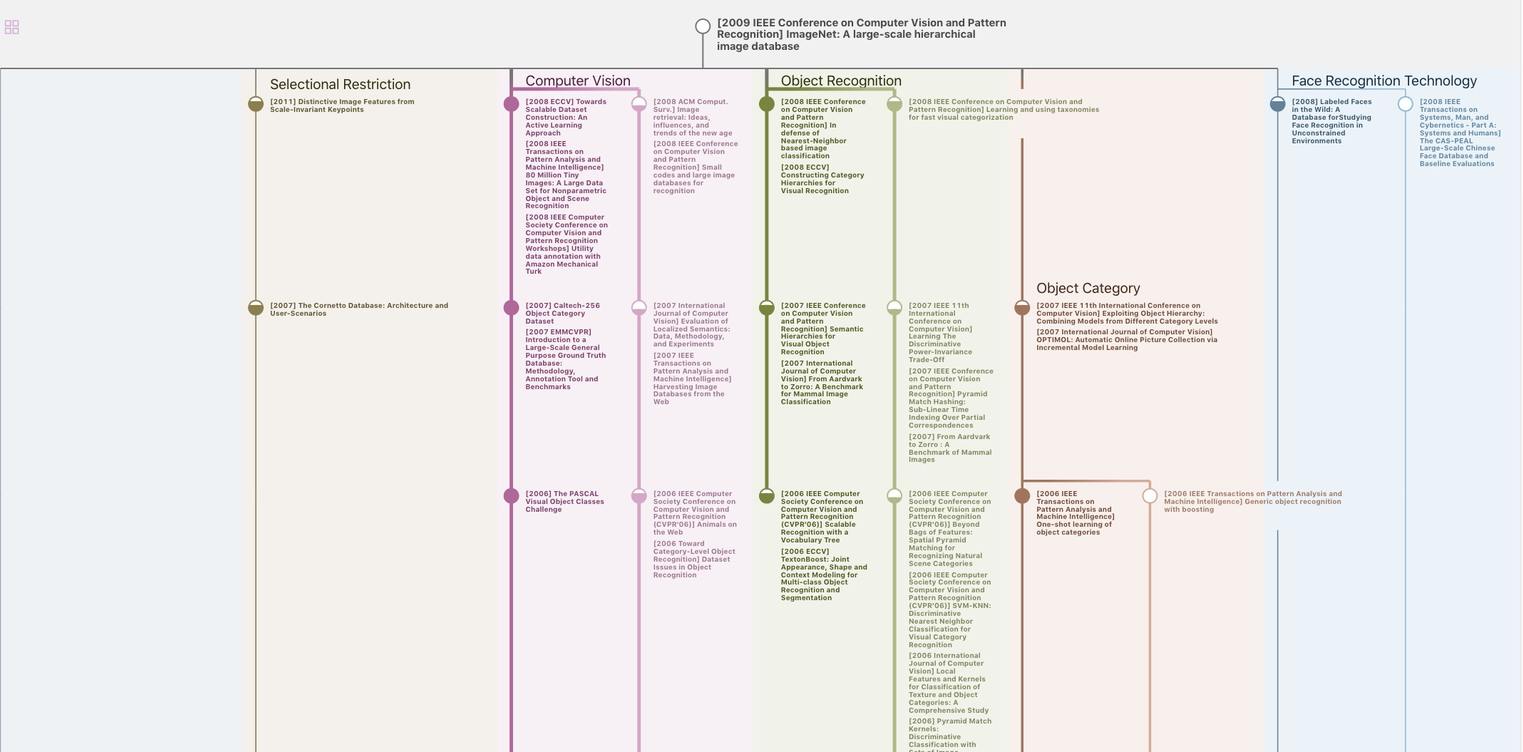
生成溯源树,研究论文发展脉络
Chat Paper
正在生成论文摘要