Multi-Feature Convergence Network for Acoustic Scene Classification.
Proceedings of the 2022 6th International Conference on Electronic Information Technology and Computer Engineering(2022)
摘要
This paper investigates a multi-feature convergence network for acoustic scene classification (ASC). A series of neural network models designed with features of the Log Mel spectrogram, Deltas, and Delta-Deltas superimposed on the channel have achieved good classification results. However, the low-frequency part of the speech spectrogram feature extracted from the audio signal has a mosaic shape due to its low resolution, which leads to the loss of information in the low-frequency part of the Log Mel-Deltas-DeltaDeltas feature and reduces the classification accuracy. To solve this problem, the constant Q-transform (CQT) spectrogram is introduced and this feature is superimposed on the channel with the log Mel-Deltas-DeltaDeltas feature to form a 4-channel feature spectrum as the input to the network model. Moreover, the proposed network model is deepened by increasing the 8 residual blocks from the baseline system to 10 residual blocks and a snapshot integration operation is performed on the various models saved during the training process due to the complementary information. And then, a 3-classifier is added based on the ASC's primarily categorized scenes' 10-classifier and chooses the final scene classification by combining the 3–10 two-stage classification scores. The classification accuracy of our proposed network reached 77.4%, which is 5.1% higher than the baseline system set in this paper and 26% higher than the baseline on the official website of DCASE 2020.
更多查看译文
AI 理解论文
溯源树
样例
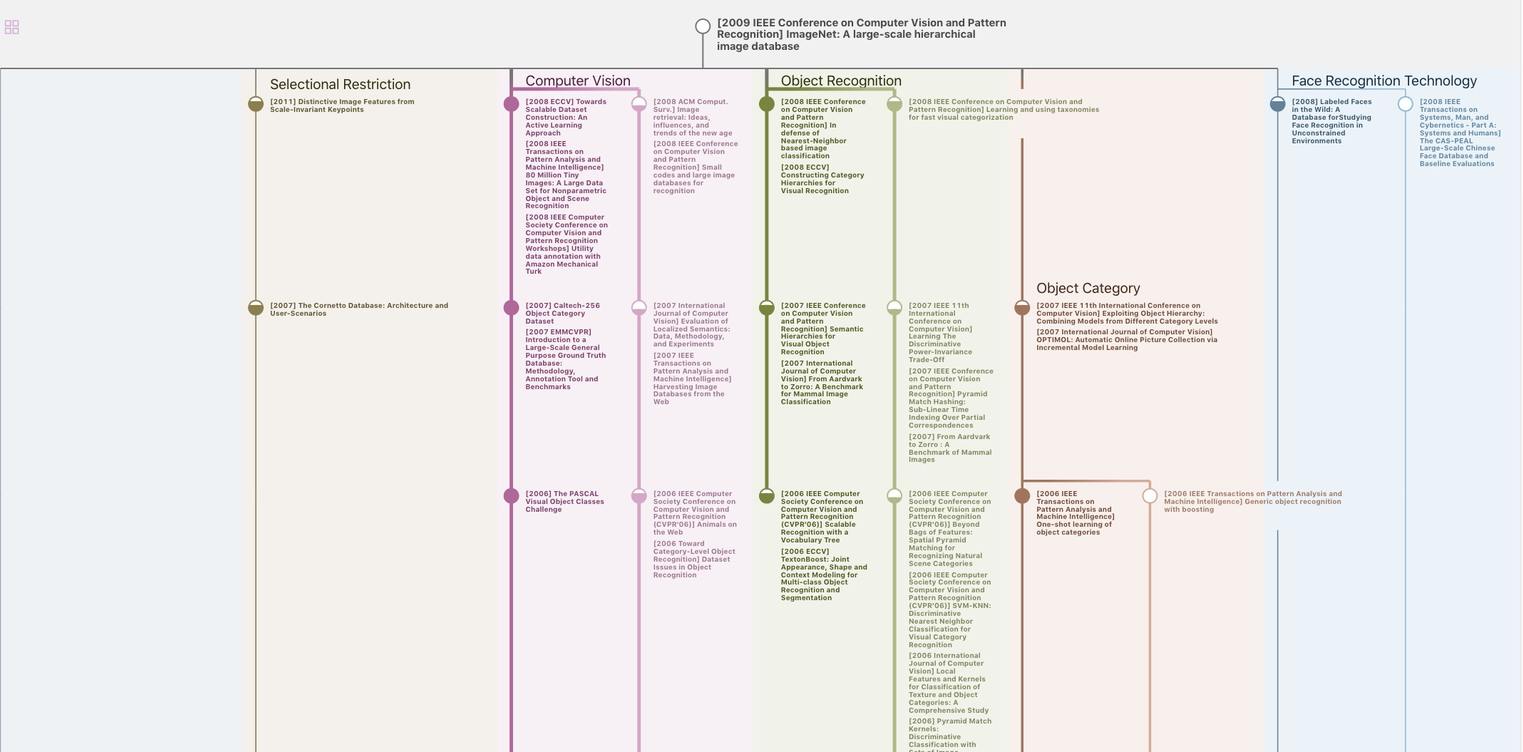
生成溯源树,研究论文发展脉络
Chat Paper
正在生成论文摘要