Image Super-Resolution Based on Gated Residual and Gated Convolution Networks
Neural Processing Letters(2023)
Abstract
Single image super-resolution based on deep neural network has been a hot topic in recent years. In this paper, we propose a gated residual and gated convolution network (GRGCN) to deal with super-resolution reconstruction. Gated residual (GR) and gated convolution (GC) are the products of introducing attention mechanism (AM) into deep neural network. Specifically, GR enables the network to focus on more important residual channel information and GC allows the network to pay attention on more important feature extraction regions. Meanwhile, we propose multi-level residual connection, which includes long connection, secondary connection and short connection. Three different levels of residual connection correspond to three scales of feature extraction module, namely residual cluster, residual group and residual block. By fusing the feature information on different scales, the convolution layer can efficiently extract the feature information. Experiments on benchmark datasets demonstrate that our proposed GRGCN has better performance and visual effects than state-of-the-art methods.
MoreTranslated text
Key words
Super-resolution,Multi-level residual,Gated residual,Gated convolution
AI Read Science
Must-Reading Tree
Example
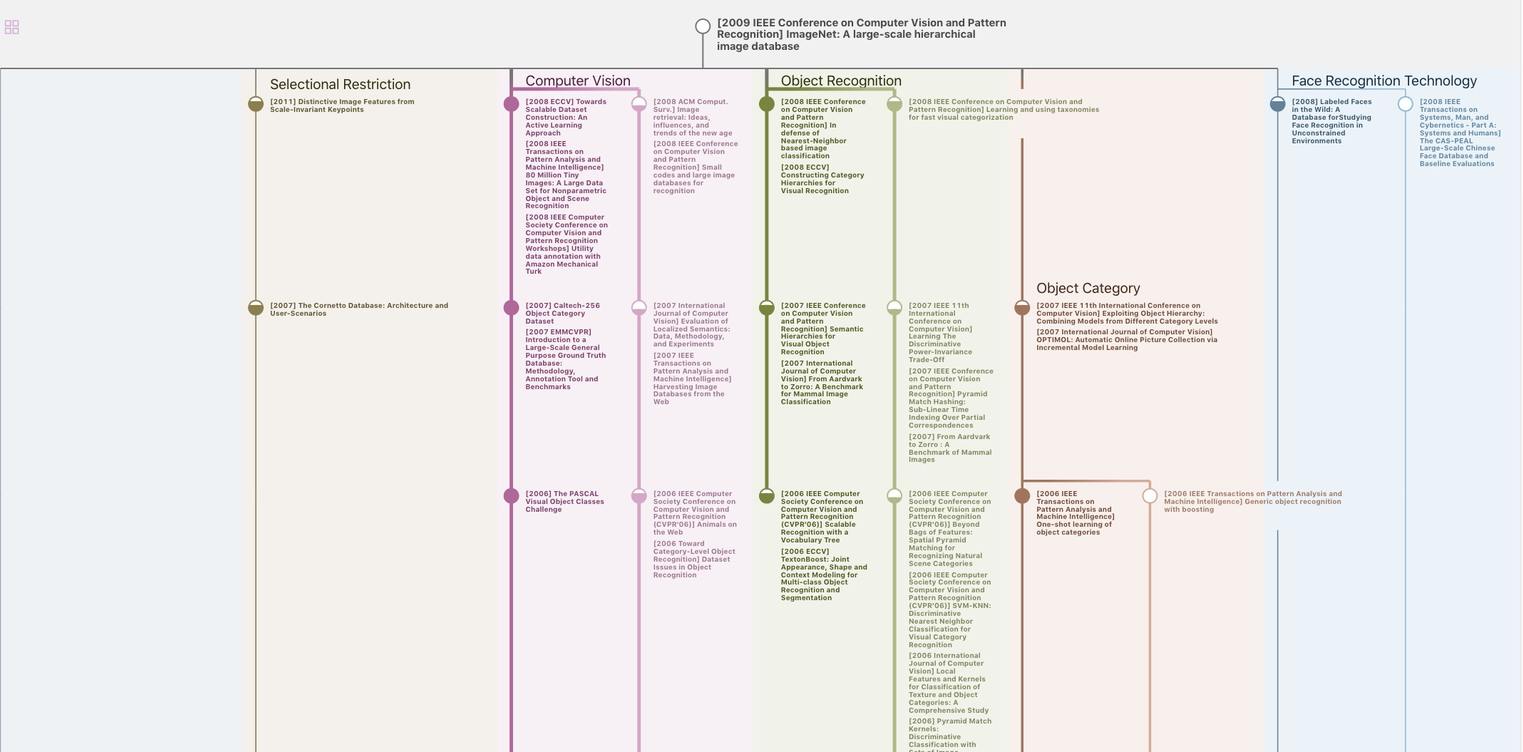
Generate MRT to find the research sequence of this paper
Chat Paper
Summary is being generated by the instructions you defined