Prediction of severe haemolysis during extracorporeal membrane oxygenation (ECMO) using multiple machine learning
Research Square (Research Square)(2023)
摘要
Abstract Objective We examine whether machine learning can be used to predict severe haemolysis in patients during extracorporeal membrane oxygenation. Methods The present study is a reanalysis of public data from 1063 ECMO patients. We trained the corresponding model using 5 machine learning and built a machine learning prediction model in Python. Results The top 5 factors found to influence haemolysis by data analysis were Sequential Organ Failure Assessment(SOFA), pump head thrombosis(PHT), platelet concentrate(PC)/ days, lactate dehydrogenase(LDH) pre, and fresh frozen plasma(FFP)/days, respectively. In the training group, among the algorithms, the highest AUC values rate was that of GradientBoosting (0.886). Our validation in the test group by different machine learning algorithms found that the three algorithms with the highest AUC values were 0.806, 0.781, and 0.759 for XGB, GradientBoosting, and Randomforest, respectively. In addition, among the algorithms, XGB had the highest accuracy with a value of 0.913. Conclusions According to our results, XGB performed best overall, with an AUC >0.8, an accuracy >90%. Besides, the top 5 factors found to influence haemolysis by data analysis were SOFA, PHT, PC/days, LDH pre, and FFP/days. Therefore, machine learning studies have better predictive value for whether patients develop severe haemolysis during ECMO.
更多查看译文
关键词
extracorporeal membrane oxygenation,severe haemolysis,ecmo
AI 理解论文
溯源树
样例
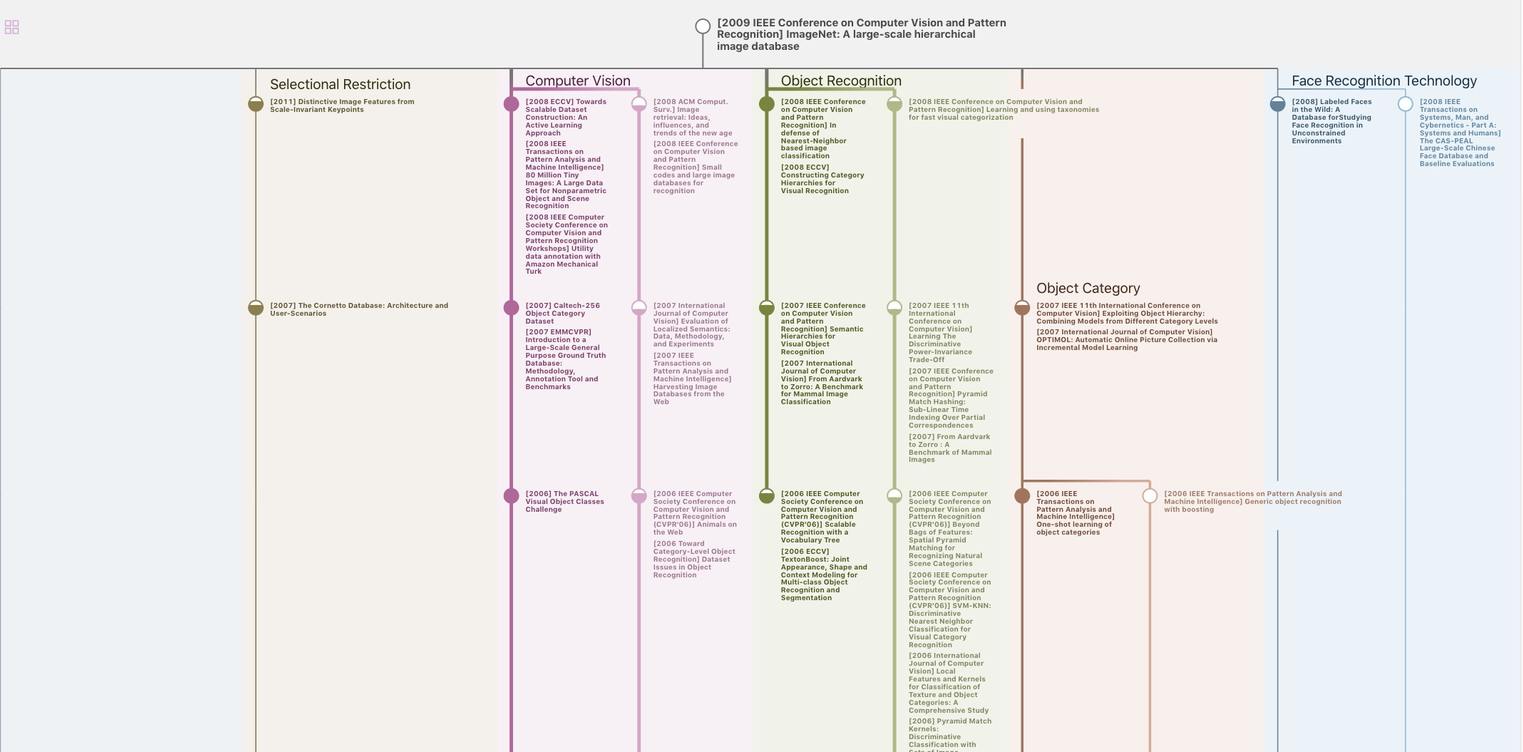
生成溯源树,研究论文发展脉络
Chat Paper
正在生成论文摘要