Mp14-05 extracellular vesicle biomarker signature detection platform for guiding cancer detection and therapy
Journal of Urology(2023)
摘要
You have accessJournal of UrologyCME1 Apr 2023MP14-05 EXTRACELLULAR VESICLE BIOMARKER SIGNATURE DETECTION PLATFORM FOR GUIDING CANCER DETECTION AND THERAPY Thomas Osinski, Samuel Walker, Karen Doersch, Jonathan Flax, and James McGrath Thomas OsinskiThomas Osinski More articles by this author , Samuel WalkerSamuel Walker More articles by this author , Karen DoerschKaren Doersch More articles by this author , Jonathan FlaxJonathan Flax More articles by this author , and James McGrathJames McGrath More articles by this author View All Author Informationhttps://doi.org/10.1097/JU.0000000000003234.05AboutPDF ToolsAdd to favoritesDownload CitationsTrack CitationsPermissionsReprints ShareFacebookLinked InTwitterEmail Abstract INTRODUCTION AND OBJECTIVE: Extracellular Vesicles (EVs) have tremendous potential in cancer diagnostics and personalized cancer therapeutics. They are abundant in all biofluids, carry molecular markers of their cell-of-origin and can be readily obtained via noninvasive techniques. Current EV biomarker assessments have several limitiations. Pooled EVs from samples have low detection sensitivity for less abundant biomarkers and preclude colocalization of biomarkers on individual EVs. Currently, expensive specialized devices requiring large biofluid inputs are needed for more detailed EV analysis. Here, we develop an easy to use and inexpensive device that permits optical detection of multiple markers on individual EVs using only 10 µl of serum. This work investigates whether the microfluidic strategy we call ‘catch and display for liquid biopsy [CAD-LB]’ can detect biomarkers on single EVs. We also sought to determine the dynamic range of accurate quantitation using purified EVs and serum and validate detection of immune checkpoint proteins (PD-L1 and/or PD-1), which may help to distinguish immune checkpoint immunotherapy (ICI) responders from non-responders in bladder cancer. METHODS: For this study EVs were derived from: 1. ultracentrifuge purified urinary EVs; 2. EVs from media of 5637 bladder cancer and primary bladder epithelium cell lines and; 3. Serum from patients with advanced urothelial carcinoma or healthy controls. EVs were labeled with the pan-EV marker carboxyfluorescein succinimidyl ester and fluorescently labeled primary antibodies. Small volumes (10 µL) of solutions were captured on our membrane with nanopores in a transparent membrane and imaged using fluorescence microscopy. Detection range was assessed by serial dilutions of EVs. EV surface antigen labeling was assessed by CAD-LB. RESULTS: 1. Counting curves demonstrate a wide dynamic range of CAD-LB (102 – 106 EVs) for purified EVs while serum plateaus at lower inputs (∼104 EVs). 2. CAD-LB detects PD-1 and PD-L1 biomarkers in urothelial carcinoma patients’ serum. 3. CAD-LB detects T cell biomarker CD3 on approximately 1-2% of EVs in healthy control serum. CONCLUSIONS: CAD-LB can detect rare and clinically significant EV associated biomarkers in conditioned medium and patient serum samples. Furthermore, CAD-LB provides single EV characterization with small sample volumes. These qualities make CAD-LB a potentially useful tool to help discover novel markers that could aid in diagnosis and treatment decisions for cancer patients. Source of Funding: None © 2023 by American Urological Association Education and Research, Inc.FiguresReferencesRelatedDetails Volume 209Issue Supplement 4April 2023Page: e183 Advertisement Copyright & Permissions© 2023 by American Urological Association Education and Research, Inc.MetricsAuthor Information Thomas Osinski More articles by this author Samuel Walker More articles by this author Karen Doersch More articles by this author Jonathan Flax More articles by this author James McGrath More articles by this author Expand All Advertisement PDF downloadLoading ...
更多查看译文
关键词
cancer detection
AI 理解论文
溯源树
样例
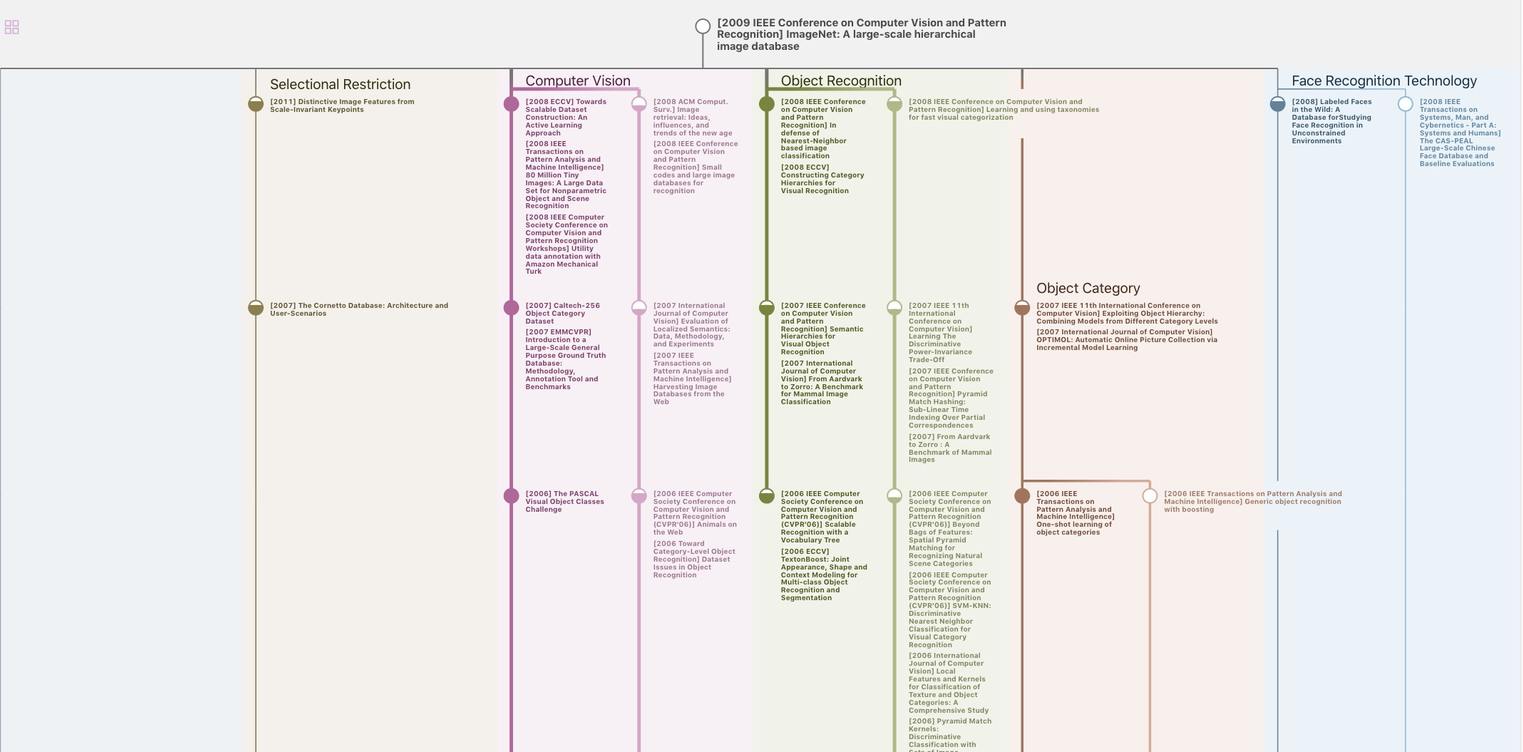
生成溯源树,研究论文发展脉络
Chat Paper
正在生成论文摘要