Clustered FedStack: Intermediate Global Models with Bayesian Information Criterion
PATTERN RECOGNITION LETTERS(2024)
摘要
Federated Learning (FL) is currently one of the most popular technologies in the field of Artificial Intelligence (AI) due to its collaborative learning and ability to preserve client privacy. However, it faces challenges such as non-identically and non-independently distributed (non-IID) data with imbalanced labels among local clients. To address these limitations, the research community has explored various approaches such as using local model parameters, federated generative adversarial learning, and federated representation learning. In our study, we propose a novel Clustered FedStack framework based on the previously published Stacked Federated Learning (FedStack) framework. Here, the local clients send their model predictions and output layer weights to a server, which then builds a robust global model. This global model clusters the local clients based on their output layer weights using a clustering mechanism. We adopt three clustering mechanisms, namely K-Means, Agglomerative, and Gaussian Mixture Models, into the framework and evaluate their performance. Bayesian Information Criterion (BIC) is used with the maximum likelihood function to determine the number of clusters. Our results show that Clustered FedStack models outperform baseline models with clustering mechanisms. To estimate the convergence of our proposed framework, we use Cyclical learning rates.
更多查看译文
关键词
Federated learning,FedStack,Clustering,Bayesian,Cyclical learning rates
AI 理解论文
溯源树
样例
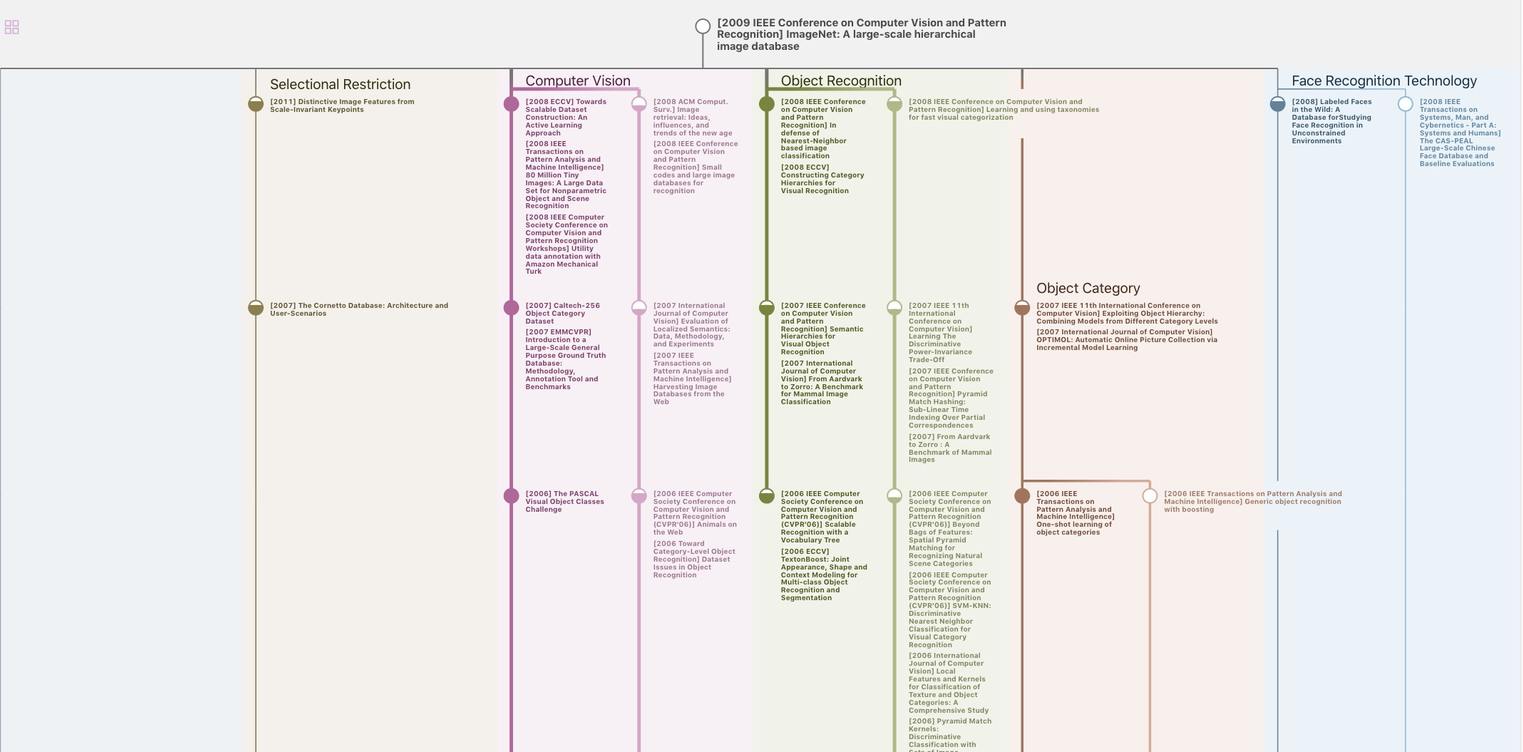
生成溯源树,研究论文发展脉络
Chat Paper
正在生成论文摘要