B-Spline Wavelet Neural-Network-Based Adaptive Control for Linear-Motor-Driven Systems via a Novel Gradient Descent Algorithm
IEEE TRANSACTIONS ON INDUSTRIAL ELECTRONICS(2024)
摘要
This article proposes a gradient descent (GD) algorithm-based B-spline wavelet neural network (GDBSWNN) learning adaptive controller for linear motor (LM) systems under system uncertainties and actuator saturation constraints. A recursive-least-squares-algorithm-based indirect adaptive strategy is used to effectively estimate model parameters, which can guarantee that they converge to true values. A novel GDBSWNN compensator is proposed to estimate the remaining complex uncertainties, where weights are updated by online GD training. An auxiliary system is integrated into the control scheme to address the saturation problem, which guarantees stability and satisfactory control performance when saturation occurs. In addition, a stability analysis is presented to prove that all the signals of the closed-loop system are bounded using the Lyapunov theory. Experiments have been conducted on an LM-driven motion platform, where different controllers have been tested, demonstrating the effectiveness and advantages of the proposed approach.
更多查看译文
关键词
Actuator saturation,B-spline wavelet neu-ral network (BSWNN),gradient descent (GD),indirect adap-tation,linear motor (LM)
AI 理解论文
溯源树
样例
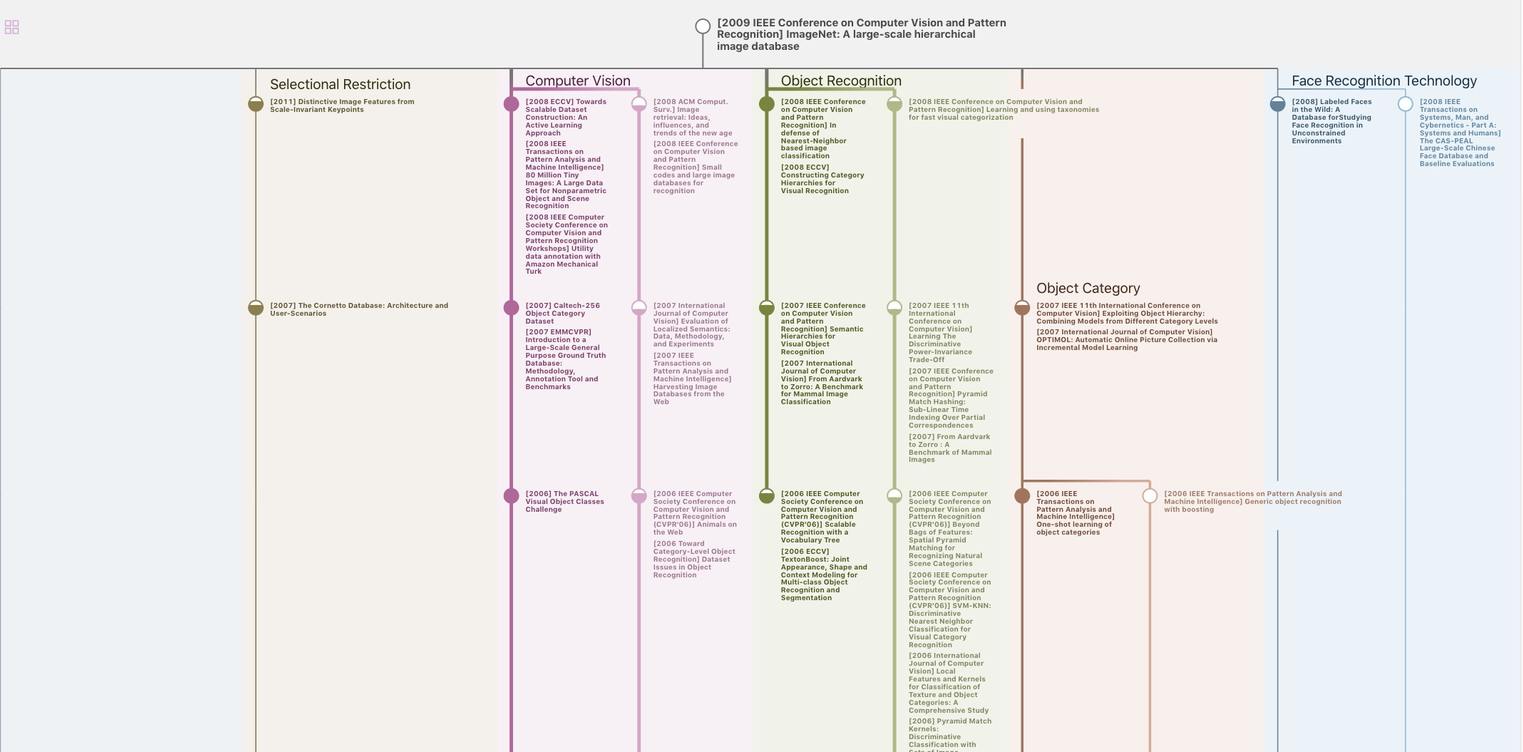
生成溯源树,研究论文发展脉络
Chat Paper
正在生成论文摘要