Lithology identification based on interpretability integration learning
EARTH SCIENCE INFORMATICS(2023)
Abstract
An interpretability model for intelligent lithology identification is proposed, which utilizes Ensemble Learning Stacking, Permutation Importance (PI), and Local Interpretable Model-agnostic Explanations (LIME) techniques. The aim of this method is to provide more accurate geological information and scientific support for oil and gas resource exploration. Two logging datasets from the public domain were used as experiments, and support vector machine (SVM), random forest (RF), and naive Bayes (NB) were employed as base learners, while SVM was utilized as the meta learner for lithology classification via stacking algorithm. The accuracy of the model was verified using evaluation metrics such as Area Under Curve (AUC), precision, recall, and F1-score. The PI and LIME techniques were employed to explain the lithology identification model. The results indicate that the stacking algorithm produced the best indexes and highest prediction accuracy. With respect to overall interpretation, PHIND, GR, and RT were found to have the most significant influence on lithology identification in a natural gas protection area in the United States, while DEN, CAL, and PEF were observed to be the most influential variables for lithology identification in the Daqing Oilfield in China. From the perspective of a single sample, the LIME algorithm can provide a quantitative prediction probability and degree of influence of the characteristic variables.
MoreTranslated text
Key words
Logging data,Lithology Identification,Ensemble Learning Stacking,Interpretability
AI Read Science
Must-Reading Tree
Example
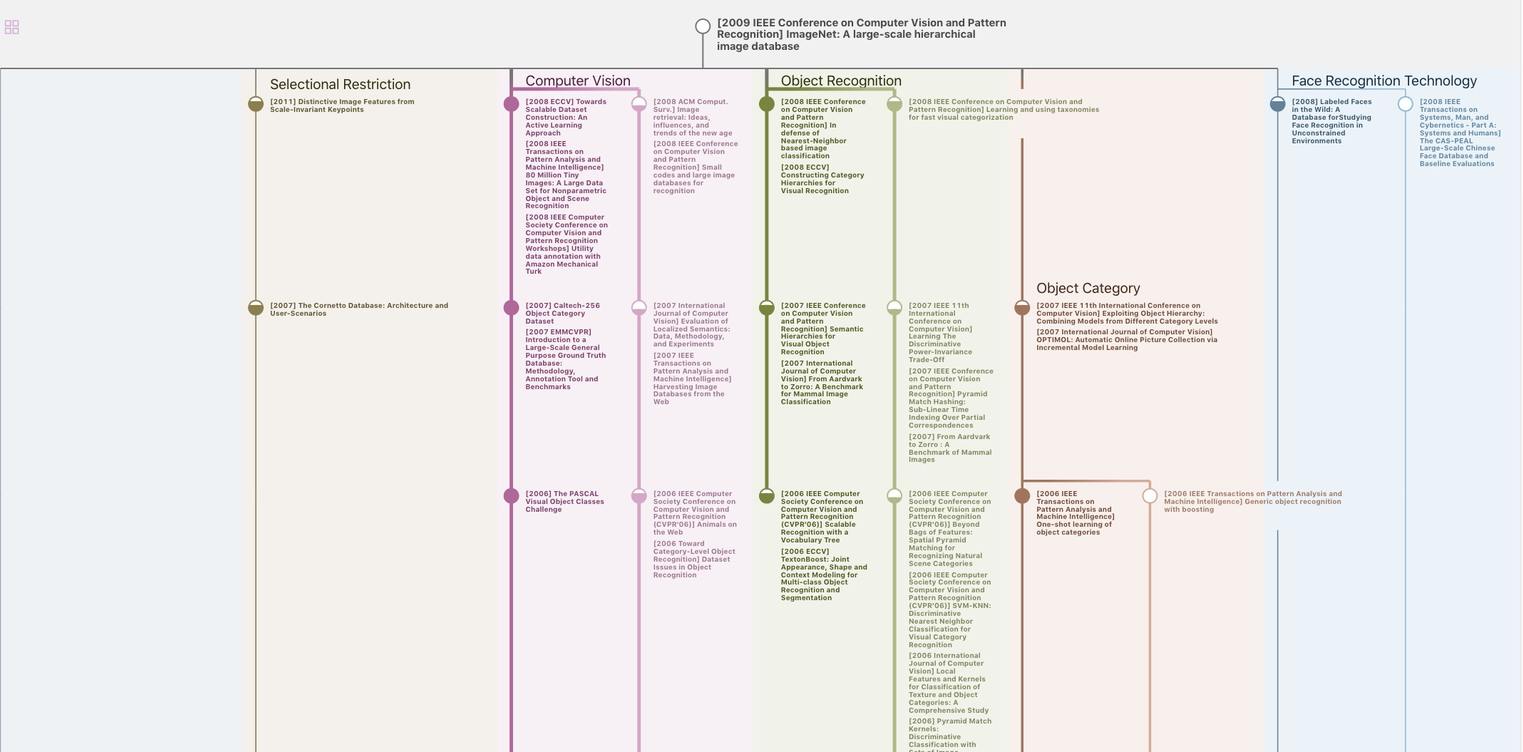
Generate MRT to find the research sequence of this paper
Chat Paper
Summary is being generated by the instructions you defined