UniQGAN: Towards Improved Modulation Classification With Adversarial Robustness Using Scalable Generator Design
IEEE Transactions on Dependable and Secure Computing(2024)
摘要
Automatic modulation classification (AMC) has been envisioned as a significant element for security issues at the physical layer due to its indispensable role in accurate communications. Recent attention to deep learning has impacted the AMC, which exhibits exceptional performance without manual feature engineering. To guarantee the accuracy and robustness of deep learning-based AMC, data augmentation is a critical issue. While existing studies have used several deep generative models to handle the data insufficiency, these studies face three challenges including low scalability, lengthy training time, and limited accuracy improvement. To this end, this paper presents UniQGAN, a novel unified generative architecture that models I/Q constellation diagrams from various signal-to-noise ratios (SNRs) using a single model. The proposed method enables the generation of high-quality data with a scalable generator, while requiring reduced training time. At the core of UniQGAN are multi-conditions embedding and multi-domains classification techniques that leverage both SNR and modulation type during the optimization process to enable unified modeling. Using abundant high-quality training data, UniQGAN accelerates the enhanced AMC with high performance and adversarial robustness. Experimental results demonstrate that the data generation by UniQGAN achieves superiority in terms of scalability, training time, and accuracy.
更多查看译文
关键词
Adversarial robustness,data augmentation,deep learning,GAN,automatic modulation classification
AI 理解论文
溯源树
样例
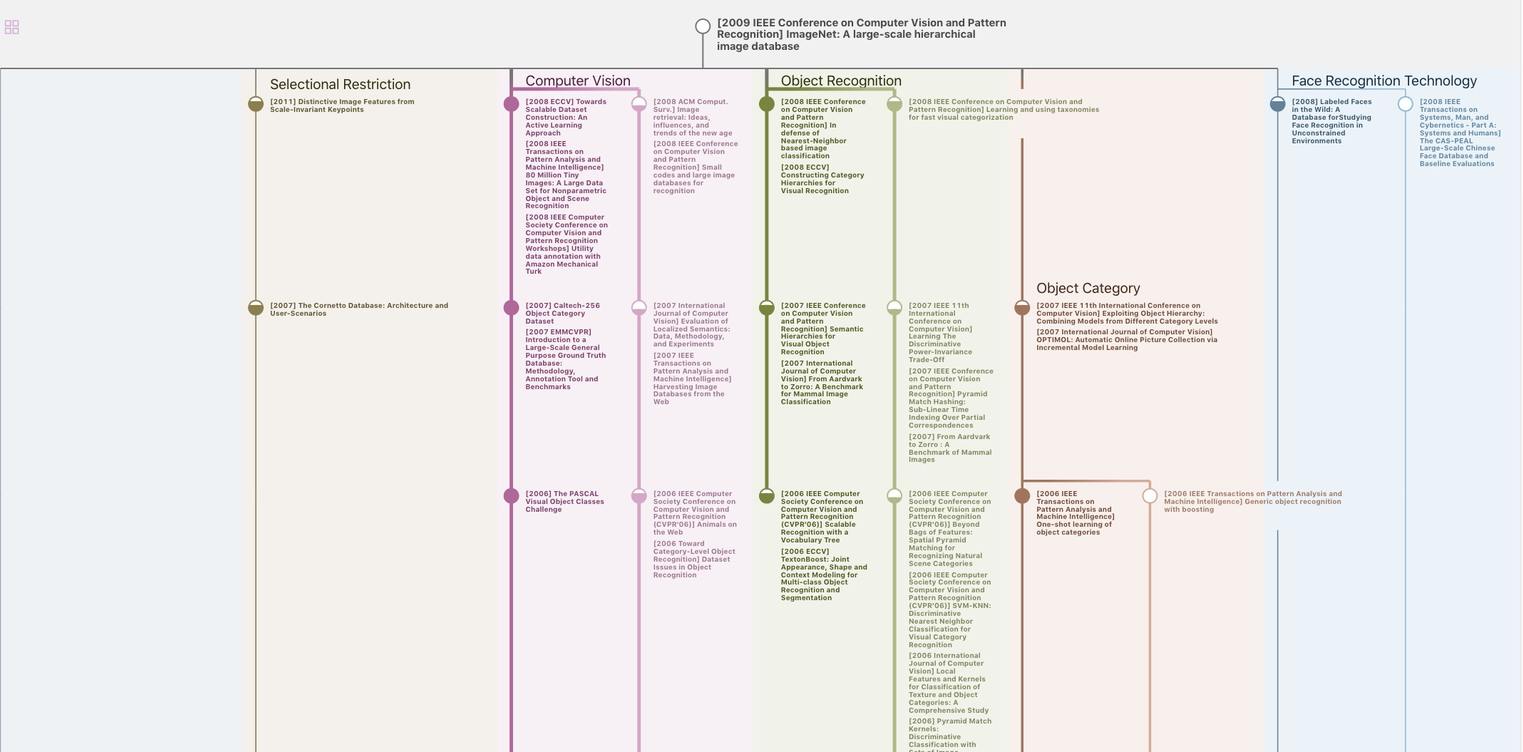
生成溯源树,研究论文发展脉络
Chat Paper
正在生成论文摘要