CAPER: Patient Preferences to Inform Non-Surgical Treatment of Chronic Low Back Pain (cLBP): A Discrete Choice Experiment
Pain Medicine(2023)
摘要
Abstract Objective We developed and used a discrete-choice measure to study patient preferences of risks and benefits of non-surgical treatments making cLBP treatment selections. Methods “CAPER TREATMENT” © was developed using standard choice-based conjoint (CBC) procedures (discrete-choice methodology that mimics individual’s decision-making). After expert input and pilot testing, our final measure had 7 attributes (chance of pain relief, duration of relief, physical activity changes, treatment method, treatment type, treatment time burden and risks of treatment) with 3–4 levels each. We created a random, full-profile, balanced-overlap experimental design using Sawtooth software. 211 respondents were recruited via emailed on-line link and completed 14 CBC choice pairs, two fixed questions, demographic, clinical, and quality-of-life questions. Analysis was random parameters multinomial logit with 1,000 Halton draws. Results Patients cared most about chance of pain relief, followed closely by improving physical activity, even more than duration of pain relief. There was comparatively less concern about time commitment and risks. Gender and socioeconomic status influenced preferences, especially with relation to strength of expectations for outcomes. Patients experiencing low pain (NRS < 4) had a stronger desire for maximally improved physical activity, while those in high pain (NRS > 6) preferred both maximum and more limited activity. Highly disabled patients (ODI > 40) demonstrated distinctly different preferences: placing more weight on achieving pain control and less on improving physical activity. Conclusions Individuals with cLBP were willing to trade risks and inconveniences for better pain control and physical activity. Additionally, different preference phenotypes exist, suggesting a need for clinicians to target treatments to particular patients.
更多查看译文
AI 理解论文
溯源树
样例
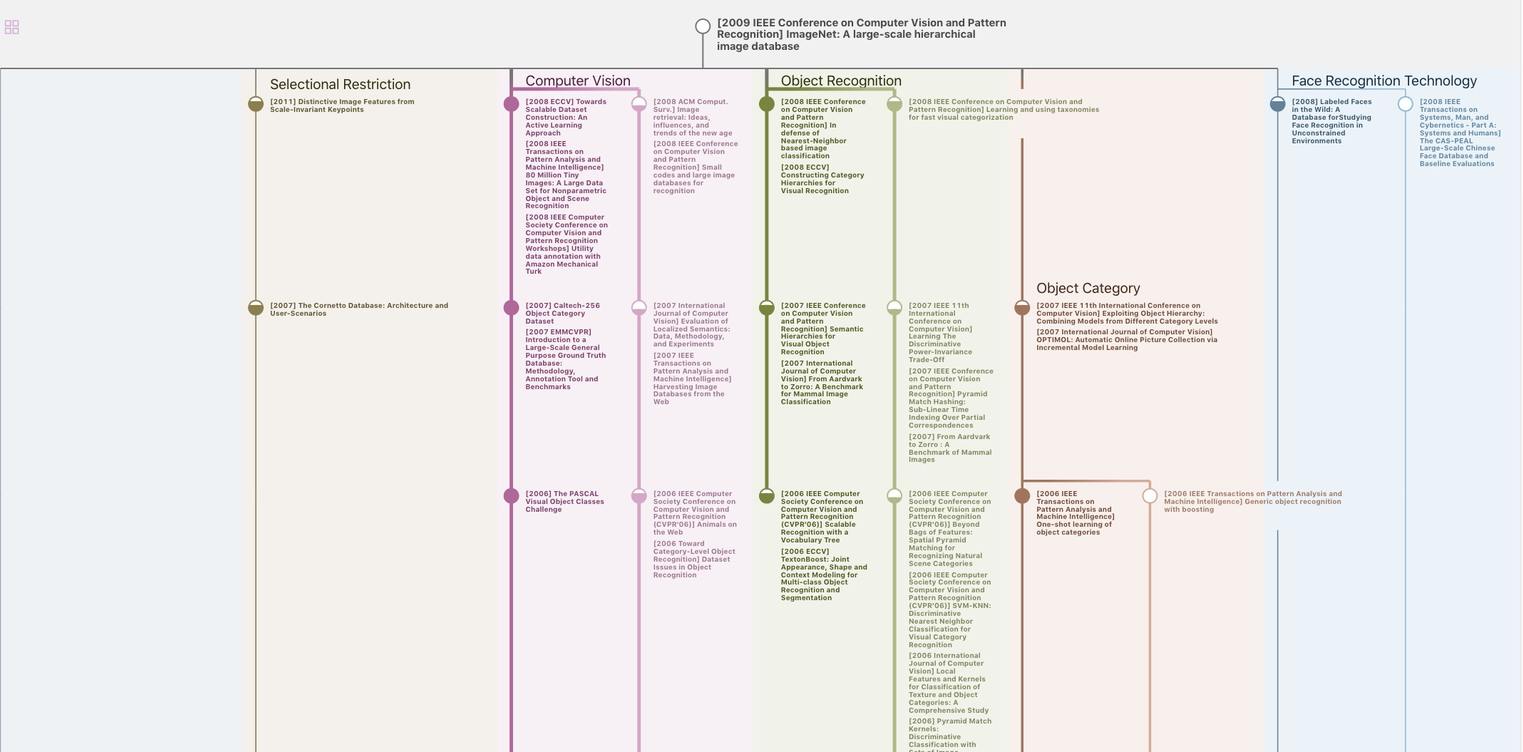
生成溯源树,研究论文发展脉络
Chat Paper
正在生成论文摘要