Language models can identify enzymatic active sites in protein sequences
crossref(2023)
摘要
Recent advances in language modeling have tremendously impacted how we handle sequential data in science. Language architectures have emerged as a hotbed of innovation and creativity in natural language processing over the last decade, and have since gained prominence in modeling proteins and chemical processes, elucidating structural relationships from textual/sequential data. Surprisingly, some of these relationships refer to three-dimensional structural features, raising important questions on the dimensionality of the information contained in sequential data. We demonstrate that the unsupervised use of a language model architecture to a language representation of bio-catalyzed chemical reactions can capture the signal at the base of the substrate-active site atomic interactions, identifying the three- dimensional active site position in unknown protein sequences. The language representation comprises a reaction-simplified molecular-input line-entry system (SMILES) for substrate and products, and amino acid sequence information for the enzyme. This approach can recover, with no supervision, 52.12% of the active site when considering co-crystallized substrate-enzyme structures as ground truth, vastly outperforming other attention-based models.
更多查看译文
AI 理解论文
溯源树
样例
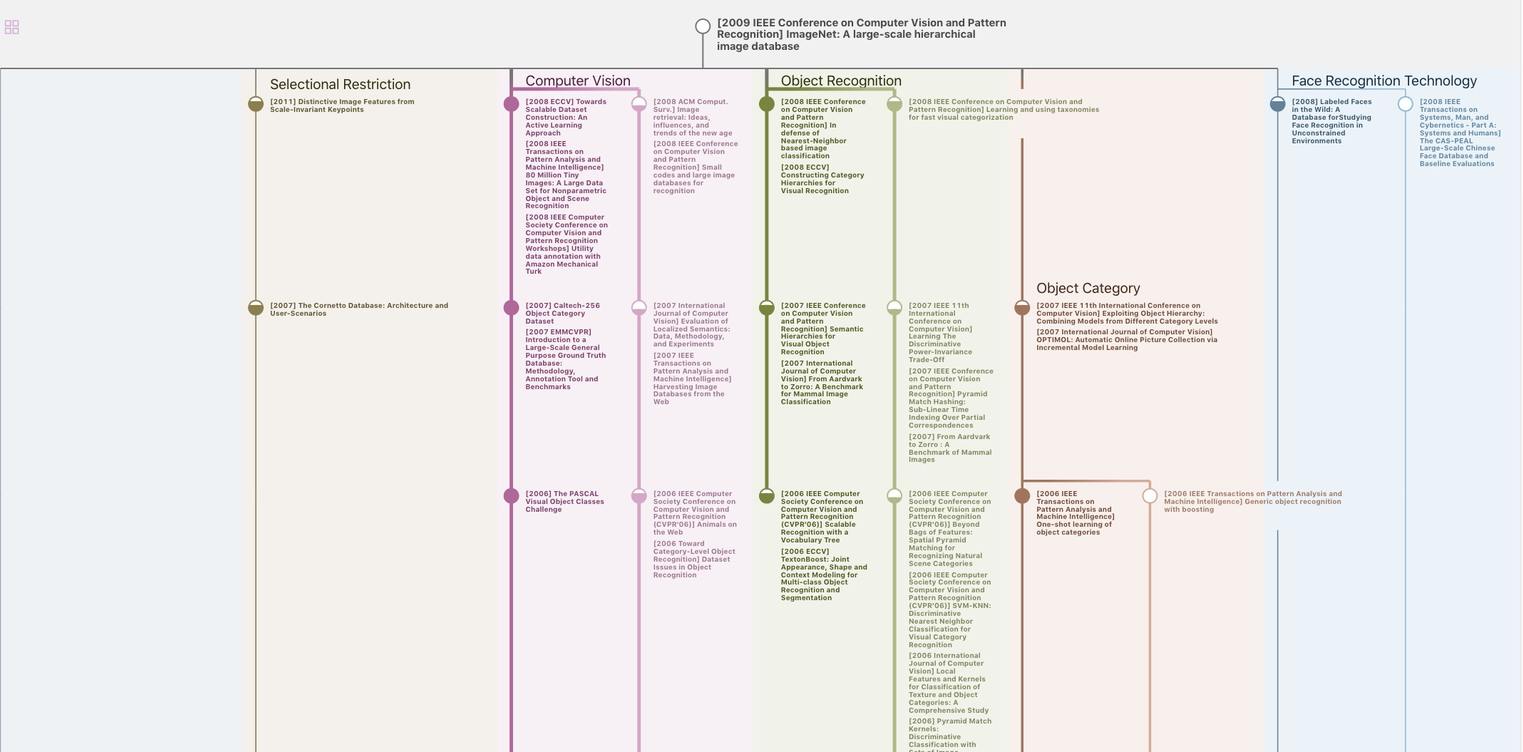
生成溯源树,研究论文发展脉络
Chat Paper
正在生成论文摘要