Road Planning for Slums via Deep Reinforcement Learning
arXiv (Cornell University)(2023)
摘要
Millions of slum dwellers suffer from poor accessibility to urban services due to inadequate road infrastructure within slums, and road planning for slums is critical to the sustainable development of cities. Existing re-blocking or heuristic methods are either time-consuming which cannot generalize to different slums, or yield sub-optimal road plans in terms of accessibility and construction costs. In this paper, we present a deep reinforcement learning based approach to automatically layout roads for slums. We propose a generic graph model to capture the topological structure of a slum, and devise a novel graph neural network to select locations for the planned roads. Through masked policy optimization, our model can generate road plans that connect places in a slum at minimal construction costs. Extensive experiments on real-world slums in different countries verify the effectiveness of our model, which can significantly improve accessibility by 14.3% against existing baseline methods. Further investigations on transferring across different tasks demonstrate that our model can master road planning skills in simple scenarios and adapt them to much more complicated ones, indicating the potential of applying our model in real-world slum upgrading.
更多查看译文
关键词
road planning,slums,reinforcement learning
AI 理解论文
溯源树
样例
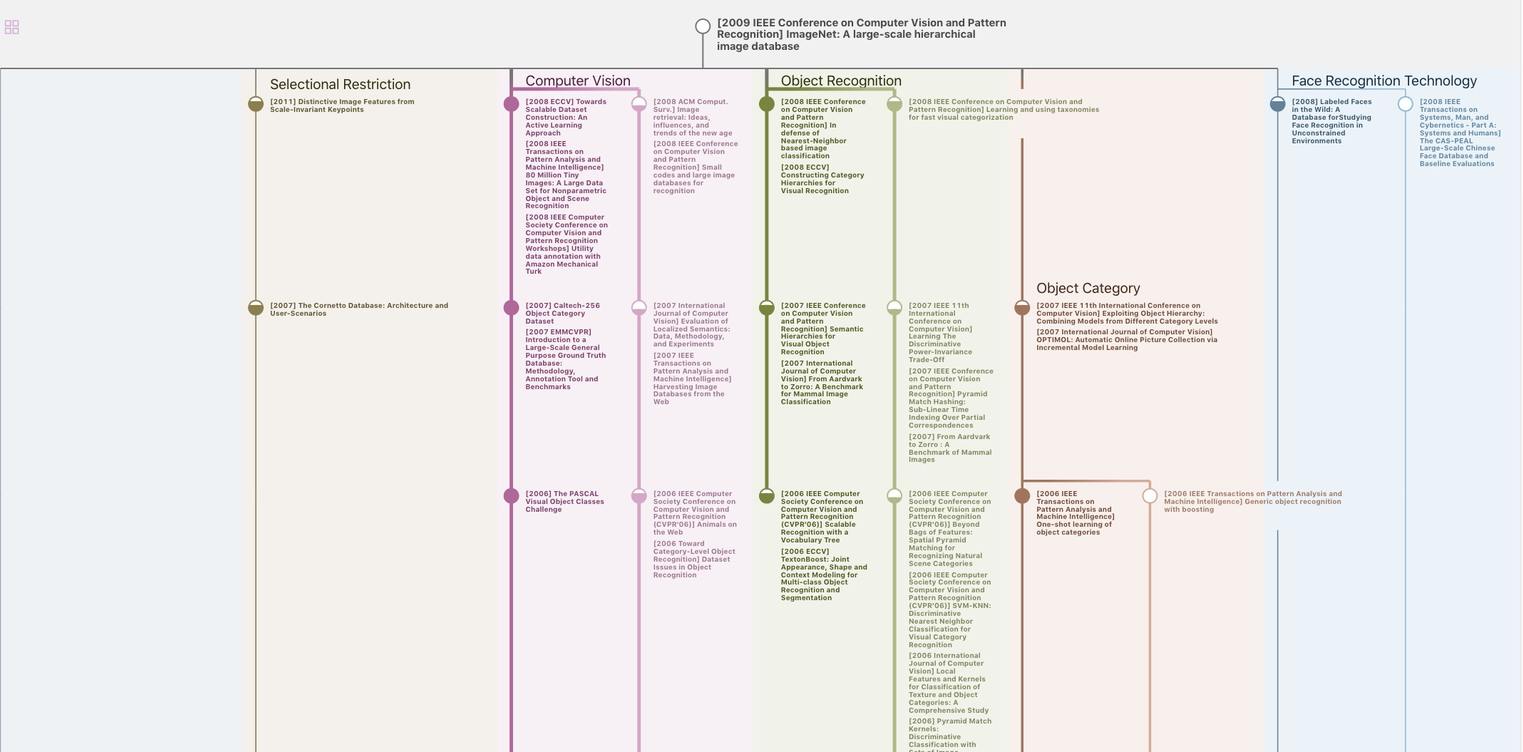
生成溯源树,研究论文发展脉络
Chat Paper
正在生成论文摘要