EXACT: Extensive Attack for Split Learning
CoRR(2023)
摘要
Privacy-Preserving machine learning (PPML) can help us train and deploy models that utilize private information. In particular, on-device Machine Learning allows us to completely avoid sharing information with a third-party server during inference. However, on-device models are typically less accurate when compared to the server counterparts due to the fact that (1) they typically only rely on a small set of on-device features and (2) they need to be small enough to run efficiently on end-user devices. Split Learning (SL) is a promising approach that can overcome these limitations. In SL, a large machine learning model is divided into two parts, with the bigger part residing on the server-side and a smaller part executing on-device, aiming to incorporate the private features. However, end-to-end training of such models requires exchanging gradients at the cut layer, which might encode private features or labels. In this paper, we provide insights into potential privacy risks associated with SL and introduce a novel attack method, EXACT, to reconstruct private information. Furthermore, we also investigate the effectiveness of various mitigation strategies. Our results indicate that the gradients significantly improve the attacker's effectiveness in all three datasets reaching almost 100% reconstruction accuracy for some features. However, a small amount of differential privacy (DP) is quite effective in mitigating this risk without causing significant training degradation.
更多查看译文
关键词
split learning,extensive attack
AI 理解论文
溯源树
样例
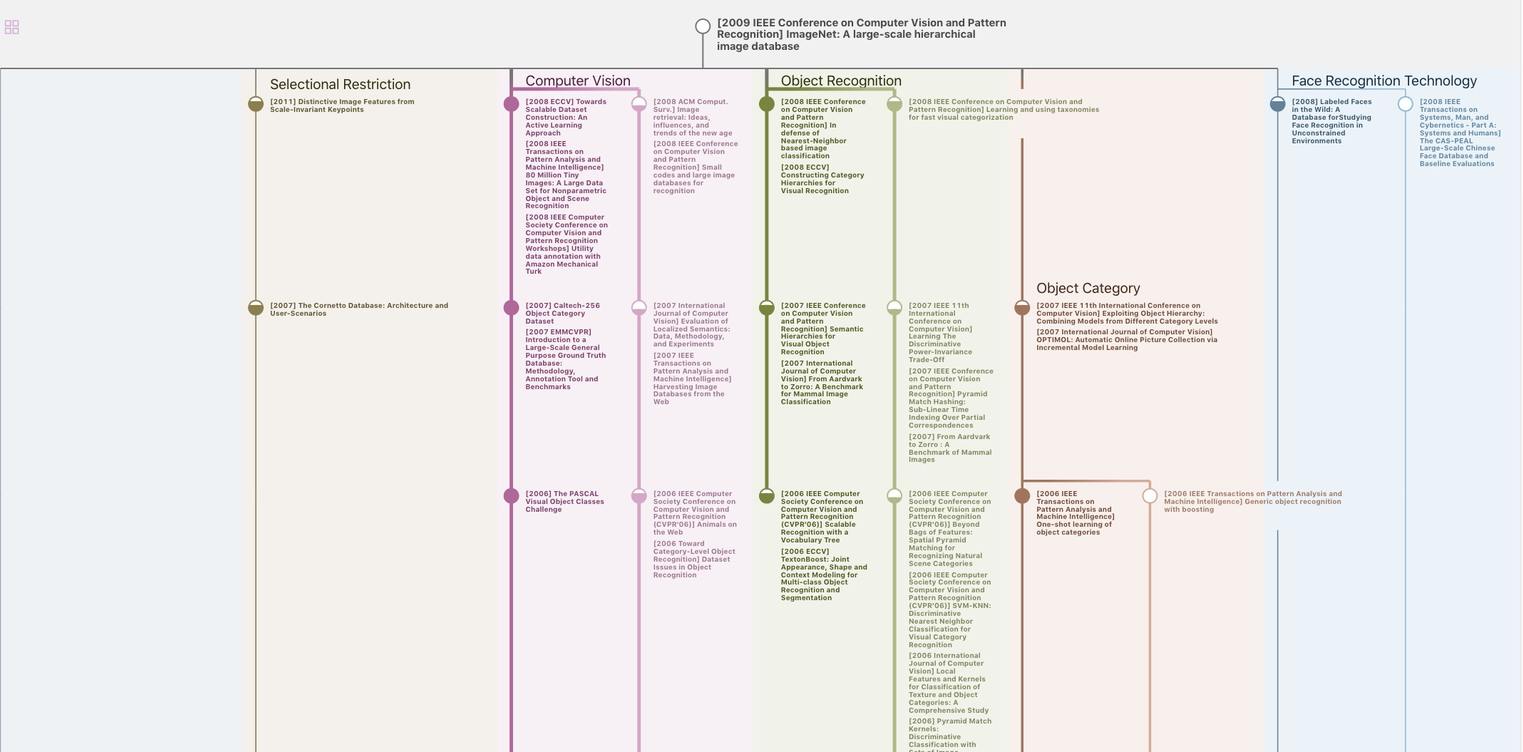
生成溯源树,研究论文发展脉络
Chat Paper
正在生成论文摘要