Feasibility of Transfer Learning: A Mathematical Framework
CoRR(2023)
Abstract
Transfer learning is a popular paradigm for utilizing existing knowledge from previous learning tasks to improve the performance of new ones. It has enjoyed numerous empirical successes and inspired a growing number of theoretical studies. This paper addresses the feasibility issue of transfer learning. It begins by establishing the necessary mathematical concepts and constructing a mathematical framework for transfer learning. It then identifies and formulates the three-step transfer learning procedure as an optimization problem, allowing for the resolution of the feasibility issue. Importantly, it demonstrates that under certain technical conditions, such as appropriate choice of loss functions and data sets, an optimal procedure for transfer learning exists. This study of the feasibility issue brings additional insights into various transfer learning problems. It sheds light on the impact of feature augmentation on model performance, explores potential extensions of domain adaptation, and examines the feasibility of efficient feature extractor transfer in image classification.
MoreTranslated text
Key words
transfer learning,mathematical framework,feasibility
AI Read Science
Must-Reading Tree
Example
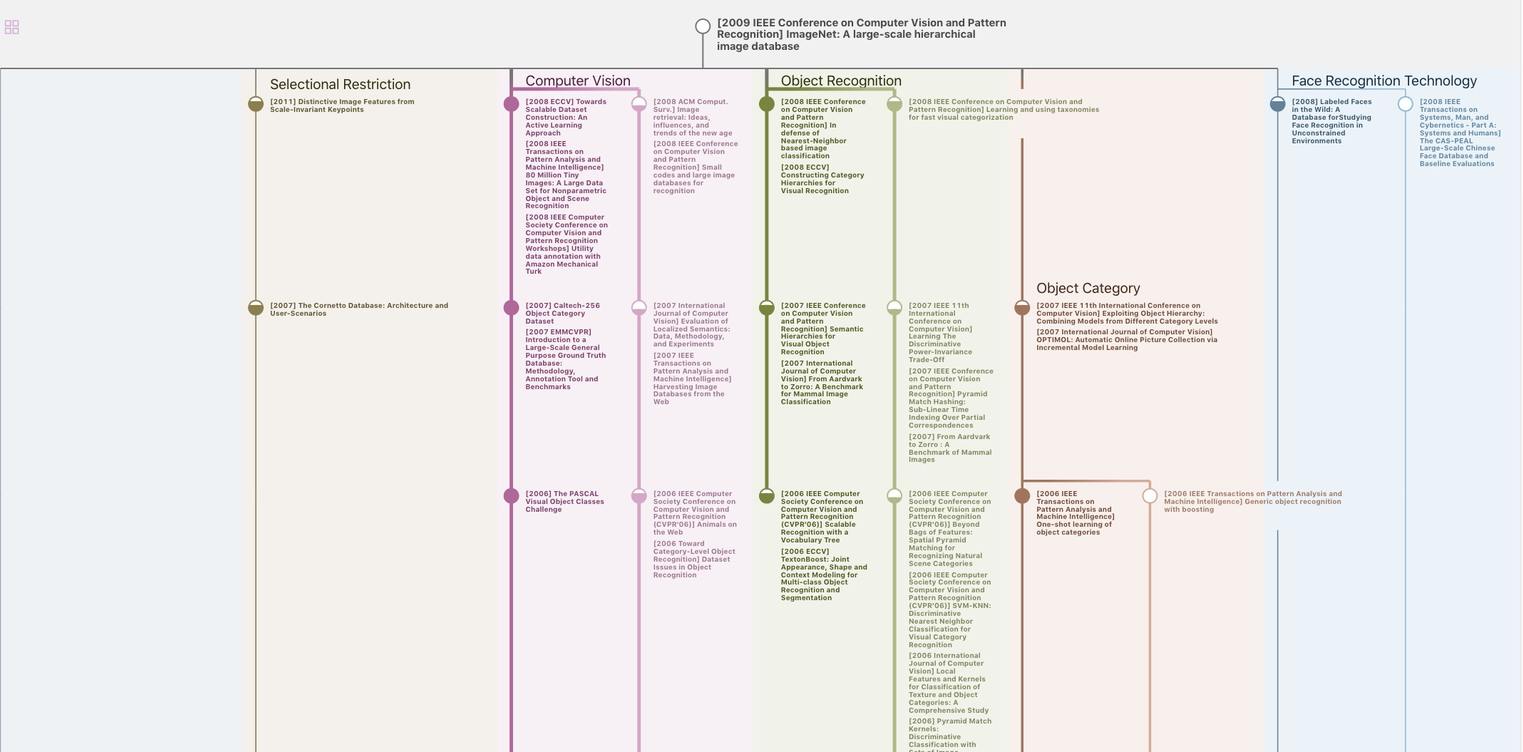
Generate MRT to find the research sequence of this paper
Chat Paper
Summary is being generated by the instructions you defined