On the Efficacy and Noise-Robustness of Jointly Learned Speech Emotion and Automatic Speech Recognition
CoRR(2023)
摘要
New-age conversational agent systems perform both speech emotion recognition (SER) and automatic speech recognition (ASR) using two separate and often independent approaches for real-world application in noisy environments. In this paper, we investigate a joint ASR-SER multitask learning approach in a low-resource setting and show that improvements are observed not only in SER, but also in ASR. We also investigate the robustness of such jointly trained models to the presence of background noise, babble, and music. Experimental results on the IEMOCAP dataset show that joint learning can improve ASR word error rate (WER) and SER classification accuracy by 10.7% and 2.3% respectively in clean scenarios. In noisy scenarios, results on data augmented with MUSAN show that the joint approach outperforms the independent ASR and SER approaches across many noisy conditions. Overall, the joint ASR-SER approach yielded more noise-resistant models than the independent ASR and SER approaches.
更多查看译文
关键词
learned speech emotion
AI 理解论文
溯源树
样例
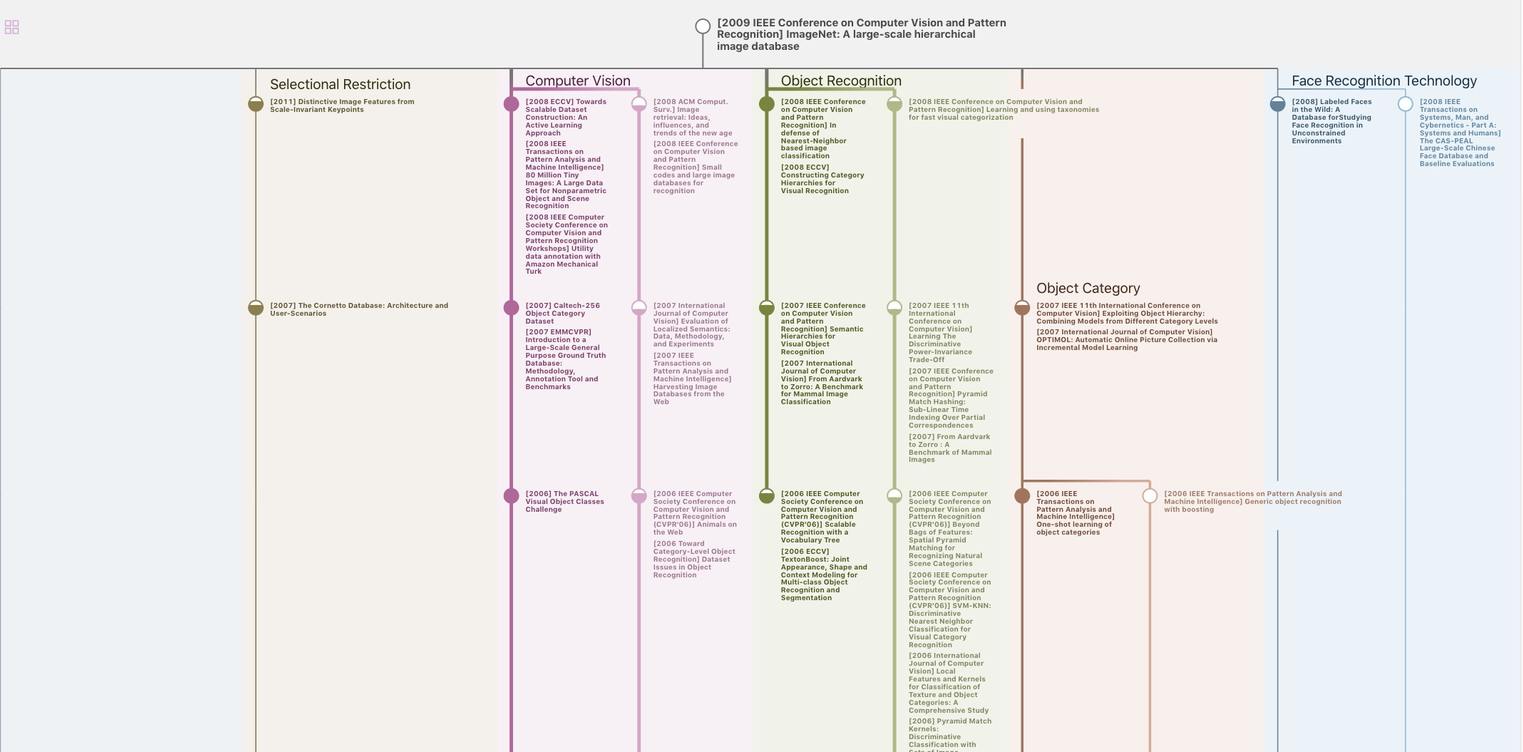
生成溯源树,研究论文发展脉络
Chat Paper
正在生成论文摘要