Mitigating Catastrophic Forgetting in Task-Incremental Continual Learning with Adaptive Classification Criterion
CoRR(2023)
摘要
Task-incremental continual learning refers to continually training a model in a sequence of tasks while overcoming the problem of catastrophic forgetting (CF). The issue arrives for the reason that the learned representations are forgotten for learning new tasks, and the decision boundary is destructed. Previous studies mostly consider how to recover the representations of learned tasks. It is seldom considered to adapt the decision boundary for new representations and in this paper we propose a Supervised Contrastive learning framework with adaptive classification criterion for Continual Learning (SCCL), In our method, a contrastive loss is used to directly learn representations for different tasks and a limited number of data samples are saved as the classification criterion. During inference, the saved data samples are fed into the current model to obtain updated representations, and a k Nearest Neighbour module is used for classification. In this way, the extensible model can solve the learned tasks with adaptive criteria of saved samples. To mitigate CF, we further use an instance-wise relation distillation regularization term and a memory replay module to maintain the information of previous tasks. Experiments show that SCCL achieves state-of-the-art performance and has a stronger ability to overcome CF compared with the classification baselines.
更多查看译文
关键词
catastrophic forgetting,learning,classification,task-incremental
AI 理解论文
溯源树
样例
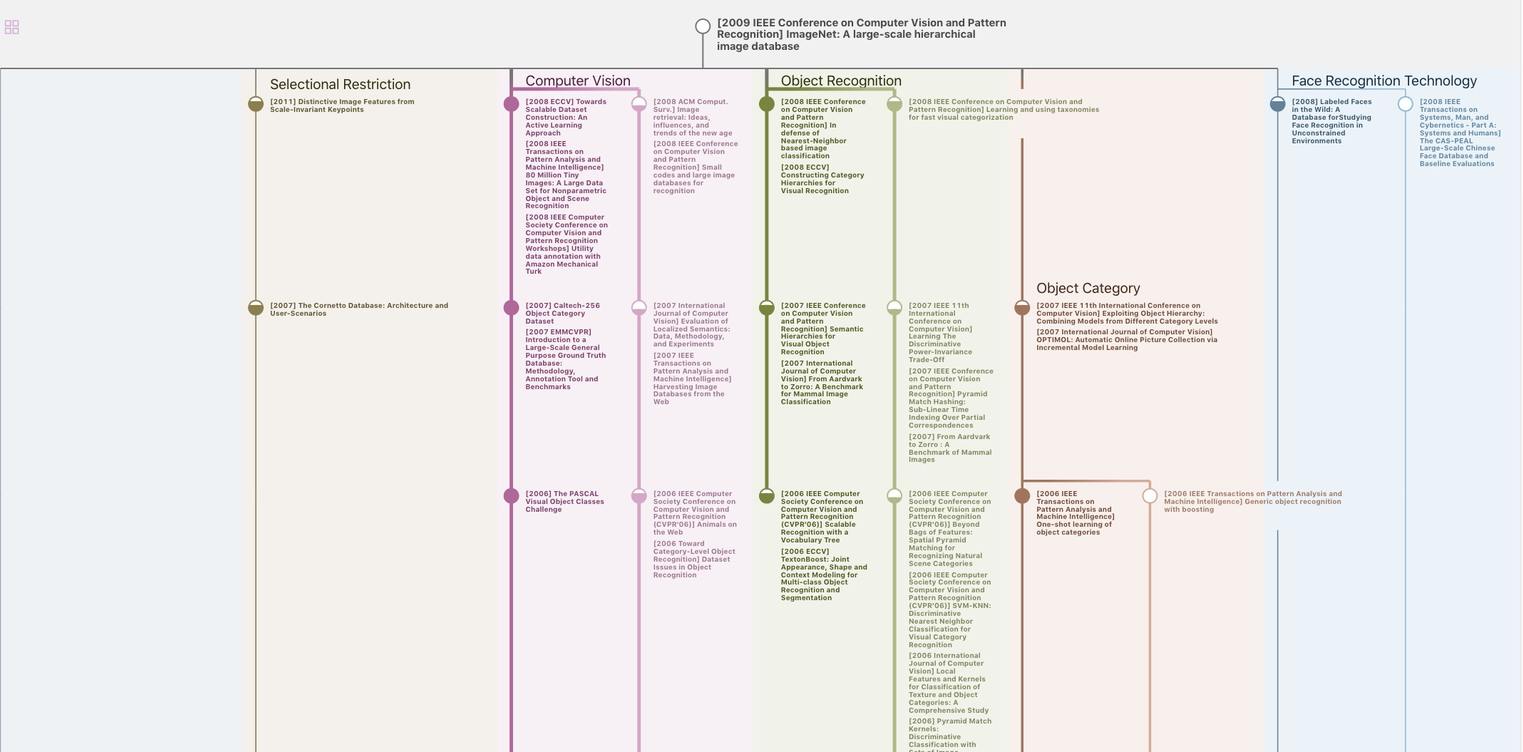
生成溯源树,研究论文发展脉络
Chat Paper
正在生成论文摘要