GFDC: A granule fusion density-based clustering with evidential reasoning
INTERNATIONAL JOURNAL OF APPROXIMATE REASONING(2024)
摘要
Density-based clustering algorithms are known for their ability to detect irregular clusters, but they have limitations when it comes to dealing with clusters of varying densities. In this paper, we propose a new clustering algorithm called granule fusion density-based clustering with evidential reasoning (GFDC). The approach introduces the concept of sparse degree, which measures both the local density and global density of samples. The sparse degree of samples reflects the stability of samples. Moreover, a core-granule is composed of the neighborhood granule of a sample, of which the sparse degree is minimum in its neighborhood. Then, the core-granules are generated based on the sparse degree and are insensitive to clusters with varying densities. The core samples, which consist of samples in core-granules, are used to form initial clusters through fusion strategies. Additionally, an assignment method is developed from Dempster-Shafer theory to assign border samples and identify outliers. The experimental results demonstrate the effectiveness of GFDC on extensive synthetic and real-world datasets.
更多查看译文
关键词
Granular computing,Granule fusion,Density-based clustering,Dempster-Shafer theory
AI 理解论文
溯源树
样例
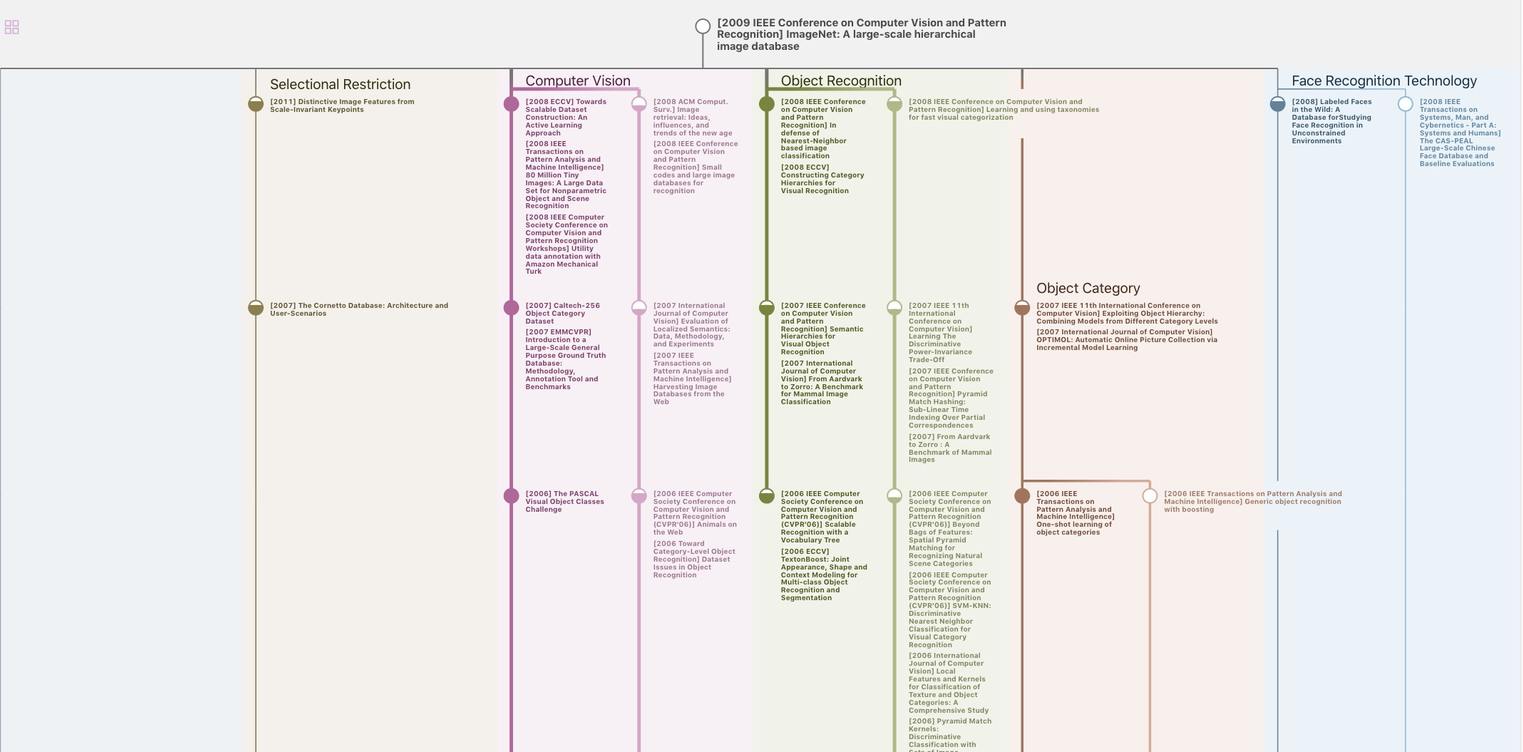
生成溯源树,研究论文发展脉络
Chat Paper
正在生成论文摘要