Fast equilibrium reconstruction by deep learning on EAST tokamak
AIP ADVANCES(2023)
摘要
A deep neural network is developed and trained on magnetic measurements (input) and EFIT poloidal magnetic flux (output) on the EAST tokamak. In optimizing the network architecture, we use automatic optimization in searching for the best hyperparameters, which helps the model generalize better. We compare the inner magnetic surfaces and last-closed-flux surfaces (LCFSs) with those from EFIT. We also calculated the normalized internal inductance, which is completely determined by the poloidal magnetic flux and can further reflect the accuracy of the prediction. The time evolution of the internal inductance in full discharges is compared with that provided by EFIT. All of the comparisons show good agreement, demonstrating the accuracy of the machine learning model, which has the high spatial resolution as the off-line EFIT while still meets the time constraint of real-time control.
更多查看译文
AI 理解论文
溯源树
样例
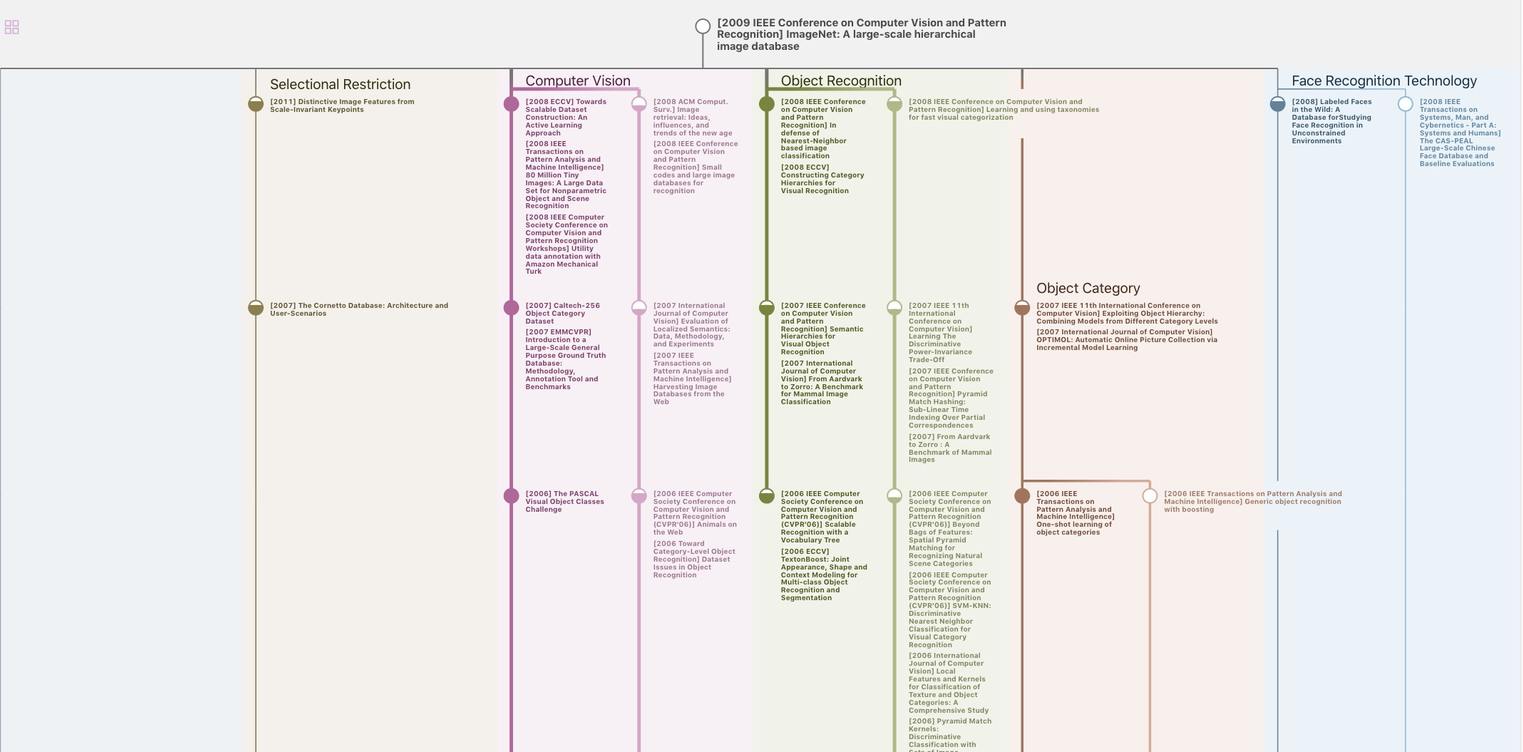
生成溯源树,研究论文发展脉络
Chat Paper
正在生成论文摘要