Novel deep learning methods for 3D flow field segmentation and classification
Expert Systems with Applications(2024)
Abstract
Flow field segmentation and classification help researchers to understand vortex structure and thus turbulent flow. Existing deep learning methods are mainly based on global vorticity information and focused on 2D circumstance. In this paper, based on flow field theory, criteria for flow field segmentation and classification in 3D space using local velocity information and the physical relationship between local vorticity and vortex wake are conducted, and then novel physical local information-embedded flow field segmentation and classification methods in 3D space using Multilayer Perceptron (MLP) are proposed. Our methods consist of three modules: a preprocessing module, a flow field segmentation module and a flow field classification module. The preprocessing module calculates input for succeeding modules based on the above criteria. The segmentation and classification modules process corresponding input to identify vortex structure, and further classify the type of vortex wakes in a 3D flow field. Experimental results show that our method can identify vortex area with an accuracy rate that reaches 90% while reducing time consumption by more than 90% compared with existing segmentation methods; our classification method reaches an accuracy rate of 99.7% while reducing time consumption by more than 90% compared with existing classification methods. Our proposed methods proved to achieve good performance both on accuracy and efficiency.
MoreTranslated text
Key words
Three-dimensional space,Deep learning,Flow field segmentation,Flow field classification
AI Read Science
Must-Reading Tree
Example
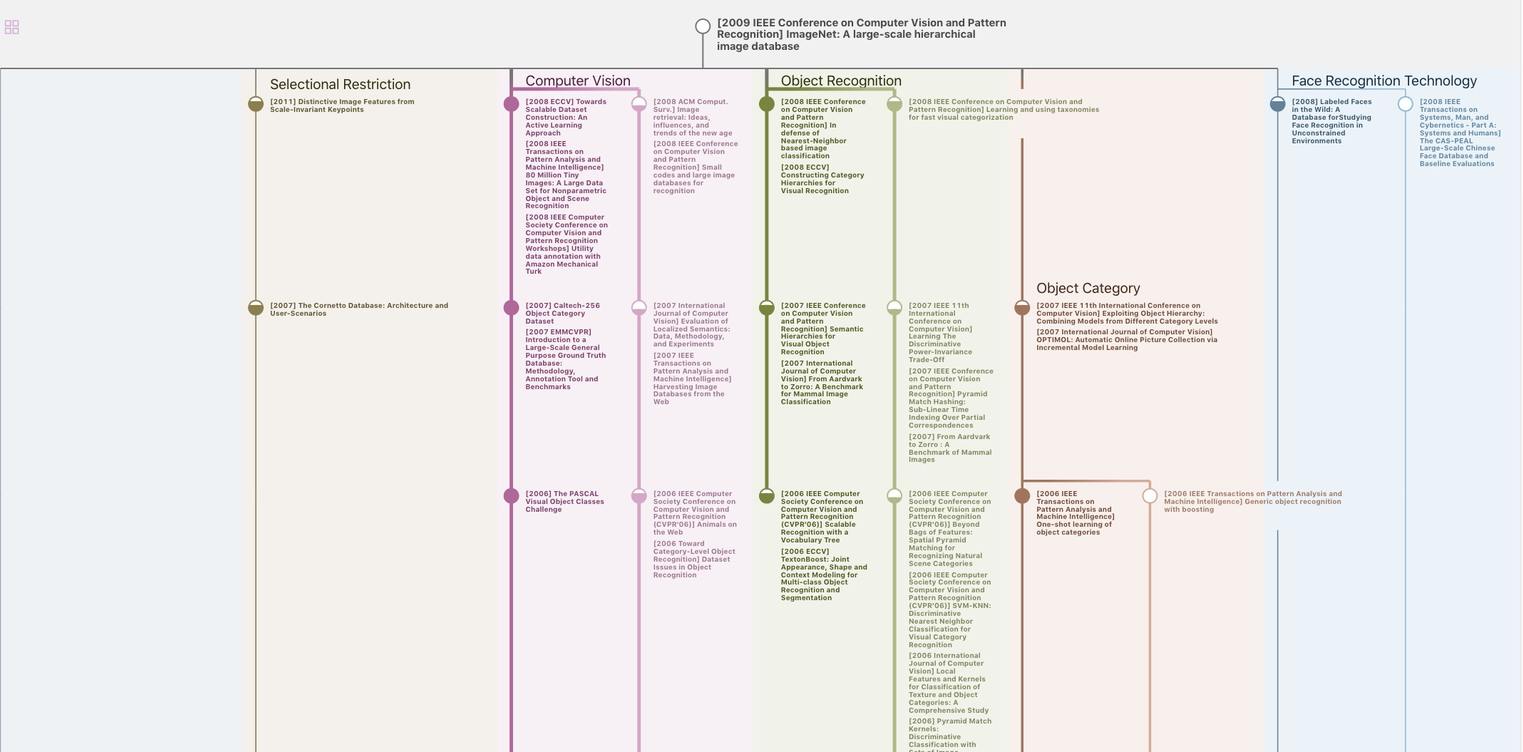
Generate MRT to find the research sequence of this paper
Chat Paper
Summary is being generated by the instructions you defined