LFWE: L inguistic F eature Based W ord E mbedding for Hindi Fake News Detection
ACM Transactions on Asian and Low-Resource Language Information Processing(2023)
Abstract
It is essential for the research communities to investigate ways for authenticating news. The use of linguistic feature-based analysis to automatically detect false news is gaining popularity among the scientific community. However, such techniques are exclusively created for English, leaving low-resource languages, like Hindi behind. To address this issue, we constructed a novel annotated Hindi Fake News (HinFakeNews) dataset of roughly 33,300 articles that can be utilized to develop autonomous fake news detection systems. This work provides a two-stage benchmark model for identifying fake news in Hindi using machine learning. The proposed model, Linguistic Feature Based Word Embedding (LFWE) generates Word Embedding (WE) over linguistic features. This paper focuses on 24 key linguistic features (14 extracted and 10 derived) for successful detection of Hindi fake news. These features are grouped as lexical, semantic, syntactic, psycholinguistic, readability, and quantity features. The contribution is two-fold: In the first phase, the dataset is pre-processed and linguistic features are extracted. In the second phase, Feature Sets (F-Sets) are generated as WE, and an Ensemble voting classification is carried out on the F-Sets. According to experimental findings, the LFWE model accurately detects and classifies fake news in Hindi with an accuracy of 98.49%.
MoreTranslated text
AI Read Science
Must-Reading Tree
Example
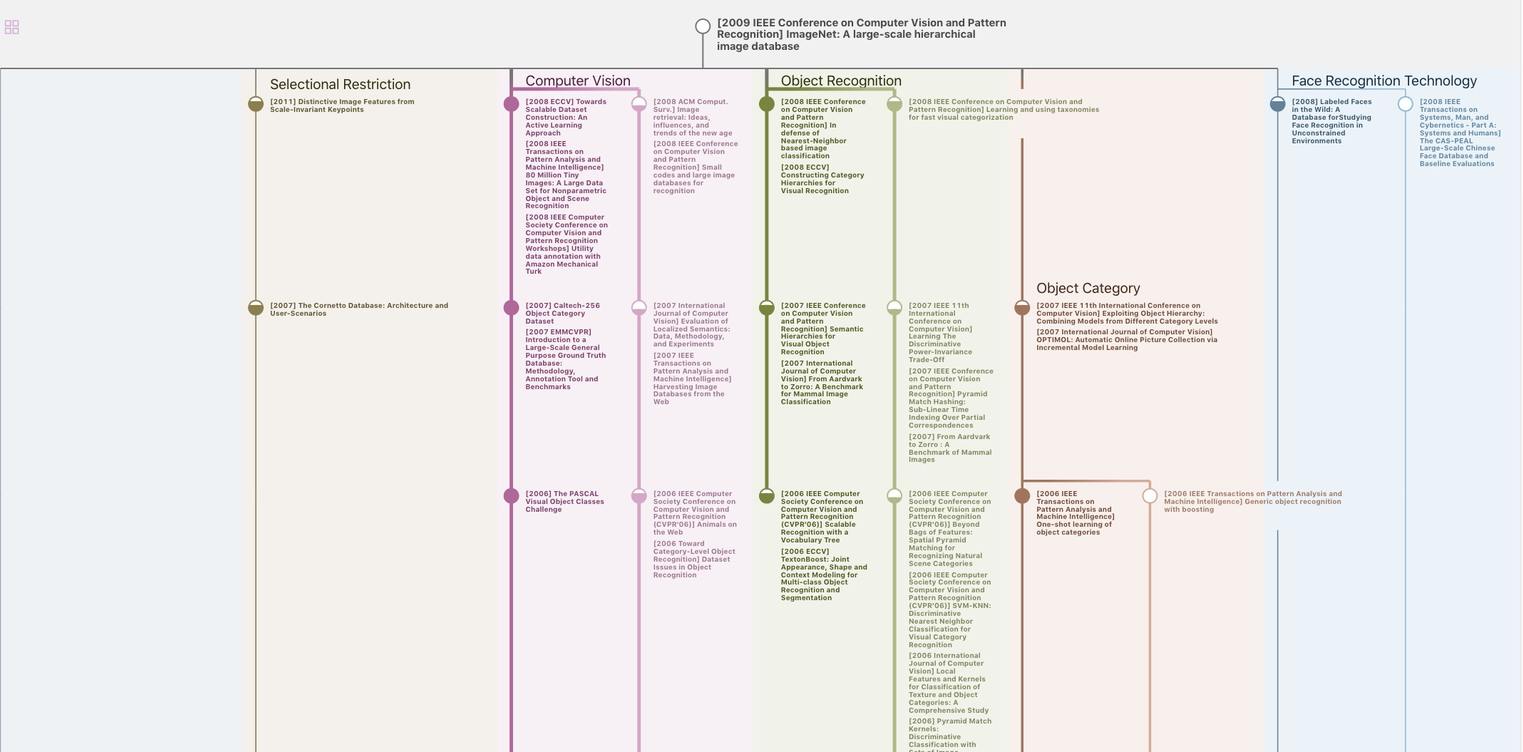
Generate MRT to find the research sequence of this paper
Chat Paper
Summary is being generated by the instructions you defined