COCOs Nucifera Tree Disease Prediction Using Faster Region-Convolutional Neural Network with ResNet-50
2024 Third International Conference on Distributed Computing and Electrical Circuits and Electronics (ICDCECE)(2024)
Abstract
Coconut diseases were predicted based on various categories such as color, shape, time frame, and changes in growth. Deep learning (DL) was applied for this purpose. However, the image dataset used was unlabelled, leading to challenges such as overfitting and complexity in computational time for prediction. The proposed method is the Faster Region-Convolutional Neural Network (R-CNN) model for detecting and classifying coconut clusters, aiming to minimize overfitting in the identification of coconut nucifera diseases. The coconut disease dataset involved performing image classification, distinguishing between healthy and unhealthy coconuts. Normalization, feature extraction, and classification techniques were utilized for this task. For feature extraction, the VGG-16 model was involved, which includes maximum pooling layers to extract features at different levels of the network enhancing the quality of feature maps and providing better region identification. Classification was performed using the R-CNN with ResNet-50. The dataset contained images of both healthy and unhealthy coconuts, and the regions were predicted to identify and classify the different diseases within the images. ResNet-50 captures high-level features and helps mitigate the vanishing gradient problem during training, making it easier to connect with the R-CNN method. The proposed Faster R-CNN with ResNet-50 achieved a performance accuracy of 98.40%. This performance was compared to existing methods such as Convolutional Neural Network (CNN), Deep-CNN (DCNN) and Long Short-Term Memory (LSTM).
MoreTranslated text
Key words
coconut disease prediction,convolutional neural network,faster region-convolutional neural network,long short-term memory
AI Read Science
Must-Reading Tree
Example
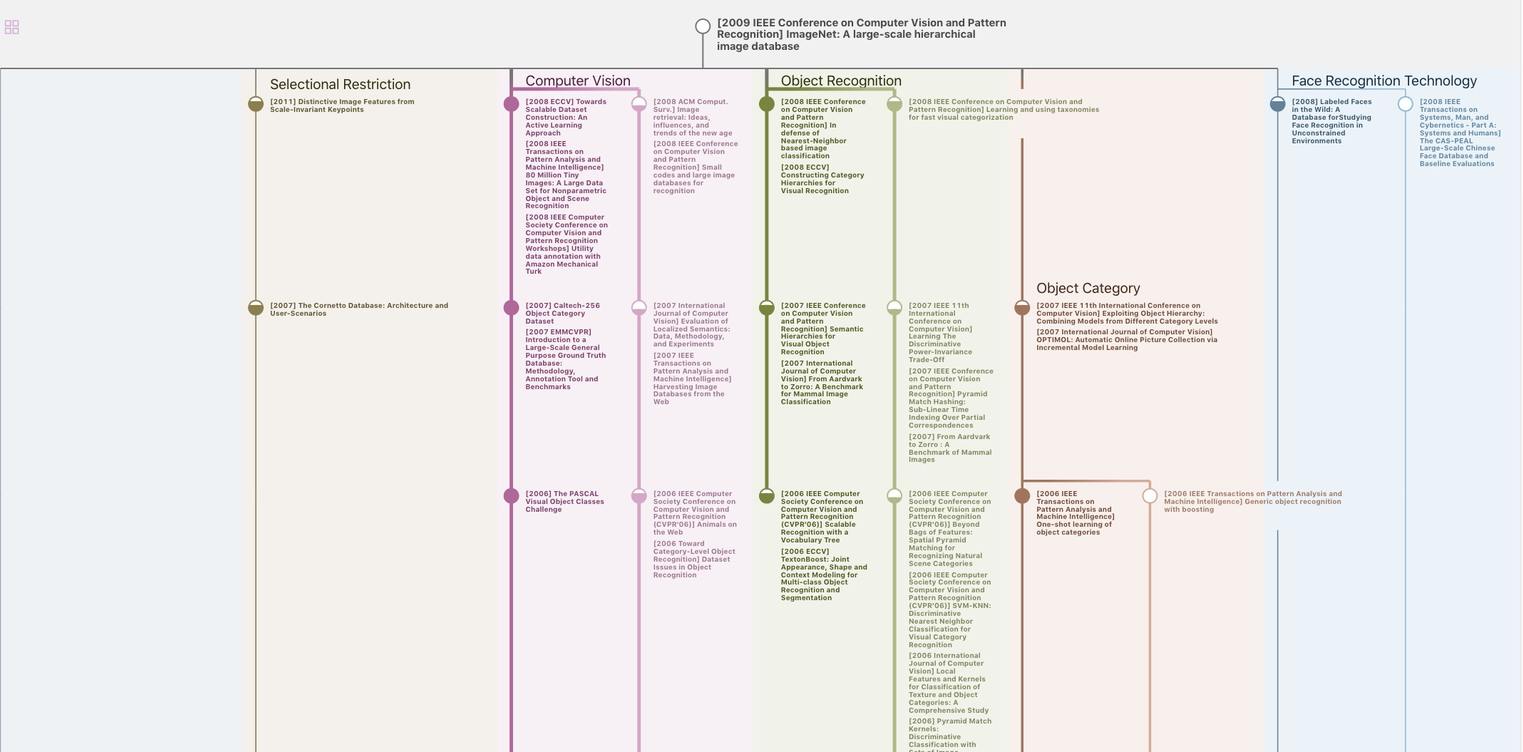
Generate MRT to find the research sequence of this paper
Chat Paper
Summary is being generated by the instructions you defined