An Efficient EEG Signal Analysis for Emotion Recognition Using FPGA
Information(2024)
摘要
Electroencephalography (EEG), electromyography (EMG), galvanic skin response (GSR), and electrocardiogram (ECG) are among the techniques developed for collecting psychophysiological data from humans. This study presents a feature extraction technique for identifying emotions in EEG-based data from the human brain. Independent component analysis (ICA) was employed to eliminate artifacts from the raw brain signals before applying signal extraction to a convolutional neural network (CNN) for emotion identification. These features were then learned by the proposed CNN-LSTM (long short-term memory) algorithm, which includes a ResNet-152 classifier. The CNN-LSTM with ResNet-152 algorithm was used for the accurate detection and analysis of human emotional data. The SEED V dataset was employed for data collection in this study, and the implementation was carried out using an Altera DE2 FPGA development board, demonstrating improved performance in terms of FPGA speed and area optimization.
更多查看译文
关键词
FPGA implementation,signal processing,emotion recognition,independent component analysis,neural networks
AI 理解论文
溯源树
样例
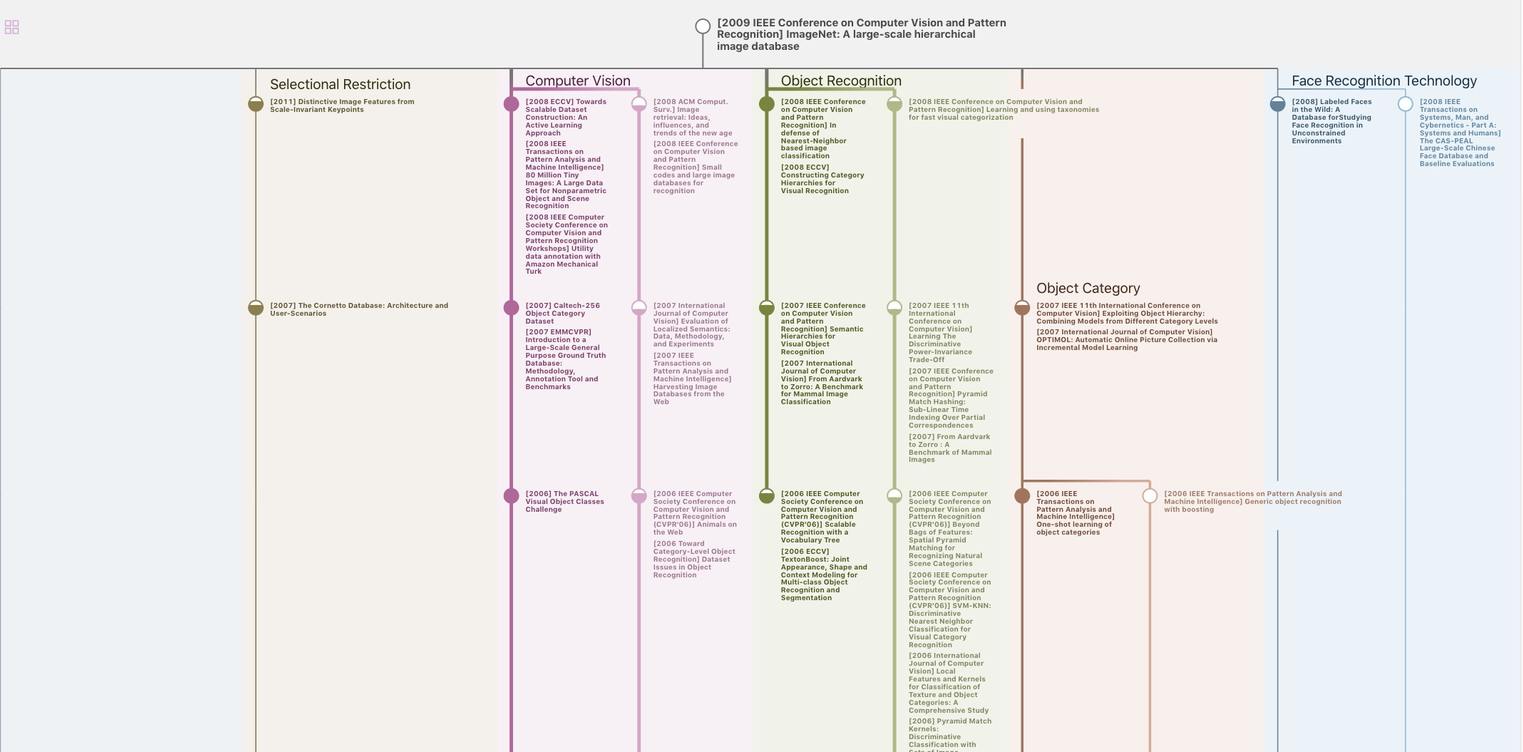
生成溯源树,研究论文发展脉络
Chat Paper
正在生成论文摘要