Unsupervised Scientific Abstract Segmentation with Normalized Mutual Information
CoRR(2023)
摘要
The abstracts of scientific papers consist of premises and conclusions. Structured abstracts explicitly highlight the conclusion sentences, whereas non-structured abstracts may have conclusion sentences at uncertain positions. This implicit nature of conclusion positions makes the automatic segmentation of scientific abstracts into premises and conclusions a challenging task. In this work, we empirically explore using Normalized Mutual Information (NMI) for abstract segmentation. We consider each abstract as a recurrent cycle of sentences and place segmentation boundaries by greedily optimizing the NMI score between premises and conclusions. On non-structured abstracts, our proposed unsupervised approach GreedyCAS achieves the best performance across all evaluation metrics; on structured abstracts, GreedyCAS outperforms all baseline methods measured by $P_k$. The strong correlation of NMI to our evaluation metrics reveals the effectiveness of NMI for abstract segmentation.
更多查看译文
关键词
normalized mutual
AI 理解论文
溯源树
样例
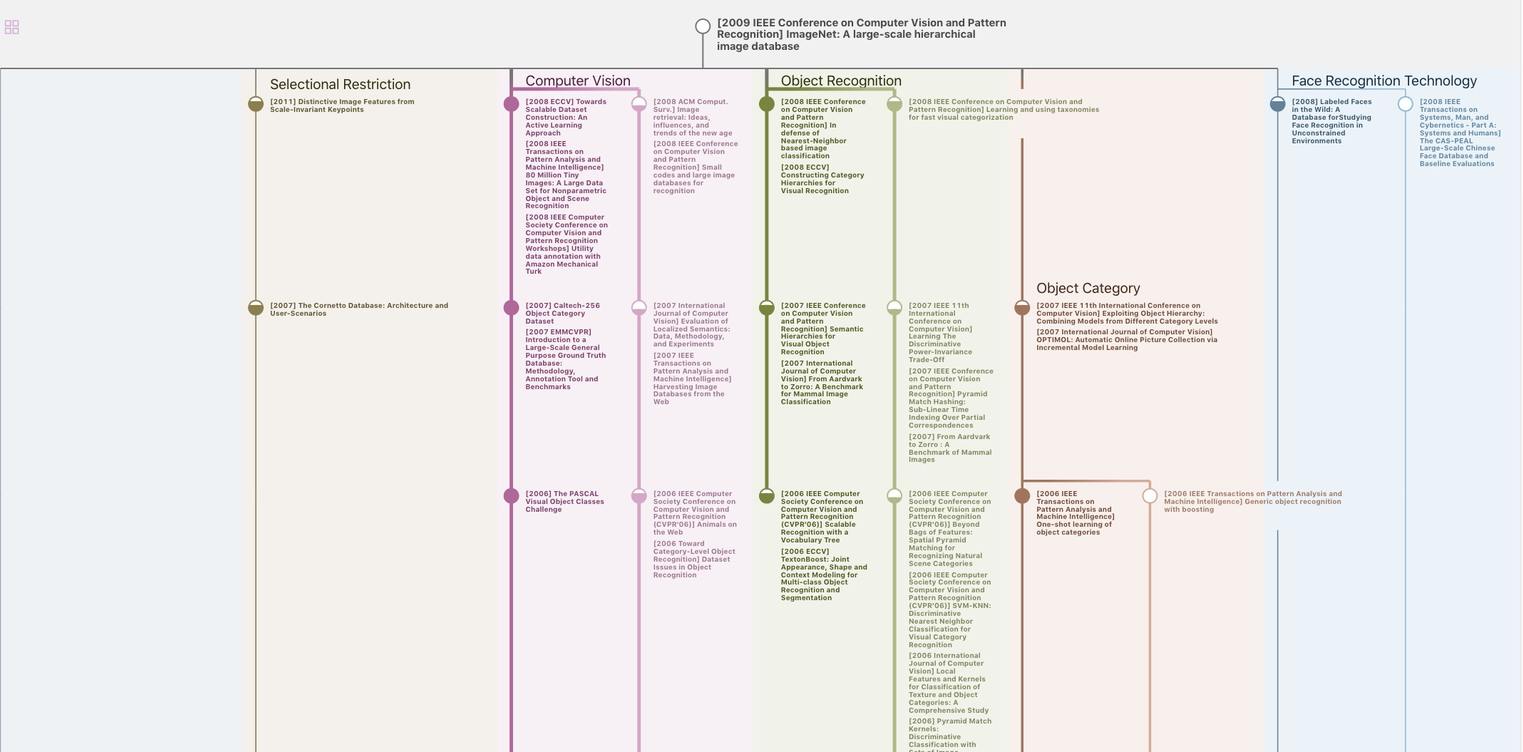
生成溯源树,研究论文发展脉络
Chat Paper
正在生成论文摘要