Memory-Enhanced Transformer for Representation Learning on Temporal Heterogeneous Graphs
Data Science and Engineering(2023)
摘要
Temporal heterogeneous graphs can model lots of complex systems in the real world, such as social networks and e-commerce applications, which are naturally time-varying and heterogeneous. As most existing graph representation learning methods cannot efficiently handle both of these characteristics, we propose a Transformer-like representation learning model, named THAN, to learn low-dimensional node embeddings preserving the topological structure features, heterogeneous semantics, and dynamic patterns of temporal heterogeneous graphs, simultaneously. Specifically, THAN first samples heterogeneous neighbors with temporal constraints and projects node features into the same vector space, then encodes time information and aggregates the neighborhood influence in different weights via type-aware self-attention. To capture long-term dependencies and evolutionary patterns, we design an optional memory module for storing and evolving dynamic node representations. Experiments on three real-world datasets demonstrate that THAN outperforms the state-of-the-arts in terms of effectiveness with respect to the temporal link prediction task.
更多查看译文
关键词
Temporal heterogeneous graphs,Graph neural networks,Graph representation learning,Transformer
AI 理解论文
溯源树
样例
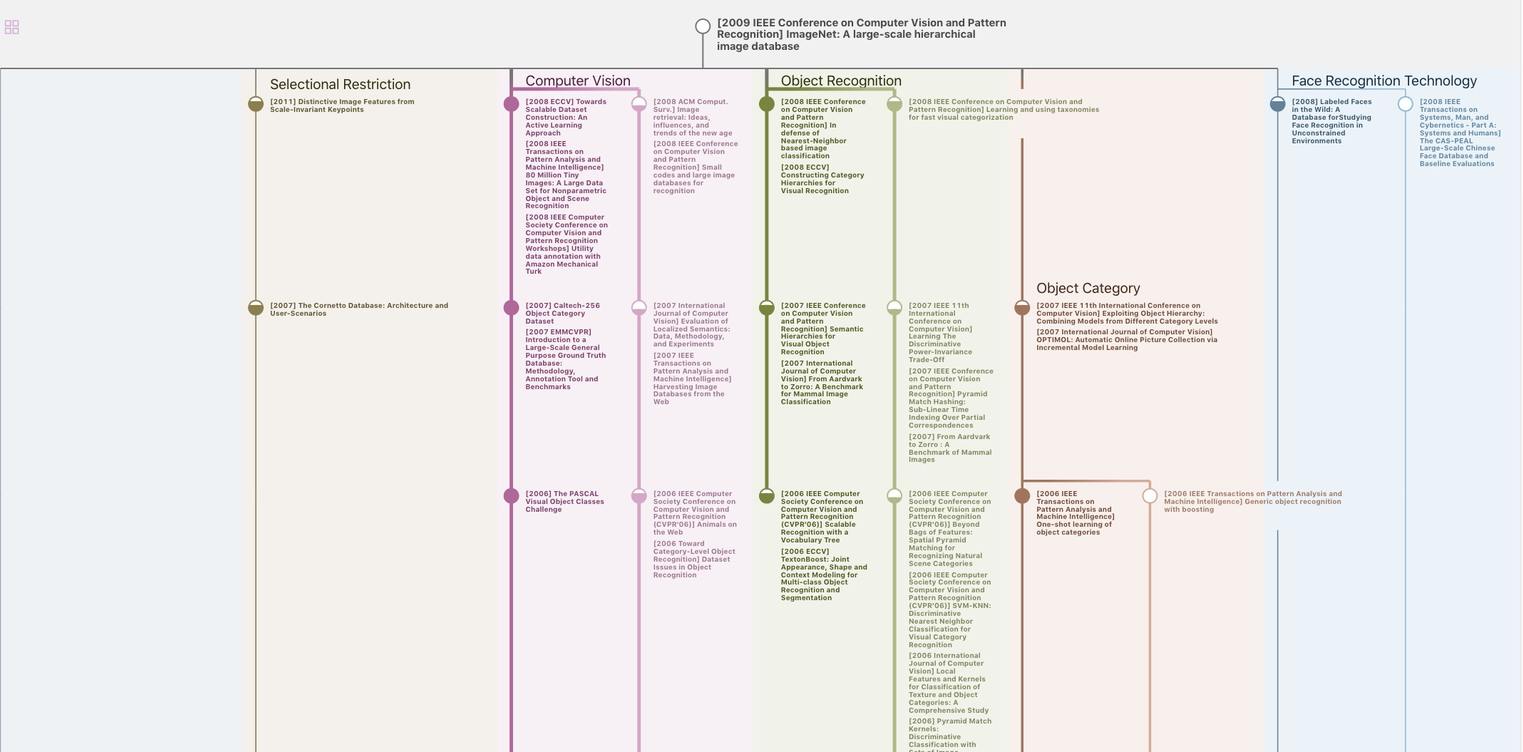
生成溯源树,研究论文发展脉络
Chat Paper
正在生成论文摘要